Multi-Perspective Reasoning Transformers.
ICMLC(2021)
摘要
Machine Reading Comprehension is defined as the ability of machines to read and understand unstructured text and answer questions about it. It is considered as a challenging task with wide range of enterprise applications. Wide range of natural language understanding and reasoning tasks are found embedded within machine reading comprehension datasets. This requires effective models with robust relational reasoning capabilities to answer complex questions. Reasoning in natural language is a long-term machine-learning goal and is critically needed for building intelligent agents. However, most papers heavily depend on underlying language modeling and thus pay little to no attention on creating effective reasoning models. This paper proposes a modified transformer architecture that effectively combines soft and hard attention to create multi-perspective reasoning model capable of tackling wide range of reasoning tasks. An attention mechanism that highlights the relational significance of input signals is considered as well. The result from this study shows performance gain as compared to its counterpart the transformer network on bAbI dataset, a natural language reasoning tasks.
更多查看译文
AI 理解论文
溯源树
样例
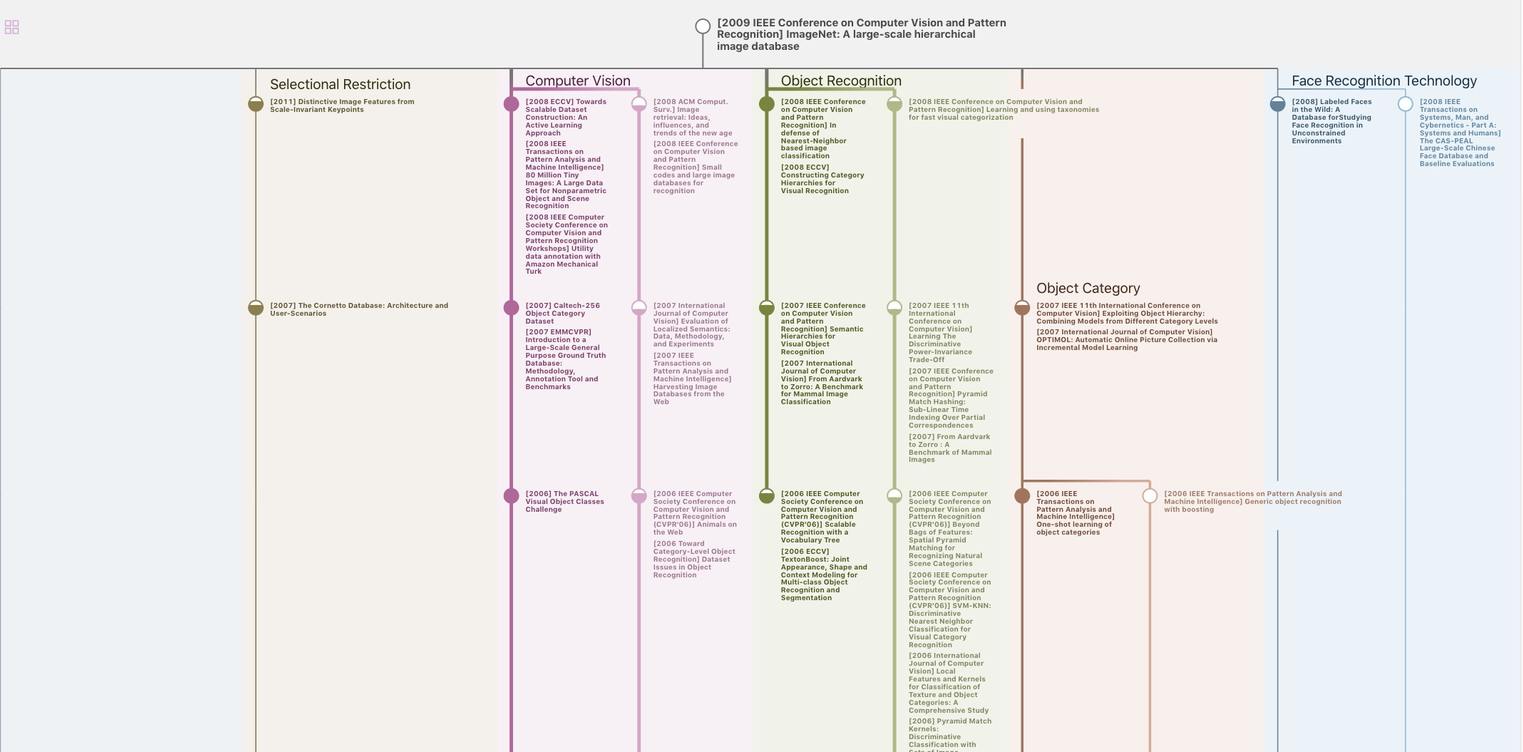
生成溯源树,研究论文发展脉络
Chat Paper
正在生成论文摘要