Direct And Accurate Feature Extraction From 3d Point Clouds Of Plants Using Ransac
COMPUTERS AND ELECTRONICS IN AGRICULTURE(2021)
摘要
While point clouds hold promise for measuring the geometrical features of 3D objects, their application to plants remains problematic. Plants are three dimensional (3D) organisms whose morphology is complex, varies from one individual to another and changes over time. Objective measurement of attributes in 3D point cloud domain is increasingly attractive as techniques improve the accuracy and reduce computational time. Analysis of point cloud data, however, is not straightforward, due to its discrete nature, imaging noise and cluttered background. In this paper, we introduce a robust method for the direct analysis of plants of point cloud data. To this end, we generalise the random sample consensus (RANSAC) algorithm for the analysis of 3D point cloud data and then use it to model different plant organs. Since 3D point clouds are obtained from multi-view stereo images, they are often contaminated with a considerable level of noise, distortions and out-of-distribution points. Key to our approach is the use of the RANSAC algorithm on 3D point cloud, making our technique more robust to undesirable outliers. We tested our proposed method on Brassica and grapevine by comparing the estimated measurements extracted from the models with manual ones taken from the actual plants. Our proposed method achieved R-2 > 0.90 for measured diameters of branches and stems in Brassica while it yielded R-2 > 0.91 for the measured leaf angles of grapevine and branch angles of Brassica. In all cases, the approach produced stable performance under imaging noise and cluttered background while the conventional methods often failed to work.
更多查看译文
关键词
Point cloud, 3D analysis, Random sample consensus (RANSAC), Brassica, Grapevine
AI 理解论文
溯源树
样例
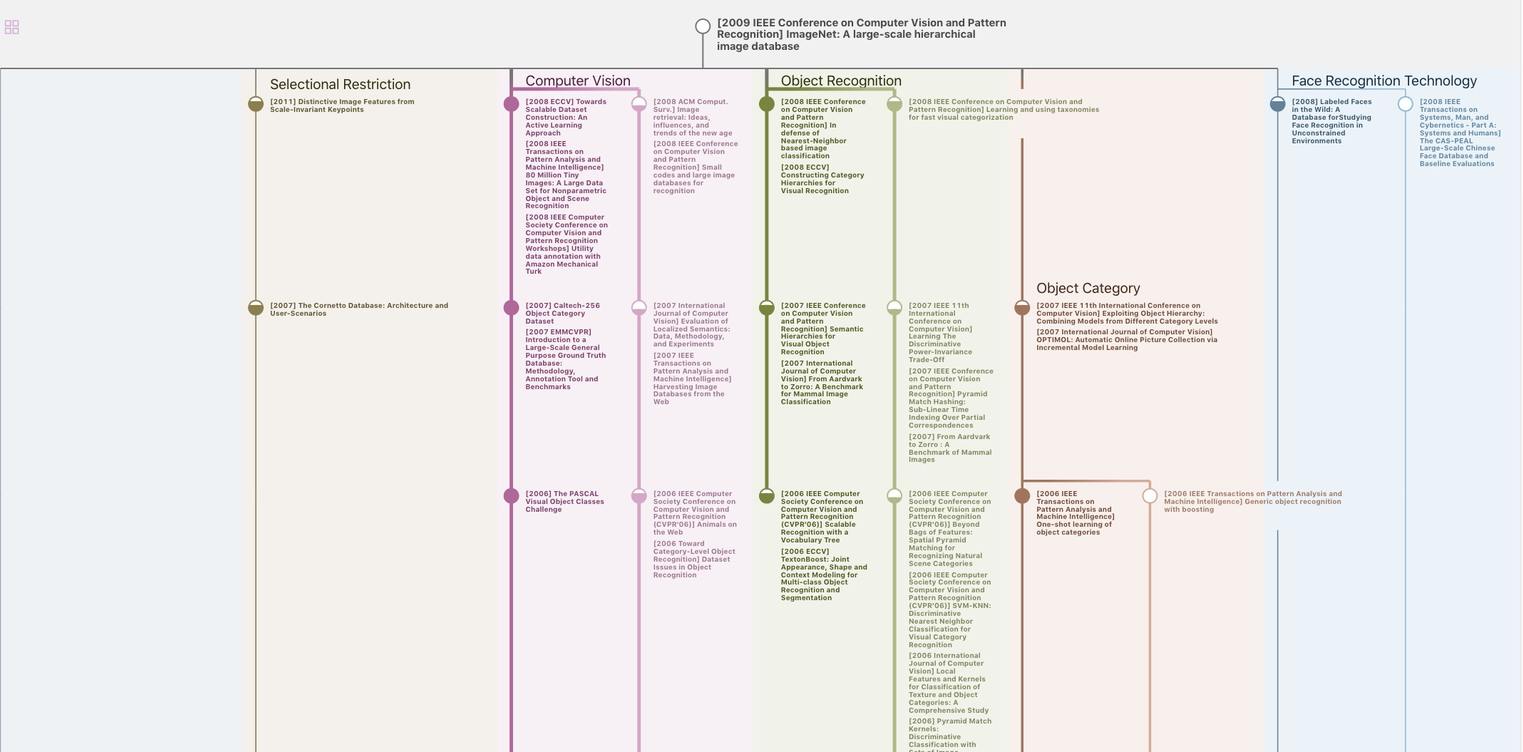
生成溯源树,研究论文发展脉络
Chat Paper
正在生成论文摘要