State, global, and local parameter estimation using local ensemble Kalman filters: Applications to online machine learning of chaotic dynamics
QUARTERLY JOURNAL OF THE ROYAL METEOROLOGICAL SOCIETY(2022)
摘要
In a recent methodological article, we showed how to learn chaotic dynamics along with the state trajectory from sequentially acquired observations, using local ensemble Kalman filters. Here, we investigate more systematically the possibility of using a local ensemble Kalman filter with either covariance localisation or local domains, in order to retrieve the state and a mix of key global and local parameters. Global parameters are meant to represent the surrogate dynamical core, for instance through a neural network, which is reminiscent of data-driven machine learning of dynamics, while the local parameters typically stand for the forcings of the model. Aiming at joint state and parameter estimation, a family of algorithms for covariance and local domain localisation is proposed. In particular, we show how to update global parameters rigorously using a local-domain ensemble Kalman filter (EnKF) such as the local ensemble transform Kalman filter (LETKF), an inherently local method. The approach is tested with success on the 40-variable Lorenz model using several of the local EnKF flavors. A two-dimensional illustration based on a multilayer Lorenz model is finally provided. It uses radiance-like nonlocal observations. It features both local domains and covariance localisation, in order to learn the chaotic dynamics and the local forcings. This article addresses more generally the key question of online estimation of both global and local model parameters.
更多查看译文
关键词
chaotic dynamics, data-driven dynamics, LEnSRF, LETKF, local ensemble Kalman filters, machine learning, parameter estimation
AI 理解论文
溯源树
样例
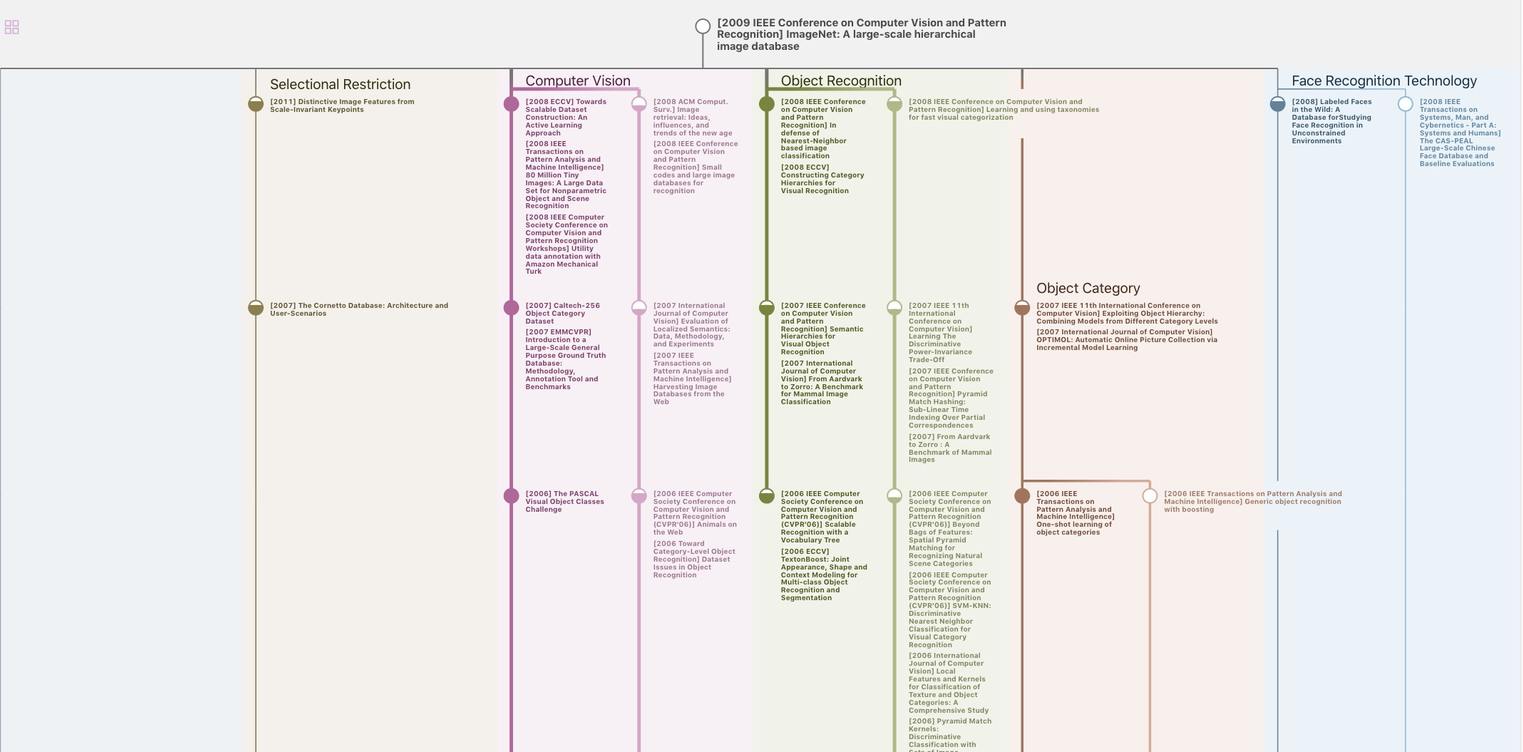
生成溯源树,研究论文发展脉络
Chat Paper
正在生成论文摘要