Magic: Learning Macro-Actions For Online Pomdp Planning
ROBOTICS: SCIENCE AND SYSTEM XVII(2021)
摘要
The partially observable Markov decision process (POMDP) is a principled general framework for robot decision making under uncertainty, but POMDP planning suffers from high computational complexity, when long-term planning is required. While temporally-extended macro-actions help to cut down the effective planning horizon and significantly improve computational efficiency, how do we acquire good macroactions? This paper proposes Macro-Action Generator-Critic (MAGIC), which performs offline learning of macro-actions optimized for online POMDP planning. Specifically, MAGIC learns a macro-action generator end-to-end, using an online planner's performance as the feedback. During online planning, the generator generates on the fly situation-aware macro-actions conditioned on the robot's belief and the environment context. We evaluated MAGIC on several long-horizon planning tasks both in simulation and on a real robot. The experimental results show that the learned macro-actions offer significant benefits in online planning performance, compared with primitive actions and handcrafted macro-actions.
更多查看译文
关键词
planning,learning,macro-actions
AI 理解论文
溯源树
样例
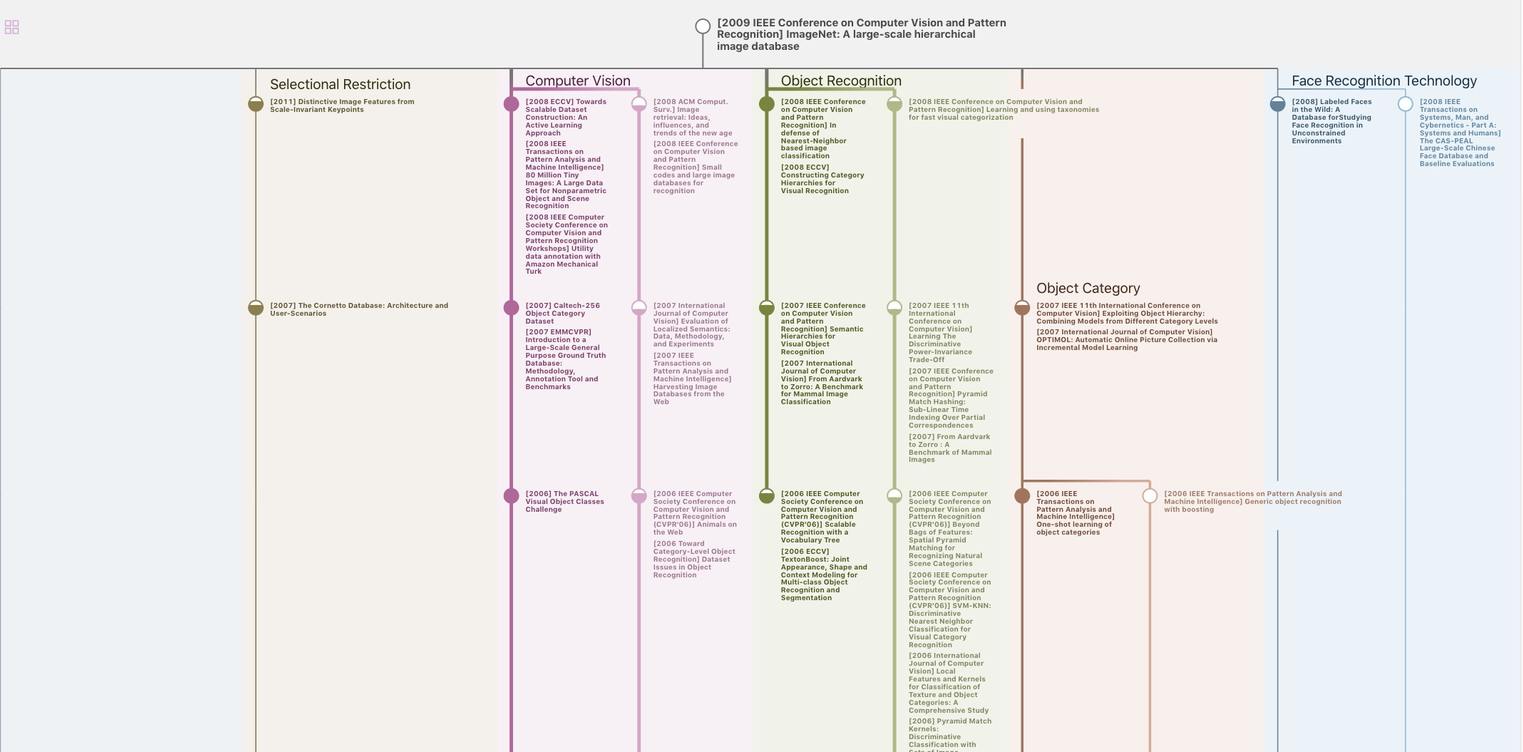
生成溯源树,研究论文发展脉络
Chat Paper
正在生成论文摘要