Performance Analysis of First-order Optimization Methods Using Interpolation Conditions Without Function Evaluations
2021 AMERICAN CONTROL CONFERENCE (ACC)(2021)
摘要
We present necessary and sufficient conditions for the interpolation of a set of points and gradient evaluations by a smooth and strongly convex function. The conditions are expressed as quadratic constraints over doubly hyperdominant matrices. The current literature focuses on interpolation conditions involving both gradient and function evaluations. An extension of these results to use only gradient evaluations requires factorial growth in the number of constraints. Our approach avoids the factorial growth when the interpolation conditions are applied to both finite step and asymptotic optimization algorithm analysis. We present a numerical example demonstrating that the use of these interpolation conditions in the asymptotic analysis setting can produce tighter bounds than are currently available in the literature.
更多查看译文
关键词
quadratic constraints,doubly hyperdominant matrices,asymptotic analysis setting,asymptotic optimization algorithm analysis,factorial growth,strongly convex function,smooth function,gradient evaluations,interpolation conditions,first-order optimization methods
AI 理解论文
溯源树
样例
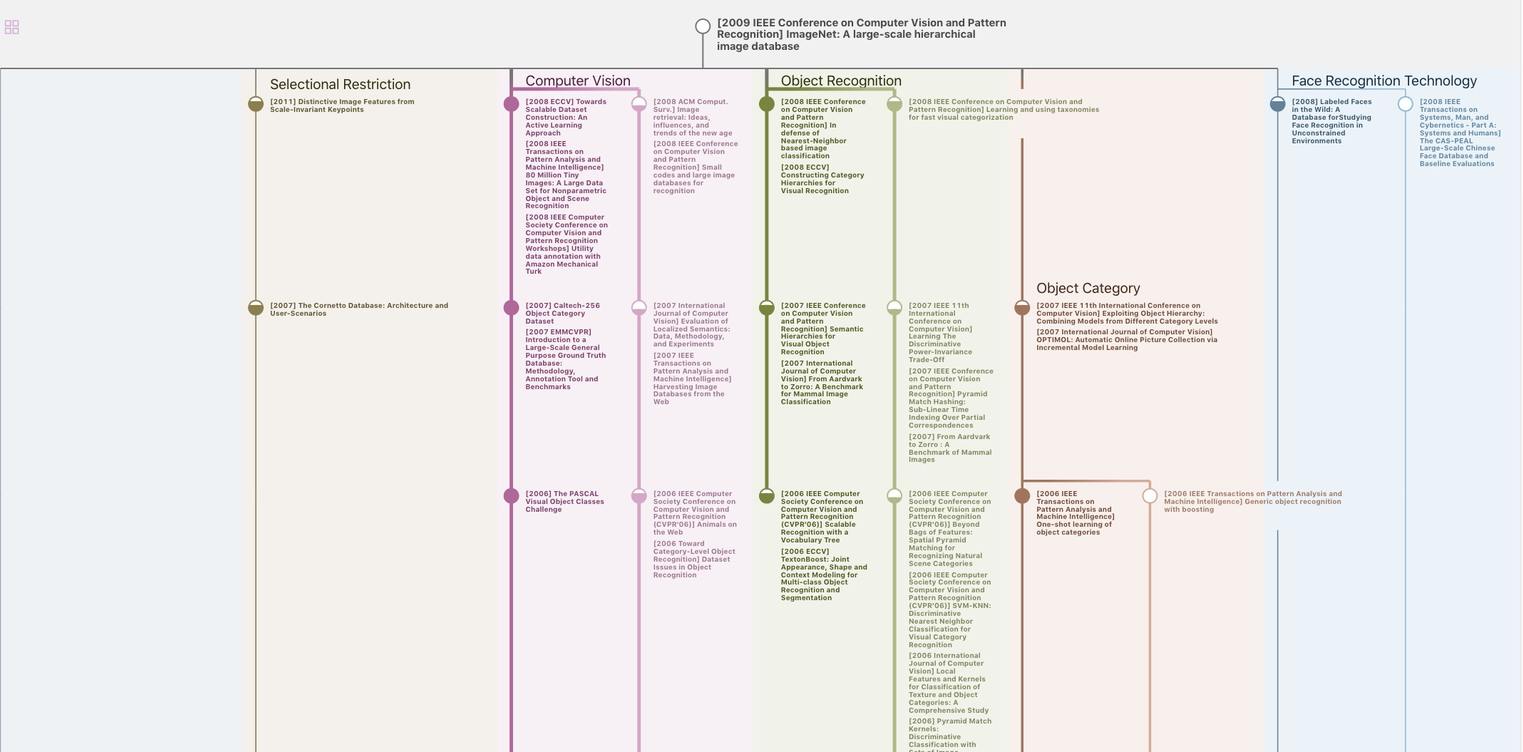
生成溯源树,研究论文发展脉络
Chat Paper
正在生成论文摘要