Learning Maritime Obstacle Detection from Weak Annotations by Scaffolding
2022 IEEE WINTER CONFERENCE ON APPLICATIONS OF COMPUTER VISION (WACV 2022)(2022)
摘要
Coastal water autonomous boats rely on robust perception methods for obstacle detection and timely collision avoidance. The current state-of-the-art is based on deep segmentation networks trained on large datasets. Per-pixel ground truth labeling of such datasets, however, is labor-intensive and expensive. We observe that far less information is required for practical obstacle avoidance - the location of water edge on static obstacles like shore and approximate location and bounds of dynamic obstacles in the water is sufficient to plan a reaction. We propose a new scaffolding learning regime (SLR) that allows training obstacle detection segmentation networks only from such weak annotations, thus significantly reducing the cost of ground-truth labeling. Experiments show that maritime obstacle segmentation networks trained using SLR substantially outperform the same networks trained with dense ground truth labels, despite a significant reduction in labelling effort. Thus accuracy is not sacrificed for labelling simplicity but is in fact improved, which is a remarkable result.
更多查看译文
关键词
Vision for Aerial/Drone/Underwater/Ground Vehicles Object Detection/Recognition/Categorization,Segmentation,Grouping and Shape,Transfer,Few-shot,Semi- and Un- supervised Learning,Vision for Robotics
AI 理解论文
溯源树
样例
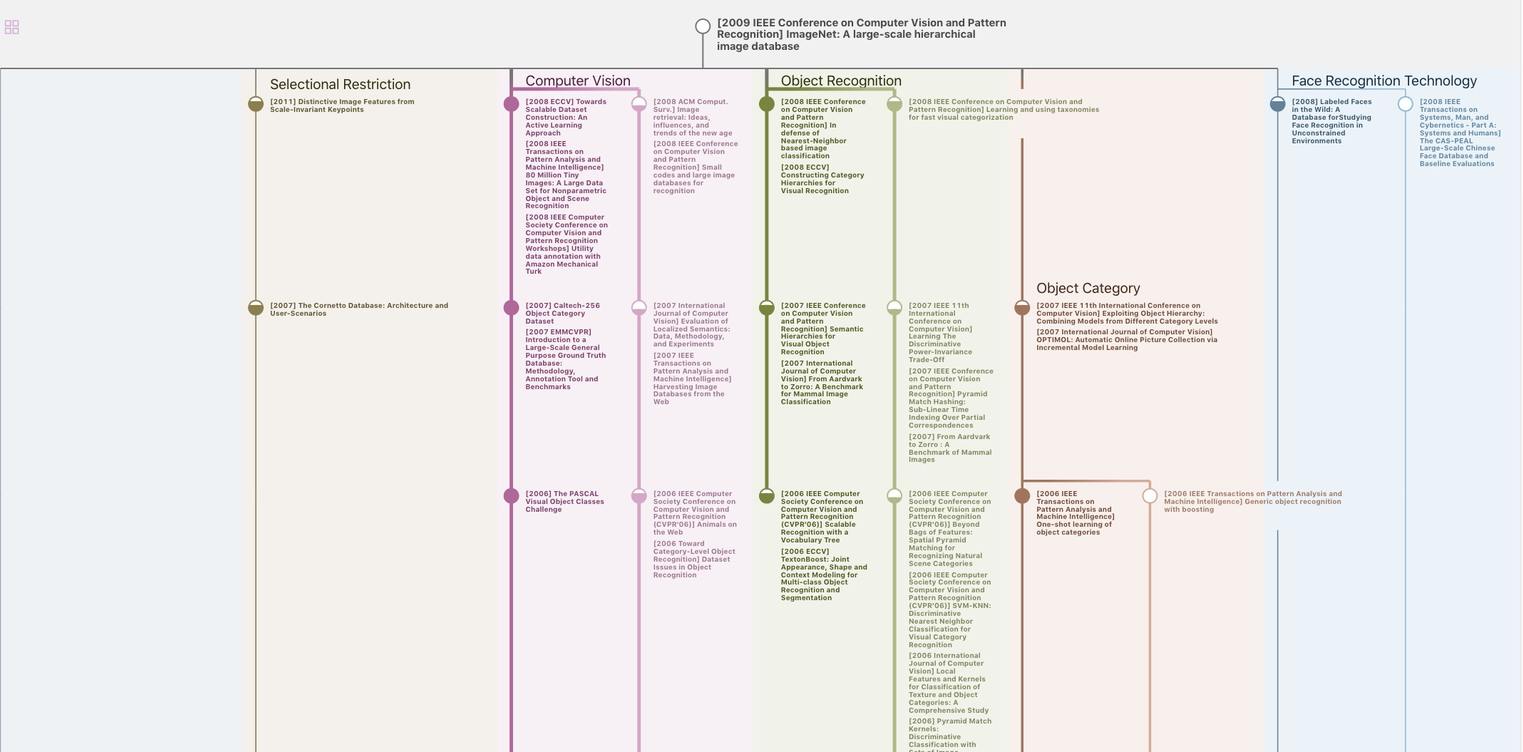
生成溯源树,研究论文发展脉络
Chat Paper
正在生成论文摘要