Rethinking and Improving Relative Position Encoding for Vision Transformer.
2021 IEEE/CVF International Conference on Computer Vision (ICCV)(2021)
摘要
Relative position encoding (RPE) is important for transformer to capture sequence ordering of input tokens. General efficacy has been proven in natural language processing. However, in computer vision, its efficacy is not well studied and even remains controversial, e.g., whether relative position encoding can work equally well as absolute position? In order to clarify this, we first review existing relative position encoding methods and analyze their pros and cons when applied in vision transformers. We then propose new relative position encoding methods dedicated to 2D images, called image RPE (iRPE). Our methods consider directional relative distance modeling as well as the interactions between queries and relative position embeddings in self-attention mechanism. The proposed iRPE methods are simple and lightweight. They can be easily plugged into transformer blocks. Experiments demonstrate that solely due to the proposed encoding methods, DeiT [21] and DETR [1] obtain up to 1.5% (top-1 Acc) and 1.3% (mAP) stable improvements over their original versions on ImageNet and COCO respectively, without tuning any extra hyperparameters such as learning rate and weight decay. Our ablation and analysis also yield interesting findings, some of which run counter to previous understanding. Code and models are open-sourced at https://github.com/microsoft/Cream/tree/main/iRPE.
更多查看译文
关键词
Datasets and evaluation,Detection and localization in 2D and 3D,Recognition and classification
AI 理解论文
溯源树
样例
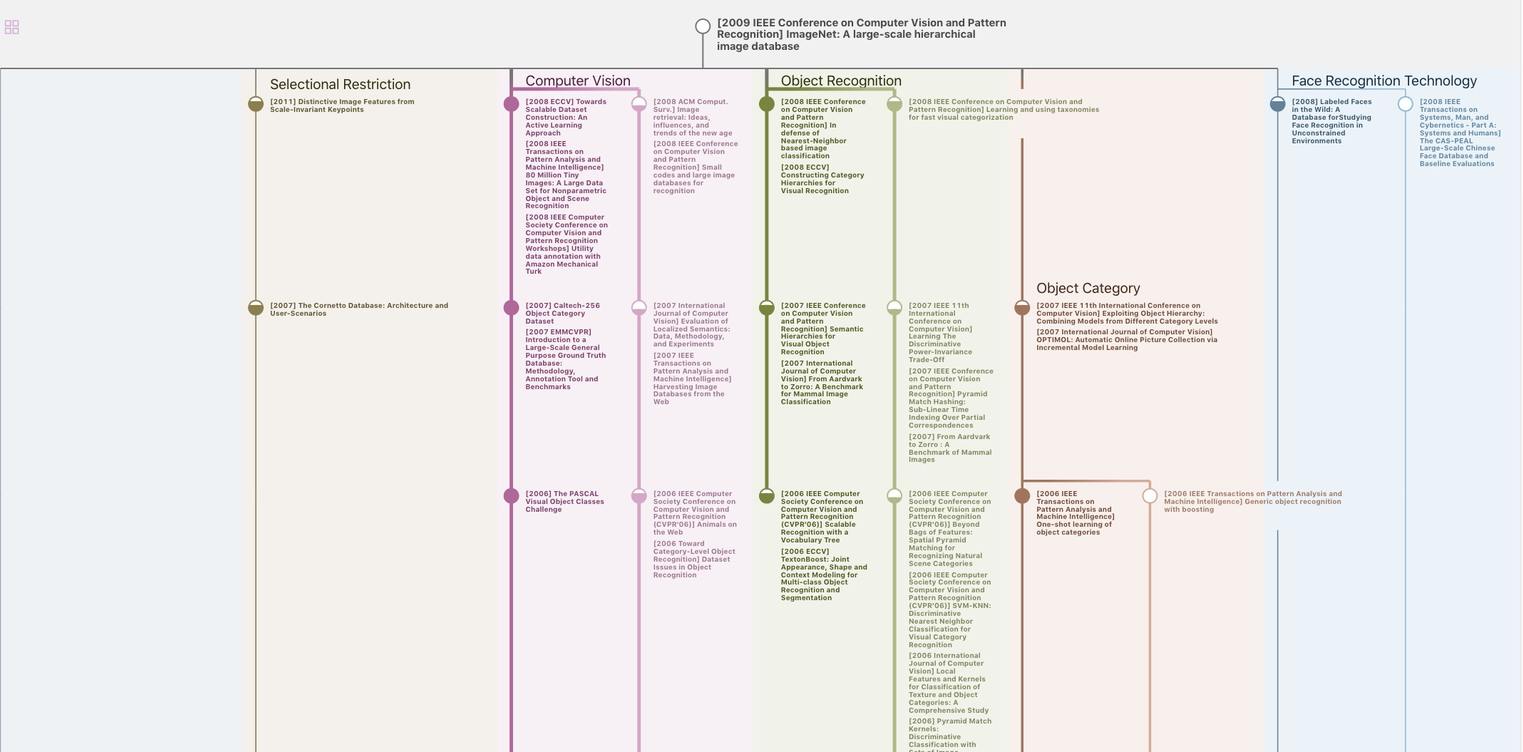
生成溯源树,研究论文发展脉络
Chat Paper
正在生成论文摘要