A tunable language model for statistical machine translation.
AMTA(2014)
摘要
A novel variation of modified KNESER-NEY model using monomial discounting is presented and integrated into the MOSES statistical machine translation toolkit. The language model is trained on a large training set as usual, but its new discount parameters are tuned to the small development set. An in-domain and cross-domain evaluation of the language model is performed based on perplexity, in which sizable improvements are obtained. Additionally, the performance of the language model is also evaluated in several major machine translation tasks including Chinese-to-English. In those tests, the test data is from a (slightly) different domain than the training data. The experimental results indicate that the new model significantly outperforms a baseline model using SRILM in those domain adaptation scenarios. The new language model is thus ideally suited for domain adaptation without sacrificing performance on in-domain experiments.
更多查看译文
关键词
statistical machine translation,tunable language model
AI 理解论文
溯源树
样例
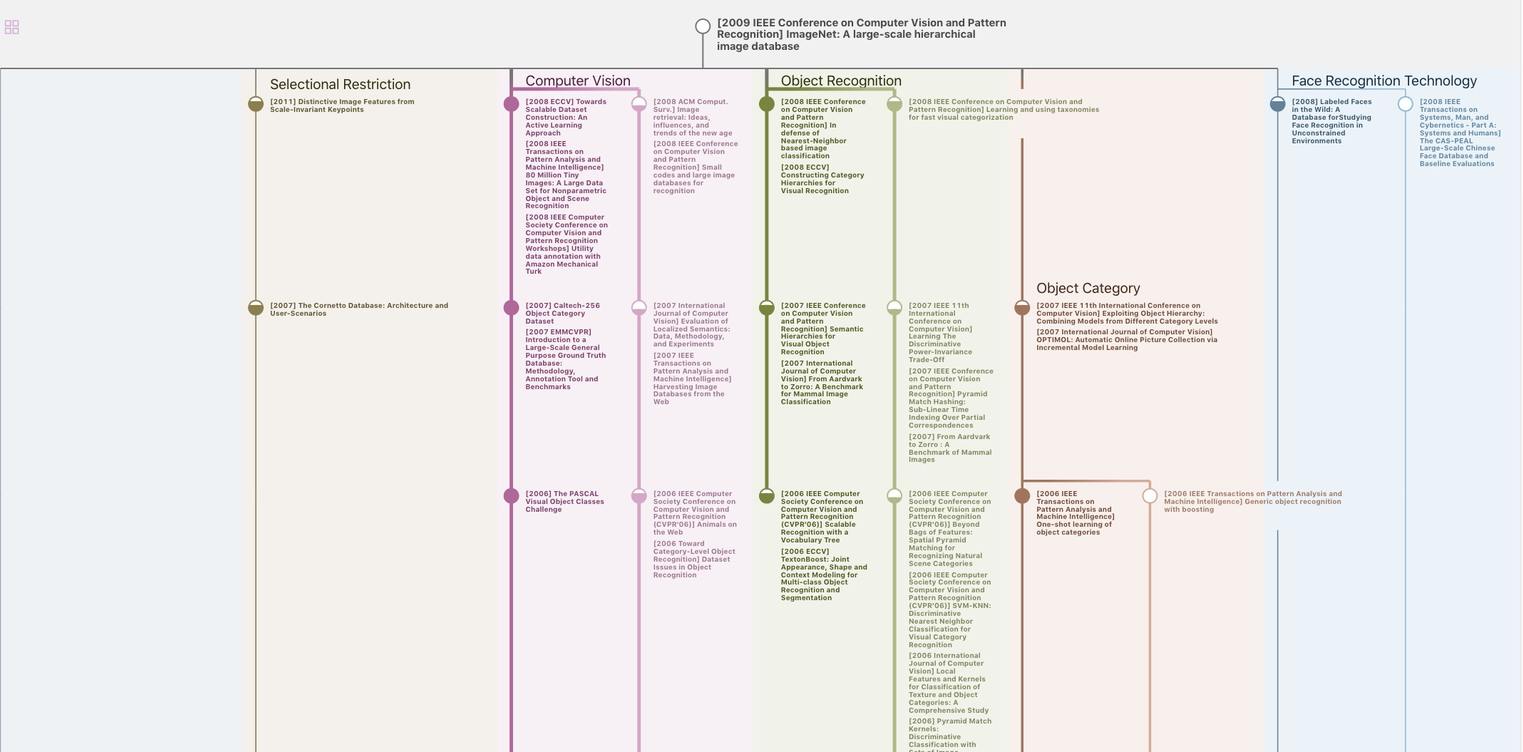
生成溯源树,研究论文发展脉络
Chat Paper
正在生成论文摘要