FACTORIZED VARIATIONAL AUTOENCODERS
user-5da93e5d530c70bec9508e2b(2019)
摘要
The disclosure provides an approach for learning latent representations of data using factorized variational autoencoders (FVAEs). The FVAE framework builds a hierarchical Bayesian matrix factorization model on top of a variational autoencoder (VAE) by learning a VAE that has a factorized representation so as to compress the embedding space and enhance generalization and interpretability. In one embodiment, an FVAE application takes as input training data comprising observations of objects, and the FVAE application learns a latent representation of such data. In order to learn the latent representation, the FVAE application is configured to use a probabilistic VAE to jointly learn a latent representation of each of the objects and a corresponding factorization across time and identity.
更多查看译文
关键词
Autoencoder,Representation (mathematics),Matrix decomposition,Interpretability,Generalization,Probabilistic logic,Factorization,Embedding,Pattern recognition,Computer science,Artificial intelligence
AI 理解论文
溯源树
样例
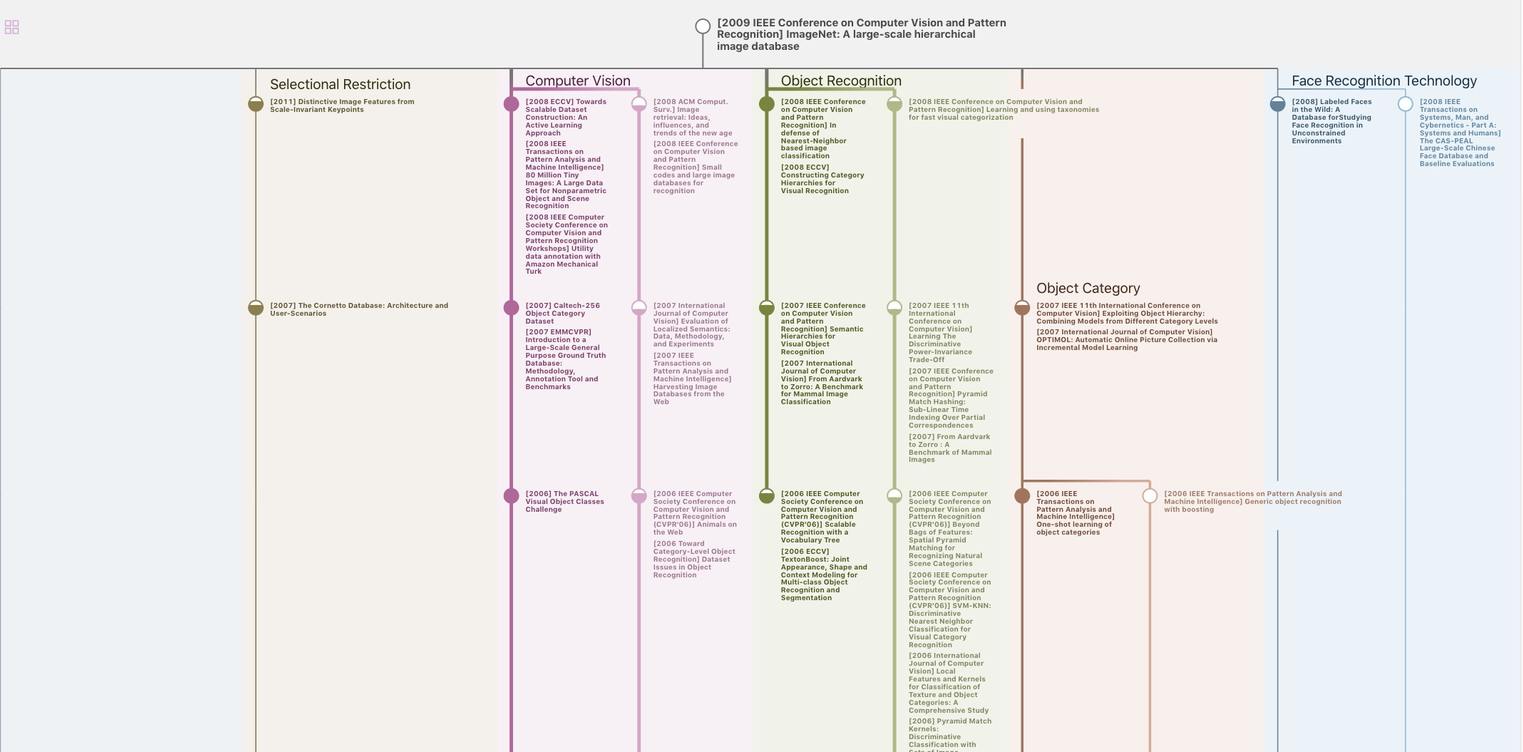
生成溯源树,研究论文发展脉络
Chat Paper
正在生成论文摘要