A Decentralized Primal-Dual Framework for Non-Convex Smooth Consensus Optimization
IEEE Transactions on Signal Processing(2023)
摘要
In this work, we introduce ADAPD,
A
D
ecentr
A
lized
P
rimal-
D
ual algorithmic framework for solving non-convex and smooth consensus optimization problems over a network of distributed agents. The proposed framework relies on a novel problem formulation that elicits ADMM-type updates, where each agent first inexactly solves a local strongly convex subproblem with any method of its choice and then performs a neighbor communication to update a set of dual variables. We present two variants that allow for a single gradient step for the primal updates or multiple communications for the dual updates, to exploit the tradeoff between the per-iteration cost and the number of iterations. When multiple communications are performed, ADAPD can achieve theoretically optimal communication complexity results for non-convex and smooth consensus problems. Numerical experiments on several applications, including a deep-learning one, demonstrate the superiority of ADAPD over several popularly used decentralized methods.
更多查看译文
关键词
Non-convex consensus optimization,decentralized optimization,primal-dual method,decentralized learning
AI 理解论文
溯源树
样例
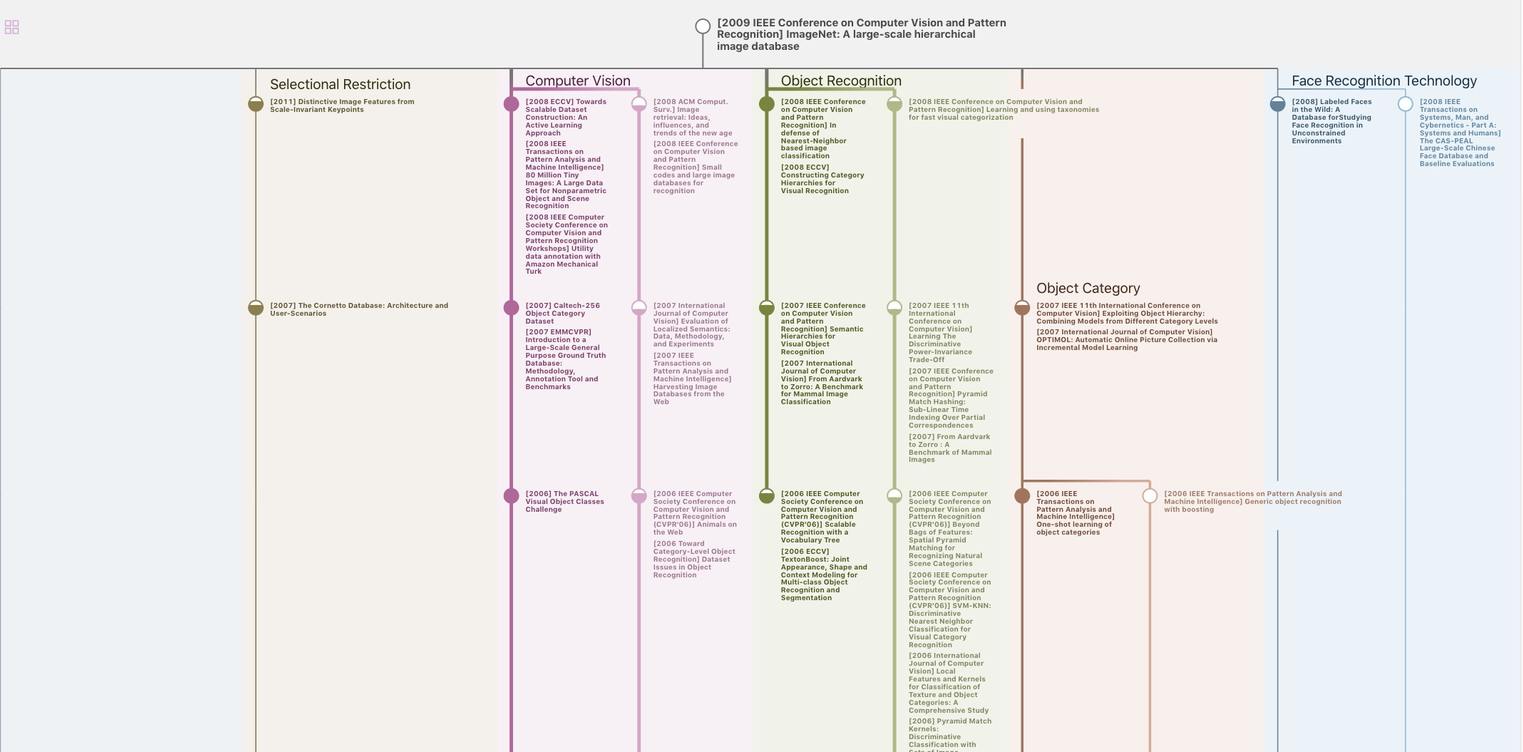
生成溯源树,研究论文发展脉络
Chat Paper
正在生成论文摘要