Multi-Perspective Content Delivery Networks Security Framework Using Optimized Unsupervised Anomaly Detection
IEEE transactions on network and service management/IEEE eTransactions on network and service management(2022)
摘要
Content delivery networks (CDNs) provide efficient content distribution over the Internet. CDNs improve the connectivity and efficiency of global communications, but their caching mechanisms may be breached by cyber-attackers. Among the security mechanisms, effective anomaly detection forms an important part of CDN security enhancement. In this work, we propose a multi-perspective unsupervised learning framework for anomaly detection in CDNs. In the proposed framework, a multi-perspective feature engineering approach, an optimized unsupervised anomaly detection model that utilizes an isolation forest and a Gaussian mixture model, and a multi-perspective validation method, are developed to detect abnormal behaviors in CDNs mainly from the client Internet Protocol (IP) and node perspectives, therefore to identify the denial of service (DoS) and cache pollution attack (CPA) patterns. Experimental results are presented based on the analytics of eight days of real-world CDN log data provided by a major CDN operator. Through experiments, the abnormal contents, compromised nodes, malicious IPs, as well as their corresponding attack types, are identified effectively by the proposed framework and validated by multiple cybersecurity experts. This shows the effectiveness of the proposed method when applied to real-world CDN data.
更多查看译文
关键词
Servers,Anomaly detection,Image edge detection,Internet,IP networks,Pollution,Protocols,Cache pollution attacks,DoS attacks,anomaly detection,content delivery networks,Gaussian mixture model,Bayesian optimization
AI 理解论文
溯源树
样例
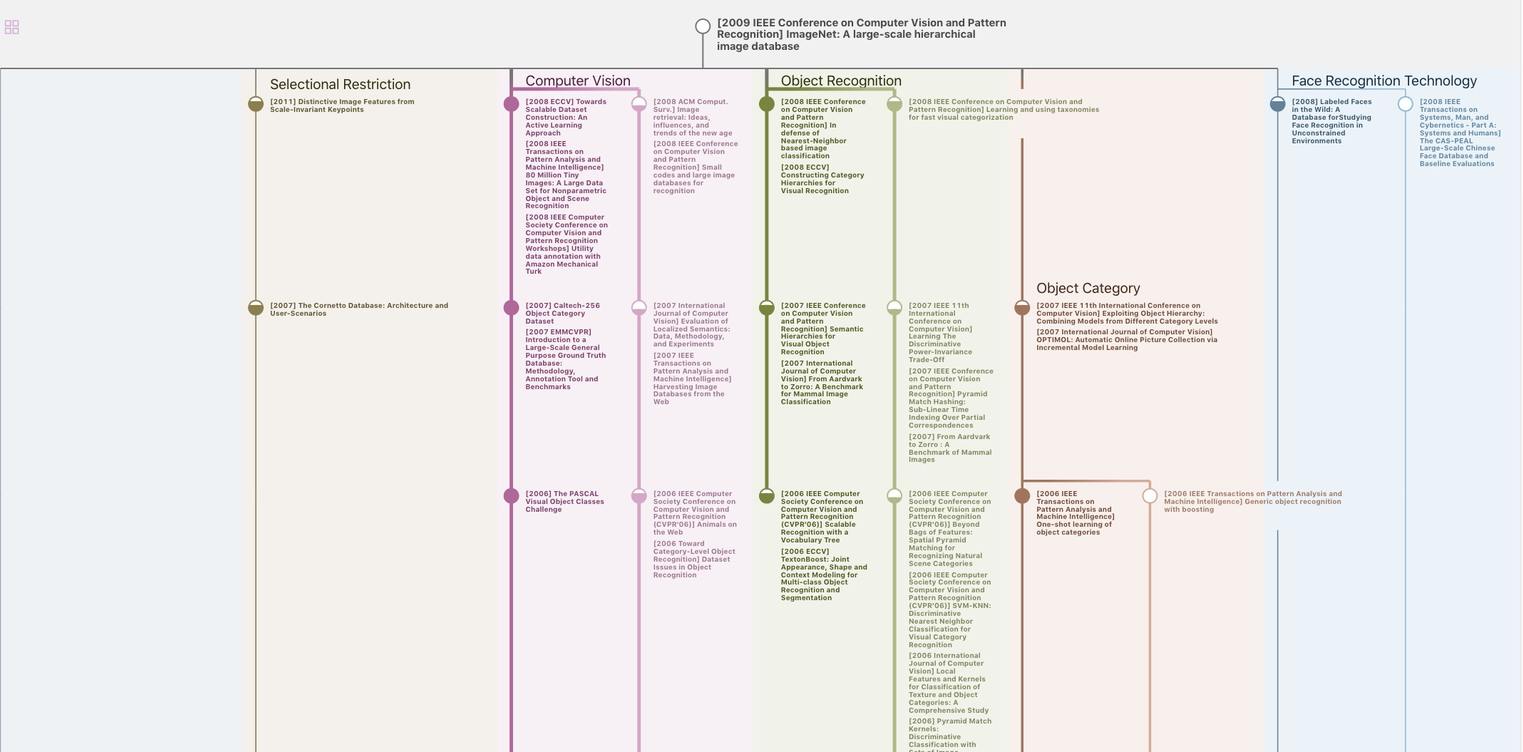
生成溯源树,研究论文发展脉络
Chat Paper
正在生成论文摘要