A Multi-User Service Migration Scheme Based On Deep Reinforcement Learning And Sdn In Mobile Edge Computing
PHYSICAL COMMUNICATION(2021)
摘要
Recently, mobile edge computing(MEC) has attracted considerable research effort, because mobile users can offload some tasks to edge servers that are closer to users than cloud servers for a better computing experience, which can bring enormous potential in next-generation wireless networks(5G). However, when users are moving, they may be far away from the edge server that undertakes the offloading task, resulting in unavoidable service discontinuity and degrading the user experience. Service migration mechanism is very crucial in 5G mobile Internet. In this paper, we propose a novel service migration scheme to support mobility. Our scheme is realized from three aspects: (1) we consider mobile user services can be deployed container virtual machine in corresponding edge servers, and develop container migration strategies to satisfy the trade-off between the users' aware delay and system energy consumption; (2) we further propose a deep reinforcement learning algorithm (DRL) based such multi-user server migration strategy (DRLMSM) to effectively achieve fast decision-making; (3) we build the architecture in Software Defined Network (SDN) framework to verify the practicality and effectiveness of DRLMSM. We conduct extensive experiments, which shows that our DRLMSM scheme outperforms the classical reinforcement learning(RL) algorithm and some other baseline algorithm. (C) 2021 Elsevier B.V. All rights reserved.
更多查看译文
关键词
Mobile edge computing, Container migration, Multi-user mobility, Deep reinforcement learning, Aware delay, Energy consumption
AI 理解论文
溯源树
样例
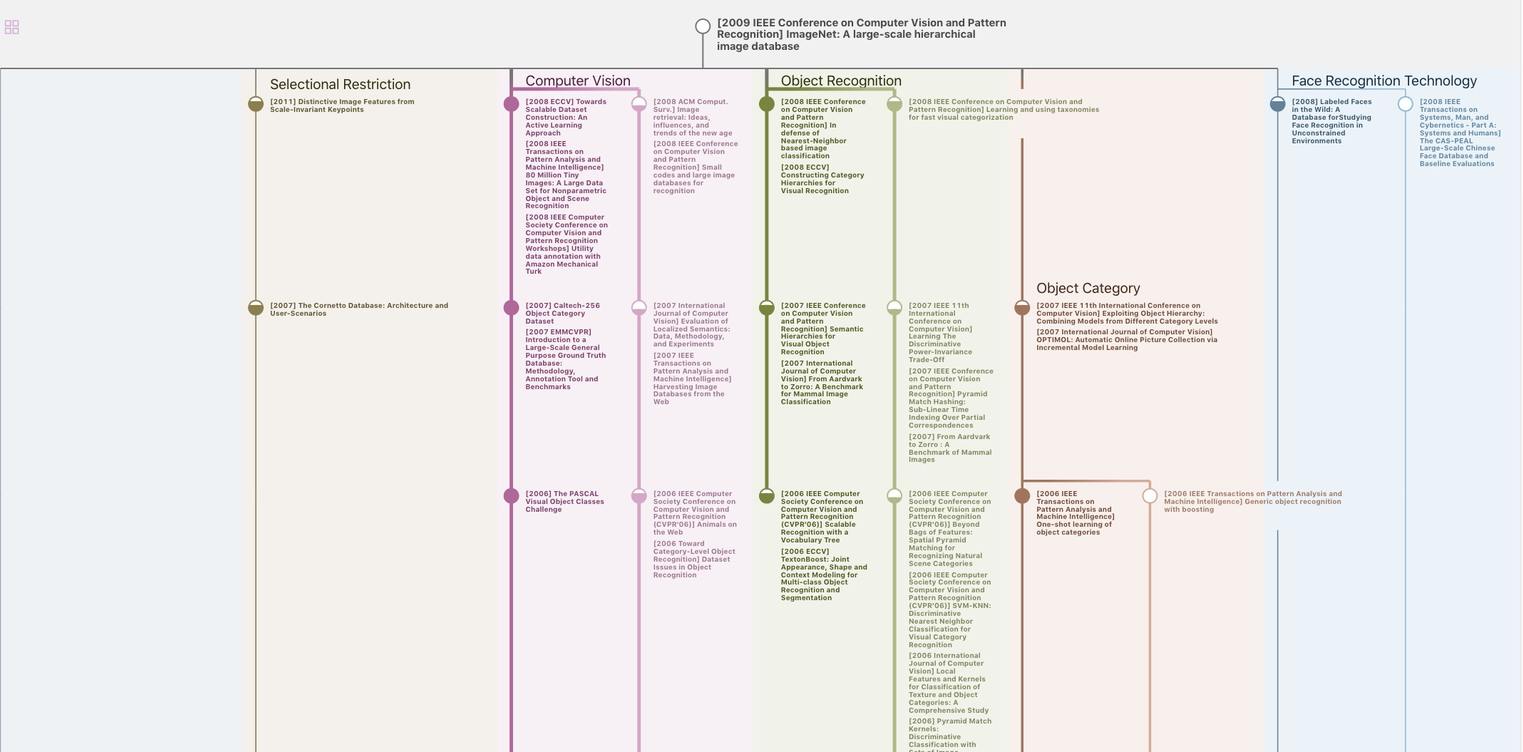
生成溯源树,研究论文发展脉络
Chat Paper
正在生成论文摘要