The Rise of “security and Privacy”: Bibliometric Analysis of Computer Privacy Research
INTERNATIONAL JOURNAL OF INFORMATION SECURITY(2024)
Abstract
The study of security and computer privacy has become a significant focus in security and privacy research. To reflect a website's, service's, or app's privacy policies, they're frequently used as a beginning step for researchers investigating the reliability of stated data regulations, user comprehension of policy, or user control methods. It's challenging to collect information about privacy practices from Internet resources like websites and mobile applications for analysis because of the wide variations in the structure, presentation, and content. Most computer privacy studies attempt to test new methods for detecting, classifying, and analyzing computer privacy content. However, numerous papers have been published to promote research activities, and no trace of any bibliometric analysis work on computer privacy demonstrates research trends. By conducting a thorough analysis of computer privacy studies, it searches the Scopus database, which contains over 2000 papers published between 1976 and 2020. Using the bibliometric analysis technique, this study examines research activity in Europe, South America, and other continents. This work investigated the number of papers published, citations, research area, keywords, institutions, topics, and researchers in detail. An overview of the research efforts is followed by listing the words into a classification of computer privacy analysis tools, emphasizing the significance of a computer privacy research study. According to the investigation findings, there are numerous significant implications of research efforts in Europe compared to other continents. Finally, we summarize the review findings for each part by highlighting potential future research directions.
MoreTranslated text
Key words
Privacy,Computer privacy,Security,Bibliometric analysis,IoT computer privacy
求助PDF
上传PDF
View via Publisher
AI Read Science
AI Summary
AI Summary is the key point extracted automatically understanding the full text of the paper, including the background, methods, results, conclusions, icons and other key content, so that you can get the outline of the paper at a glance.
Example
Background
Key content
Introduction
Methods
Results
Related work
Fund
Key content
- Pretraining has recently greatly promoted the development of natural language processing (NLP)
- We show that M6 outperforms the baselines in multimodal downstream tasks, and the large M6 with 10 parameters can reach a better performance
- We propose a method called M6 that is able to process information of multiple modalities and perform both single-modal and cross-modal understanding and generation
- The model is scaled to large model with 10 billion parameters with sophisticated deployment, and the 10 -parameter M6-large is the largest pretrained model in Chinese
- Experimental results show that our proposed M6 outperforms the baseline in a number of downstream tasks concerning both single modality and multiple modalities We will continue the pretraining of extremely large models by increasing data to explore the limit of its performance
Upload PDF to Generate Summary
Must-Reading Tree
Example
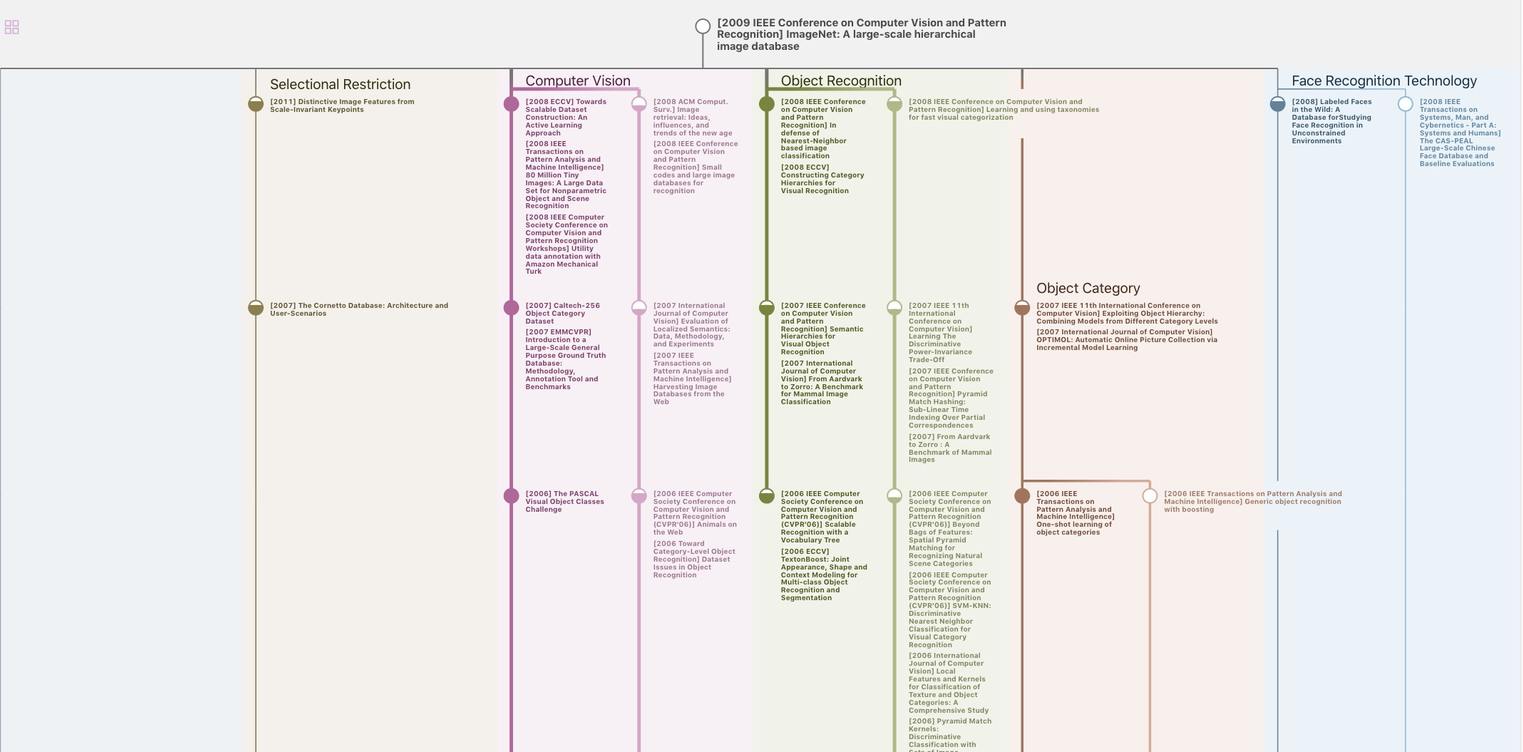
Generate MRT to find the research sequence of this paper
Related Papers
1999
被引用1436 | 浏览
2006
被引用84 | 浏览
2011
被引用1133 | 浏览
2012
被引用20 | 浏览
2009
被引用8 | 浏览
2019
被引用78 | 浏览
Analysis and Text Classification of Privacy Policies from Rogue and Top-100 Fortune Global Companies
2019
被引用4 | 浏览
2019
被引用2 | 浏览
2021
被引用13 | 浏览
Data Disclaimer
The page data are from open Internet sources, cooperative publishers and automatic analysis results through AI technology. We do not make any commitments and guarantees for the validity, accuracy, correctness, reliability, completeness and timeliness of the page data. If you have any questions, please contact us by email: report@aminer.cn
Chat Paper