Geometric Deep Learning for Subject Independent Epileptic Seizure Prediction Using Scalp EEG Signals
IEEE Journal of Biomedical and Health Informatics(2022)
摘要
Recently, researchers in the biomedical community have introduced deep learning-based epileptic seizure prediction models using electroencephalograms (EEGs) that can anticipate an epileptic seizure by differentiating between the pre-ictal and interictal stages of the subject’s brain. Despite having the appearance of a typical anomaly detection task, this problem is complicated by subject-specific characteristics in EEG data. Therefore, studies that investigate seizure prediction widely employ subject-specific models. However, this approach is not suitable in situations where a target subject has limited (or no) data for training. Subject-independent models can address this issue by learning to predict seizures from multiple subjects, and therefore are of greater value in practice. In this study, we propose a subject-independent seizure predictor using Geometric Deep Learning (GDL). In the first stage of our GDL-based method we use graphs derived from physical connections in the EEG grid. We subsequently seek to synthesize subject-specific graphs using deep learning. The models proposed in both stages achieve state-of-the-art performance using a one-hour early seizure prediction window on two benchmark datasets (CHB-MIT-EEG: 95.38% with 23 subjects and Siena-EEG: 96.05% with 15 subjects). To the best of our knowledge, this is the first study that proposes synthesizing subject-specific graphs for seizure prediction. Furthermore, through model interpretation we outline how this method can potentially contribute towards Scalp EEG-based seizure localization.
更多查看译文
关键词
Machine learning,neural networks,signal processing,seizure prediction,electroencephalography
AI 理解论文
溯源树
样例
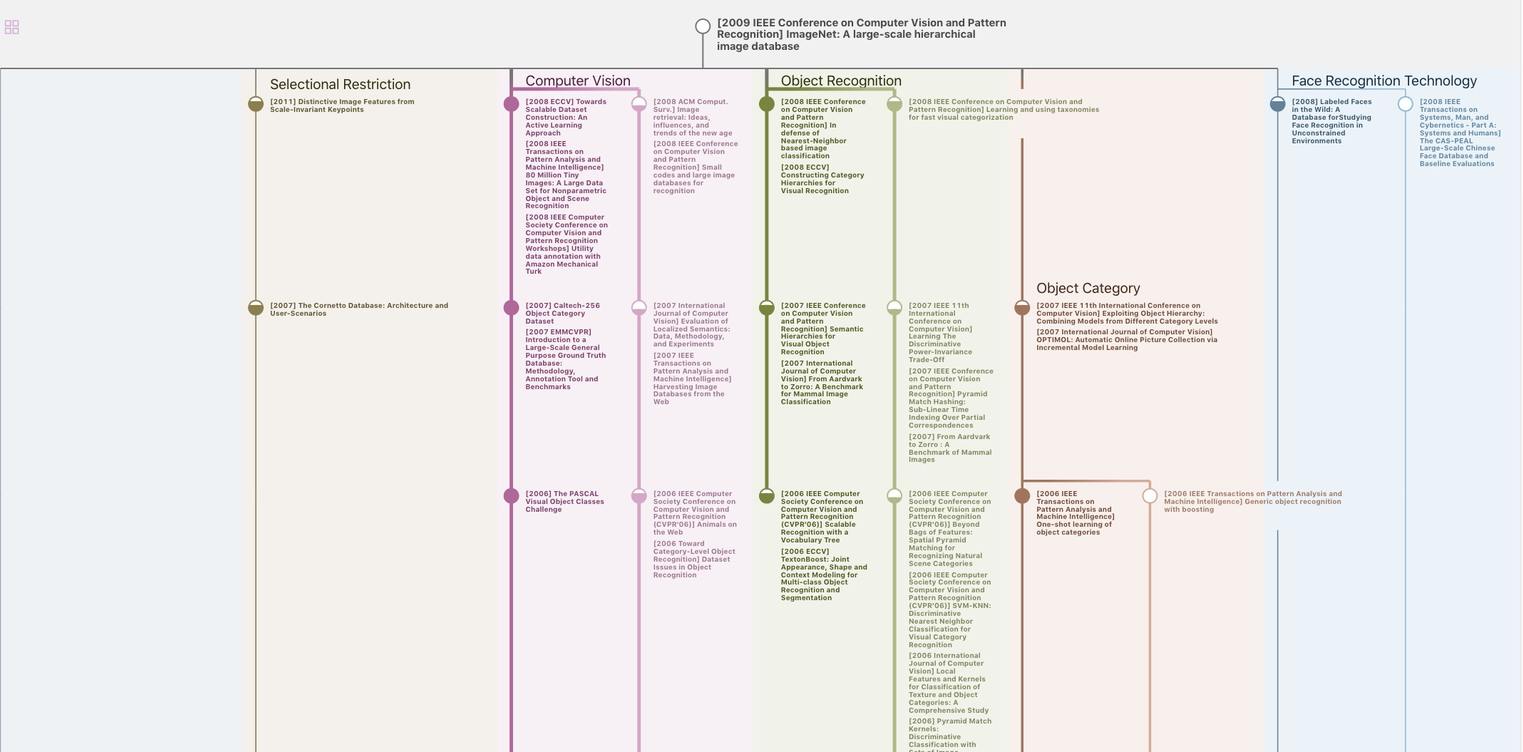
生成溯源树,研究论文发展脉络
Chat Paper
正在生成论文摘要