Compute-in-Memory Upside Down - A Learning Operator Co-Design Perspective for Scalability.
DATE(2021)
摘要
This paper discusses the potential of model-hardware co-design to simplify the implementation complexity of compute-in-SRAM deep learning considerably. Although compute-in-SRAM has emerged as a promising approach to improve the energy efficiency of DNN processing, current implementations suffer due to complex and excessive mixed-signal peripherals, such as the need for parallel digital-to-analog converters (DACs) at each input port. Comparatively, our approach inherently obviates complex peripherals by co-designing learning operators to SRAM's operational constraints. For example, our co-designed implementation is DAC-free even for multibit precision DNN processing. Additionally, we also discuss the interaction of our compute-in-SRAM operator with Bayesian inference of DNNs. We show a synergistic interaction of Bayesian inference with our framework, where Bayesian methods allow achieving similar accuracy with much smaller network size. Although each iteration of sample-based Bayesian inference is computationally expensive, the cost is minimized by our compute-in-SRAM approach. Meanwhile, by reducing the network size, Bayesian methods reduce the footprint cost of compute-in-SRAM implementation, which is a crucial concern for the method. We characterize this interaction for deep learning-based pose (position and orientation) estimation for a drone.
更多查看译文
关键词
Deep neural networks,Compute-in-memory,Pose-estimation,Nanodrone
AI 理解论文
溯源树
样例
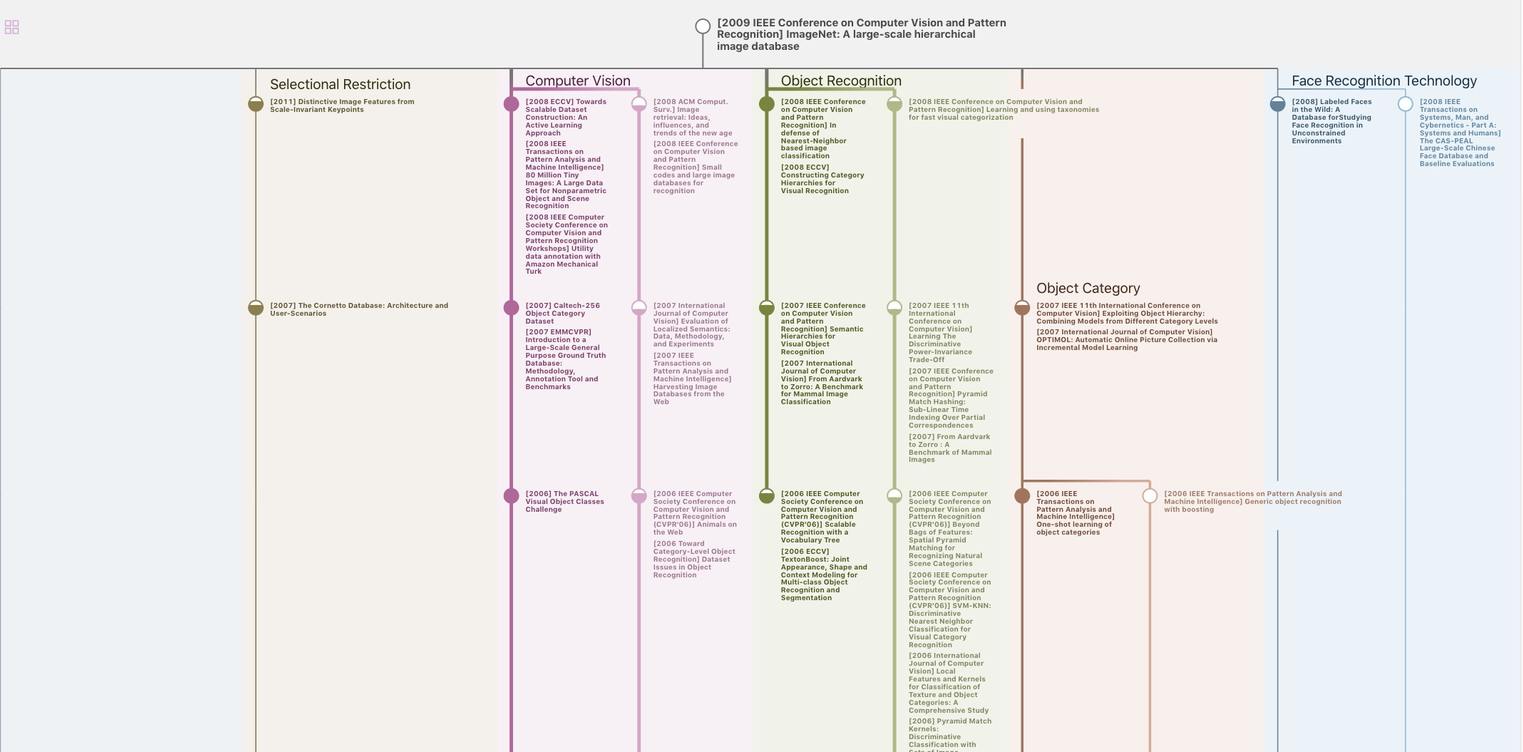
生成溯源树,研究论文发展脉络
Chat Paper
正在生成论文摘要