InSAR Deformation Time-series Reconstruction for Rainfall-induced Landslides Based on Gaussian Process Regression.
ICMLC(2021)
摘要
Multi-baseline interferometric synthetic aperture radar (InSAR) techniques have been accepted as effective remote sensing tools for detecting and monitoring landslide movements. With the use of stacked synthetic aperture radar (SAR) imageries, it is capable of generating precise ground displacement time-series. In order to further suppress noise induced by atmospheric effects, a post-process step, named as temporal filter, is required to be applied to the final displacement time-series in most applications. As displacement signals are strongly correlated in time, the traditional window-based/least squares filter is widely adopted. Since the window-based filter balances a tradeoff between noise smoothing and signal smoothing, the resulting time-series may strongly deviate from the true values when ground displacements appear high nonlinearity. In this paper, a new approach is proposed to reconstruct the InSAR deformation time-series for rainfall-induced landslides. This method establishes a nonparametric model based on the idea of Gaussian process regression (GPR) and introduces precipitation data as a priori knowledge. A strong relationship between rainfall history and ground movements is therefore constructed, which is extremely helpful in preventing the loss of high-frequency displacement signals. The proposed approach was applied to the InSAR landslide displacement time-series obtained from 108 European Space Agency (ESA) Sentinel-1A satellite SAR images. Experimental results demonstrate that it is capable of preserving the details of the temporal evolution of ground displacements effectively compared to the traditional window-based method, in particular on the surface of sliding mass.
更多查看译文
AI 理解论文
溯源树
样例
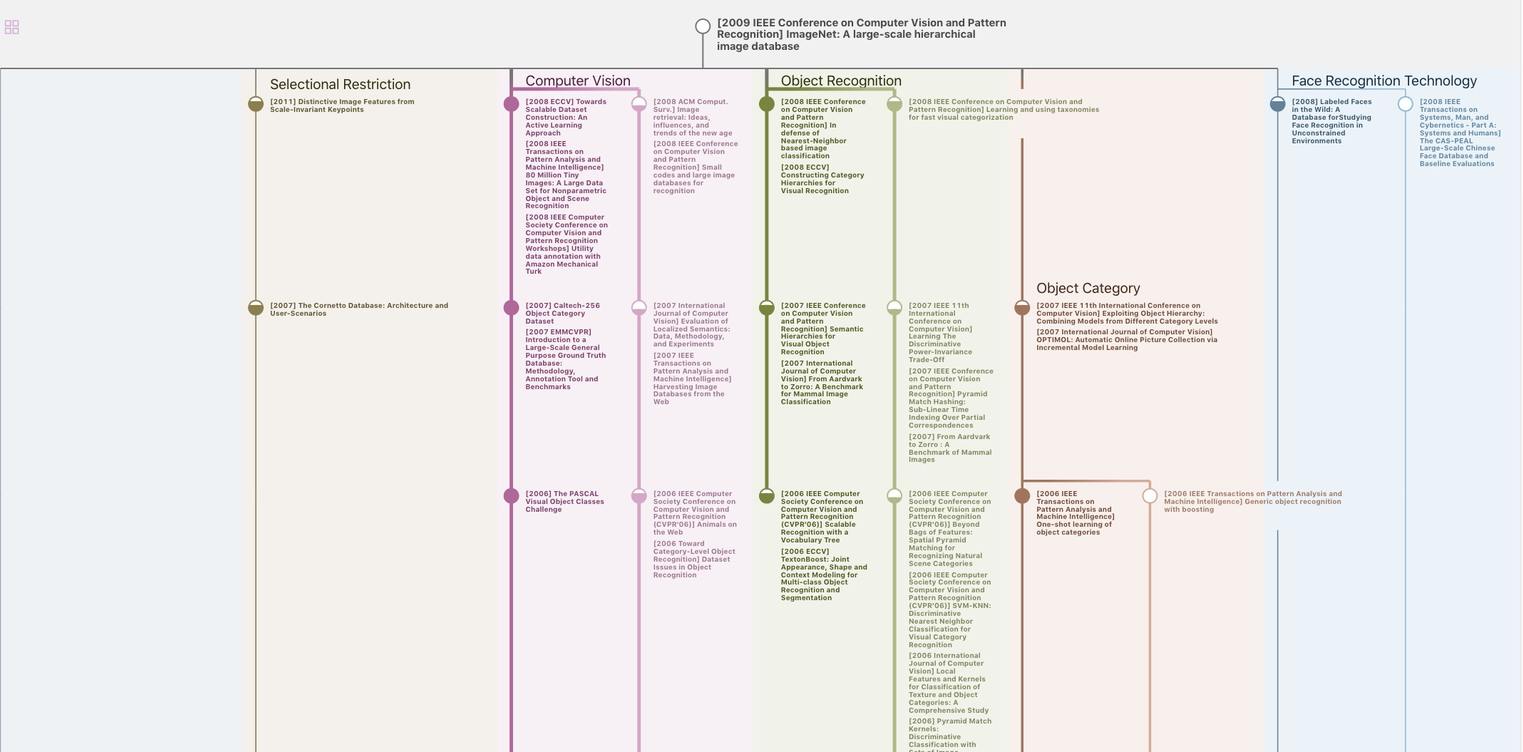
生成溯源树,研究论文发展脉络
Chat Paper
正在生成论文摘要