Task-motion Planning via Tree-based Q-learning Approach for Robotic Object Displacement in Cluttered Spaces.
ICINCO(2021)
摘要
In this paper, a Reinforcement Learning approach to the problem of grasping a target object from clutter by a robotic arm is addressed. A layered architecture is devised to the scope. The bottom layer is in charge of planning robot motion in order to relocate objects while taking into account robot constraints, whereas the top layer takes decision about which obstacles to relocate. In order to generate an optimal sequence of obstacles according to some metrics, a tree is dynamically built where nodes represent sequences of relocated objects and edge weights are updated according to a Q-learning-inspired algorithm. Four different exploration strategies of the solution tree are considered, ranging from a random strategy to a e-Greedy learning-based exploration. The four strategies are compared based on some predefined metrics and in scenarios with different complexity. The learning-based approaches are able to provide optimal relocation sequences despite the high dimensional search space, with the e-Greedy strategy showing better performance, especially in complex scenarios.
更多查看译文
关键词
Motion Planning,Task Planning,Reinforcement Learning
AI 理解论文
溯源树
样例
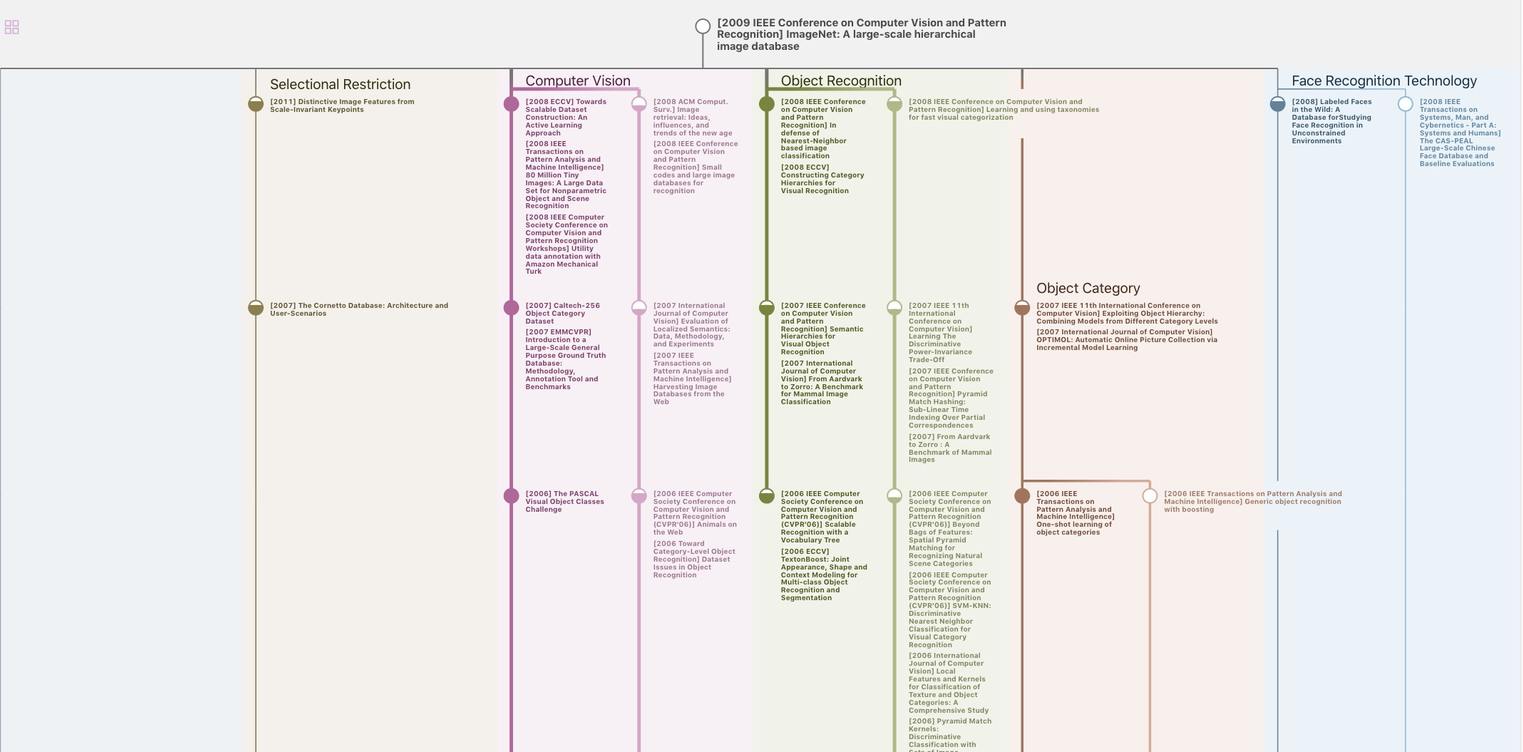
生成溯源树,研究论文发展脉络
Chat Paper
正在生成论文摘要