Comparison of RNN and Embeddings Methods for Next-item and Last-basket Session-based Recommendations.
ICMLC(2021)
摘要
Recurrent Neural Networks (RNNs) have been shown to perform very effectively in session-based recommendation settings, when compared to other commonly used methods that consider the last viewed item of the user and precomputed item-to-item similarities. However, there is little systematic study on how RNNs perform in comparison to methods that use embeddings for item representation for Collaborative Filtering. In this paper we use two industry datasets to compare RNNs with other sequential recommenders that use various embedding methods to represent items. The first dataset corresponds to a typical e-commerce session-based scenario demanding effective next-item recommendation. The second dataset represents a last-basket prediction setting. Results show that although the RNN greatly outperforms embedding methods in the next-item scenario, the dynamic nature of the RNNs has not the same positive impact in the last-basket prediction task. We also present and test a framework that enables the hybrid utilization of text content and item sequences using embeddings. Finally, we report on experiments with reranking methods that demonstrate the effectiveness of simple and practical methods, using item categories, to improve the results.
更多查看译文
AI 理解论文
溯源树
样例
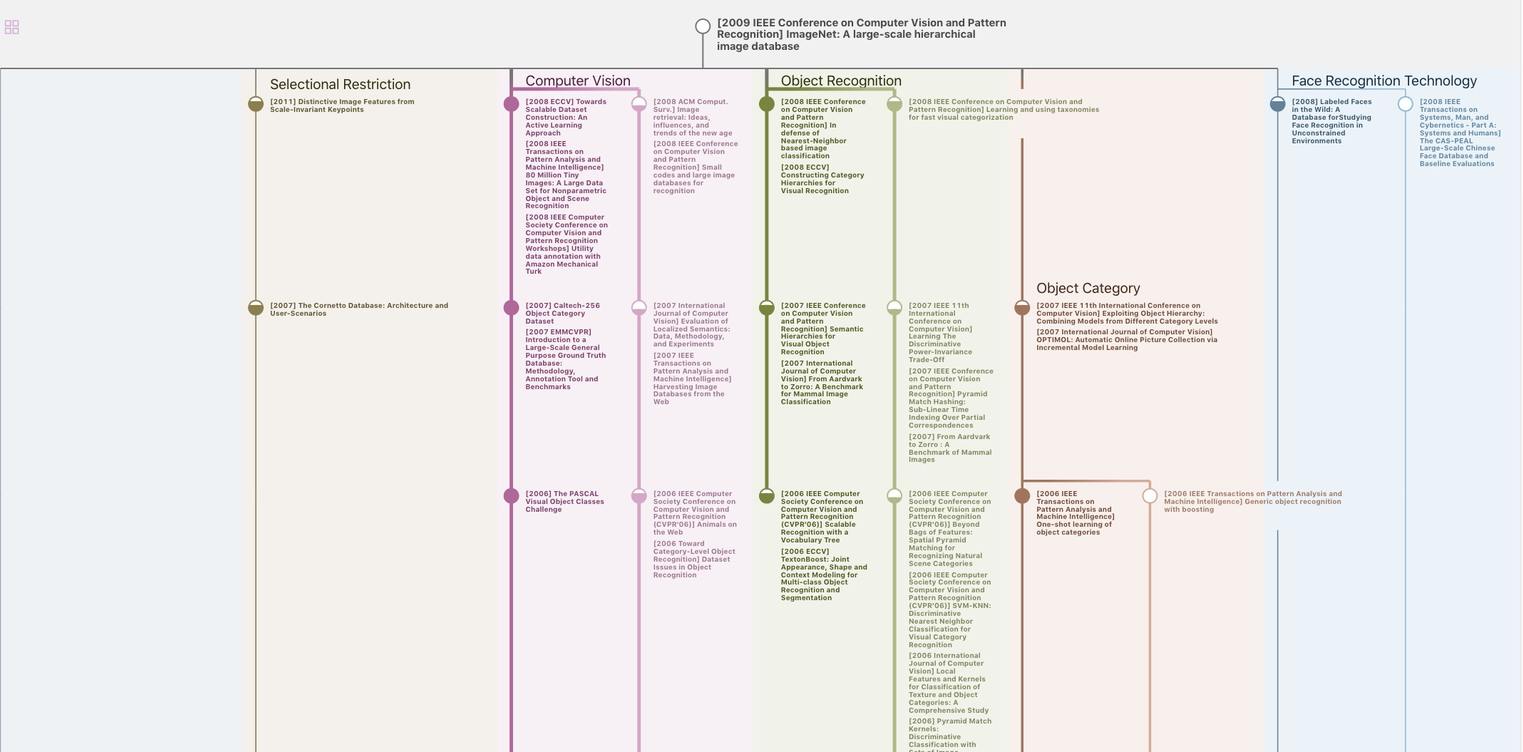
生成溯源树,研究论文发展脉络
Chat Paper
正在生成论文摘要