Deep Learning-Based Cattle Behaviour Classification Using Joint Time-Frequency Data Representation
COMPUTERS AND ELECTRONICS IN AGRICULTURE(2021)
摘要
In this paper, a sequential deep neural network in conjunction with a joint time-frequency domain data representation is explored for the problem of cattle behaviour classification. The experimental evaluation is based on a real-world dataset with over 3 million samples, collected from sensors with a tri-axial accelerometer, magnetometer and gyroscope, attached to the collar tags of 10 beef steers. The experimental result demonstrate that the time-frequency domain data representation allows to efficiently trade-off a large reduction of model size and computational complexity for a very minor reduction in classification accuracy. This shows the potential of this classification approach to run on resource-constrained embedded and IoT devices. Most importantly, the proposed behaviour classification method achieves a high classification performance with an F-1 Score of 94.9% for 3 behaviour classes, and 89.3% for 9 behaviour classes. This is in comparison to the current state-of-the-art with an F-1 Score of 94.3% (for two classes) and 88.7% (for 8 classes).
更多查看译文
关键词
Inertial Measurement Unit, Sensors, DNN, TFD, Livestock
AI 理解论文
溯源树
样例
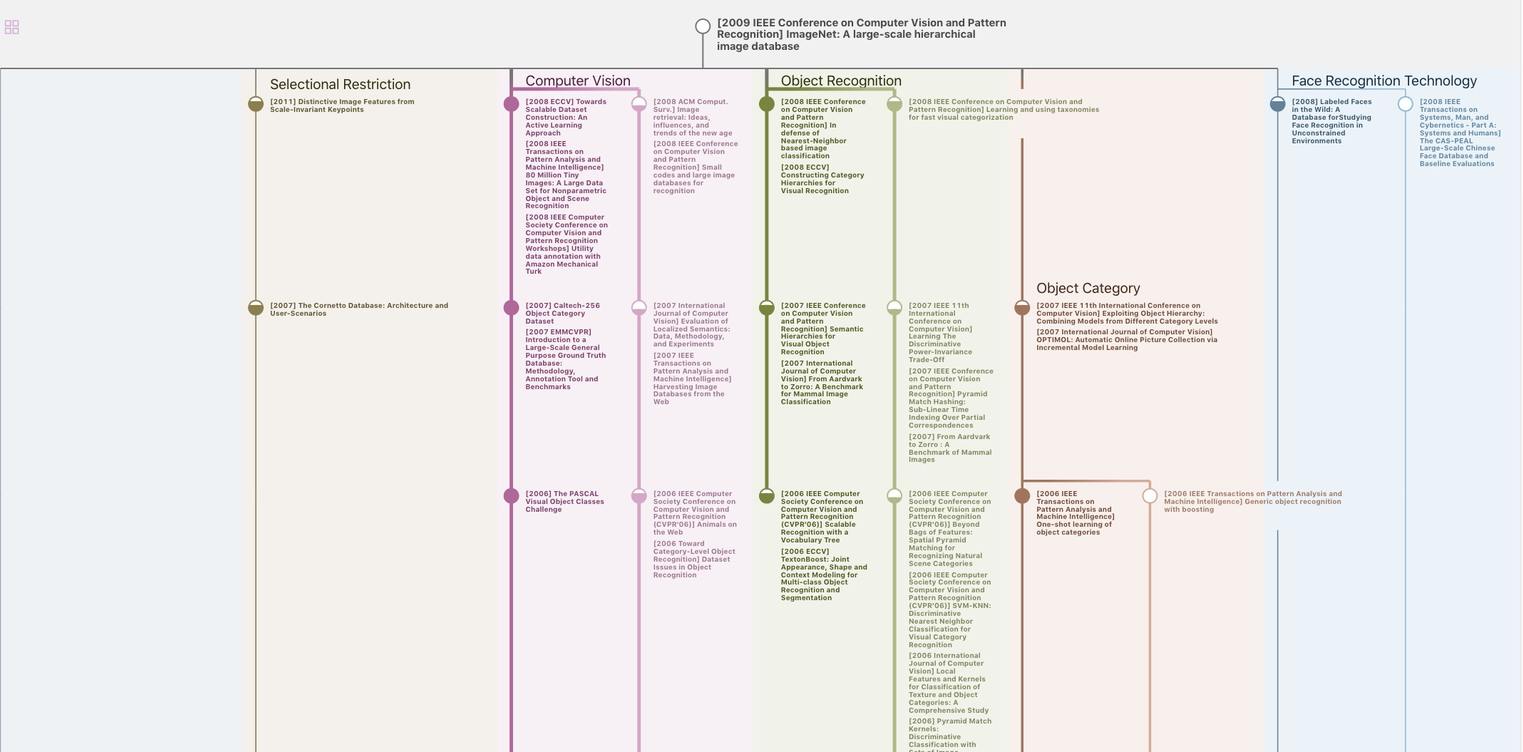
生成溯源树,研究论文发展脉络
Chat Paper
正在生成论文摘要