Don't Miss the Labels: Label-semantic Augmented Meta-Learner for Few-Shot Text Classification.
FINDINGS OF THE ASSOCIATION FOR COMPUTATIONAL LINGUISTICS, ACL-IJCNLP 2021(2021)
摘要
Increasing studies leverage pre-trained language models and meta-learning frameworks to solve few-shot text classification problems. Most of the current studies focus on building a meta-learner from the information of input texts but ignore abundant semantic information beneath class labels. In this work, we show that class-label information can be utilized for extracting more discriminative feature representation of the input text from a pretrained language model like BERT, and can achieve a performance boost when the samples are scarce. Building on top of this discovery, we propose a framework called Labelsemantic augmented meta-learner (LaSAML) to make full use of label semantics. We systematically investigate various factors in this framework and show that it can be plugged into the existing few-shot text classification system. Through extensive experiments, we demonstrate that the few-shot text classification system upgraded by LaSAML can lead to significant performance improvement over its original counterparts.
更多查看译文
AI 理解论文
溯源树
样例
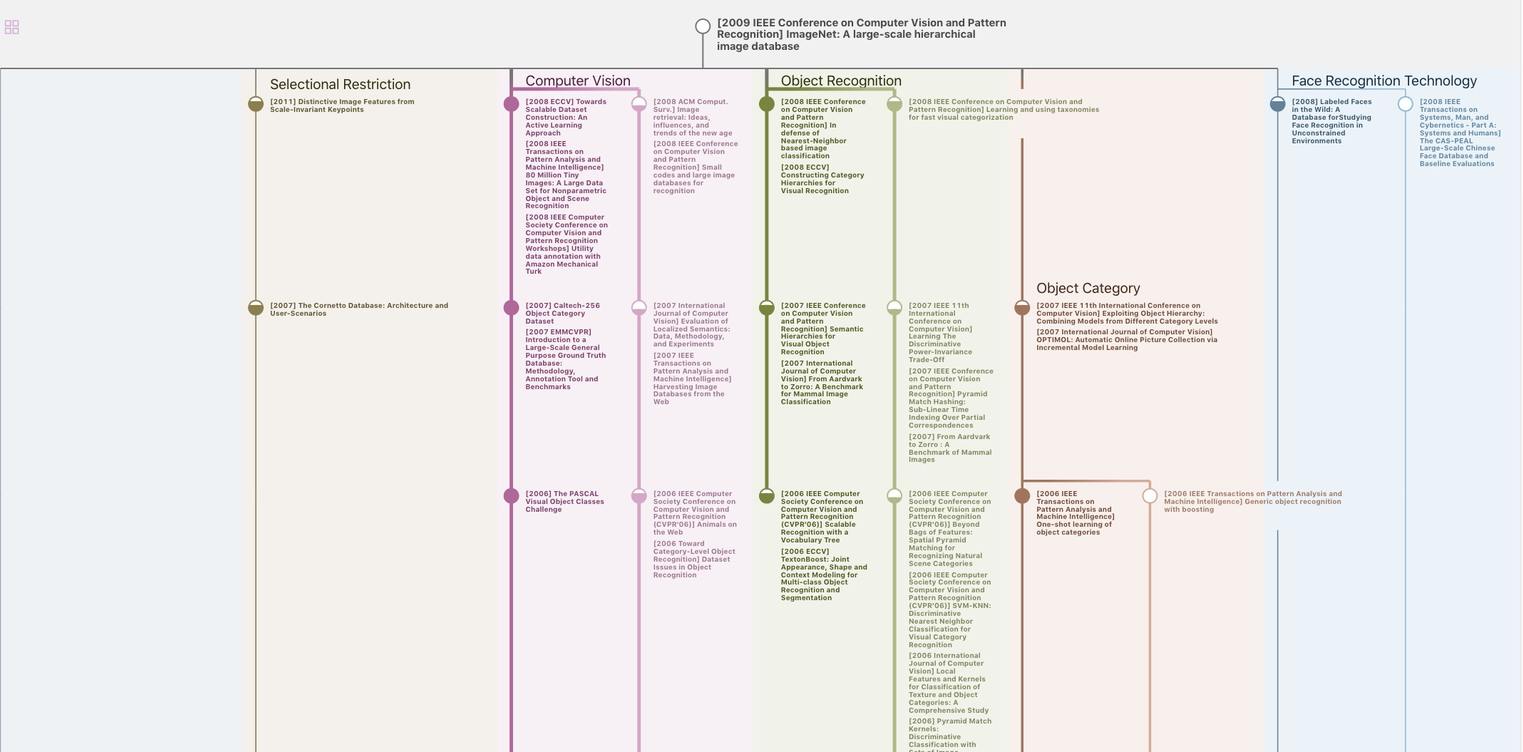
生成溯源树,研究论文发展脉络
Chat Paper
正在生成论文摘要