Automatic vocabulary and graph verification for accurate loop closure detection
JOURNAL OF FIELD ROBOTICS(2022)
摘要
Localizing previously visited places during long-term localization and mapping, that is, loop closure detection (LCD), is a crucial technique to correct accumulated inconsistencies. In common bag-of-words (BoW) model, a visual vocabulary is built to associate features for detecting loops. Currently, methods that build vocabularies off-line determine scales of the vocabulary by trial-and-error, which results in unreasonable feature association. Moreover, the detection precision of the algorithm declines due to perceptual aliasing given that the BoW-based method ignores the positions of visual features. To build the optimal vocabulary automatically and eliminate human heuristics, we propose a natural convergence criterion based on the comparison between the radii of nodes and the drifts of feature descriptors in vocabulary construction. Furthermore, a novel topological graph verification method is proposed for validating loop candidates, which can effectively distinguish visual ambiguities by involving geometrical position of features and thus improve the precision of LCD. Experiments on various public datasets verify the effectiveness of our proposed approach.
更多查看译文
关键词
bag of words, feature association, loop closure detection, simultaneous localization and mapping (SLAM)
AI 理解论文
溯源树
样例
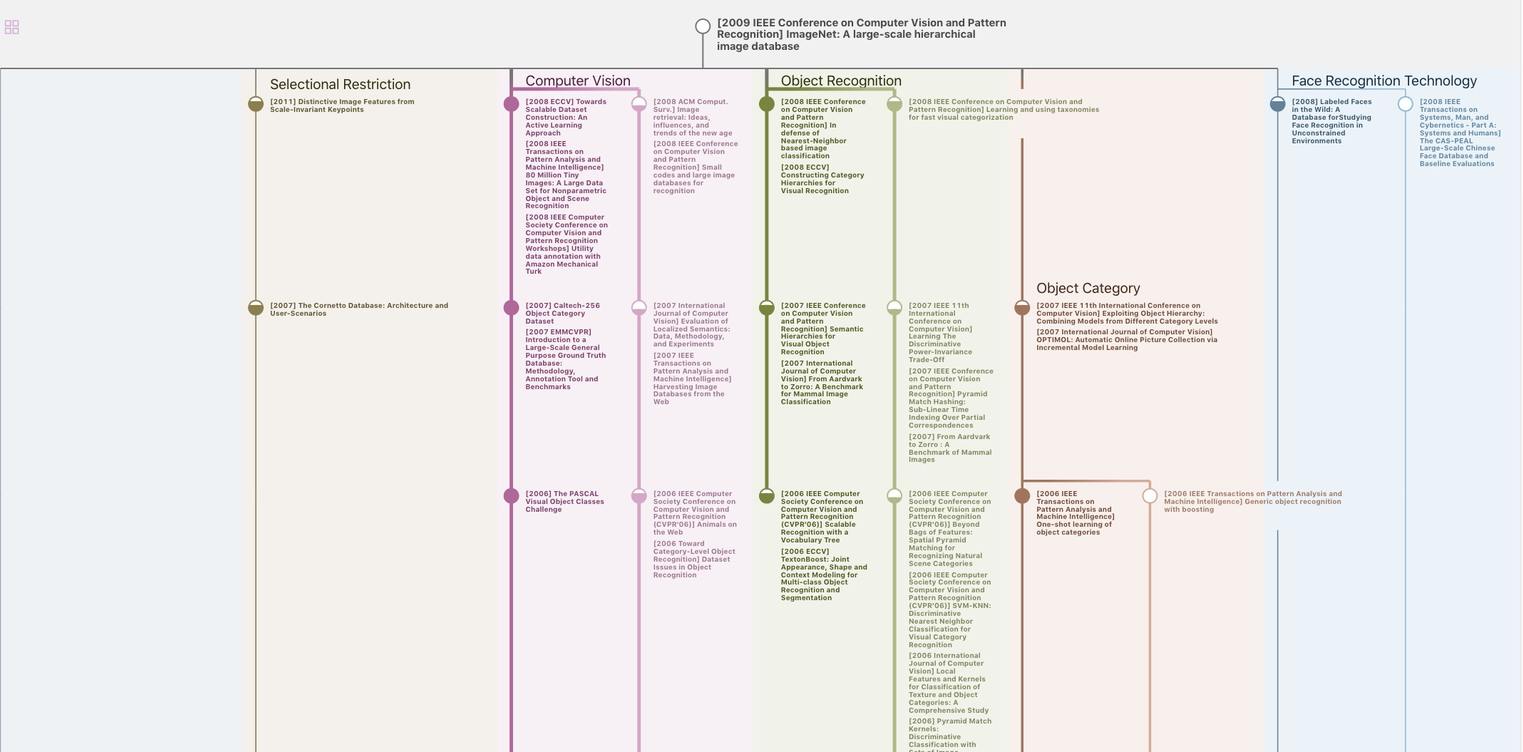
生成溯源树,研究论文发展脉络
Chat Paper
正在生成论文摘要