The Limits to Learning a Diffusion Model
Economics and Computation(2021)
摘要
ABSTRACTThis paper provides the first sample complexity lower bounds for the estimation of simple diffusion models which seek to explain the diffusion of an epidemic in a network. The Susceptible-Infected-Recovered (SIR) model is a classic example, proposed nearly a century ago [2]. The SIR model remains a cornerstone for the forecasting of epidemics. The so-called Bass model [1] remains a basic building block in forecasting consumer adoption of new products and services. The durability of these models arises from the fact that they have shown an excellent fit to data, in numerous studies spanning both the epidemiology and marketing literatures. Somewhat paradoxically, using these same models as reliable forecasting tools presents a challenge. While we are ultimately motivated by the problem of forecasting a diffusion model, this paper asks a more basic question that is surprisingly unanswered: What are the limits to learning a diffusion model? We answer this question by characterizing sample complexity lower bounds for a class of stochastic diffusion models that encompass both the Bass model and the SIR model. We show that the time to collect a number of observations that exceeds these lower bounds is too large to allow for accurate forecasts early in the process. In the context of the Bass model our results imply that when adoption is driven by imitation, one cannot hope to predict the eventual number of adopting customers until one is at least two-thirds of the way to the time at which the rate of new adopters is at its peak. In a similar vein, our results imply that in the case of an SIR model, one cannot hope to predict the eventual number of infections until one is approximately two-thirds of the way to the time at which the infection rate has peaked. Our analysis is conceptually simple and relies on the Cramer-Rao bound. The core technical difficulty in our analysis rests in characterizing the Fisher information in the observations available due to the fact that they have a non-trivial correlation structure. Maximum likelihood estimation of diffusion models on product adoption datasets (for products on Amazon.com), and epidemic data (from the ongoing COVID-19 epidemic) illustrate precisely the behavior predicted by our theory. As a byproduct of our analysis, we see that the difficulty in learning a diffusion model stems solely from uncertainty in a single unknown 'effective population size' parameter. In particular, other parameters, including those related to the 'rate of imitation' (in the Bass model) or the 'reproduction number' (in the SIR model) are easy to learn. This suggests that estimators that rely on an (informative) bias in this population size parameter can in fact overcome the limitations presented by our analysis. Although not a primary contribution of the present work, we describe a heuristic procedure used to construct such a biased estimator that yielded one of the first US county-level forecasters available for COVID-19. The full paper is available at https://arxiv.org/abs/2006.06373.
更多查看译文
AI 理解论文
溯源树
样例
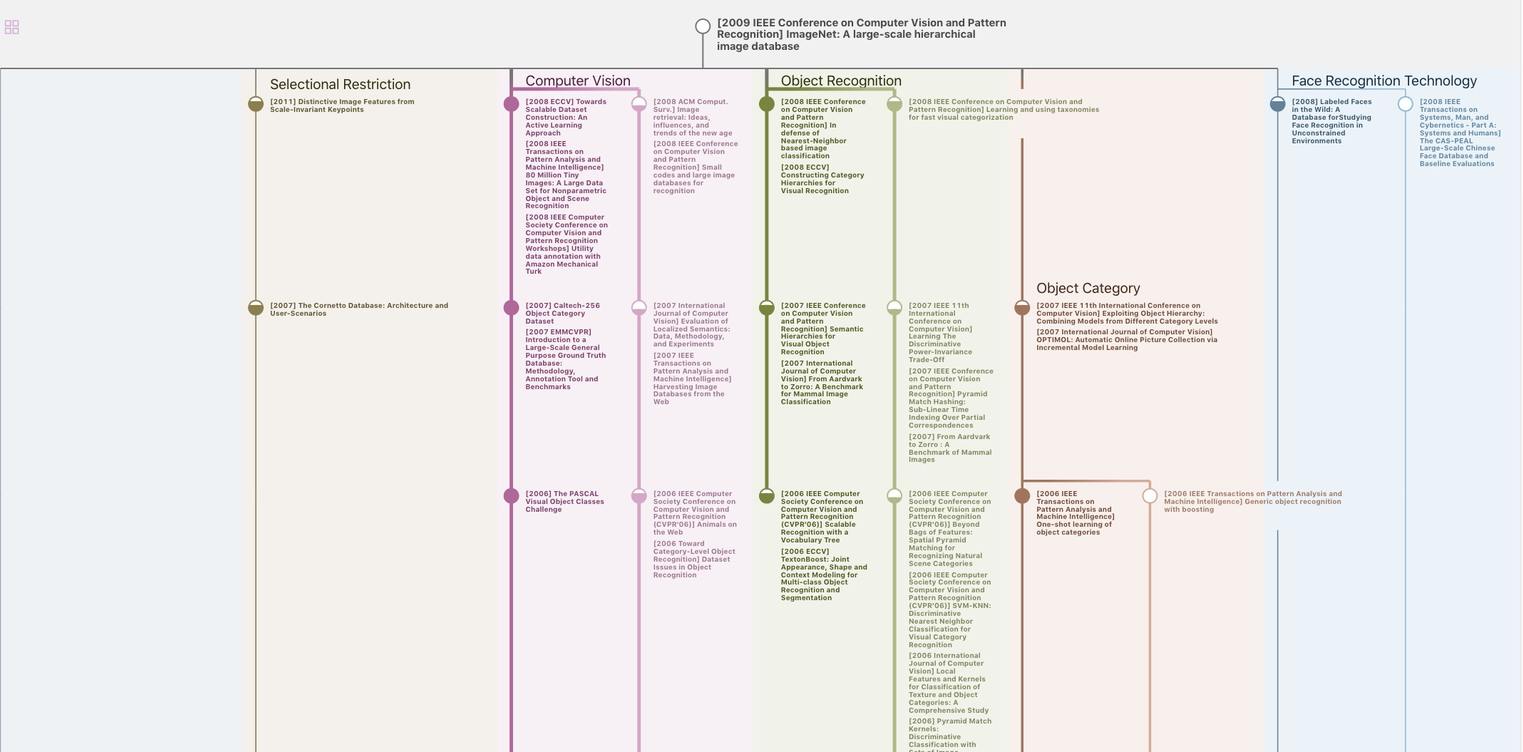
生成溯源树,研究论文发展脉络
Chat Paper
正在生成论文摘要