Time-based Dynamic Controllability of Disjunctive Temporal Networks with Uncertainty: A Tree Search Approach with Graph Neural Network Guidance
arxiv(2021)
摘要
Scheduling in the presence of uncertainty is an area of interest in artificial intelligence due to the large number of applications. We study the problem of dynamic controllability (DC) of disjunctive temporal networks with uncertainty (DTNU), which seeks a strategy to satisfy all constraints in response to uncontrollable action durations. We introduce a more restricted, stronger form of controllability than DC for DTNUs, time-based dynamic controllability (TDC), and present a tree search approach to determine whether or not a DTNU is TDC. Moreover, we leverage the learning capability of a message passing neural network (MPNN) as a heuristic for tree search guidance. Finally, we conduct experiments for which the tree search shows superior results to state-of-the-art timed-game automata (TGA) based approaches. We observe that using an MPNN for tree search guidance leads to a significant increase in solving performance and scalability to harder DTNU problems.
更多查看译文
关键词
disjunctive temporal networks,dynamic controllability,uncertainty,neural networks,time-based
AI 理解论文
溯源树
样例
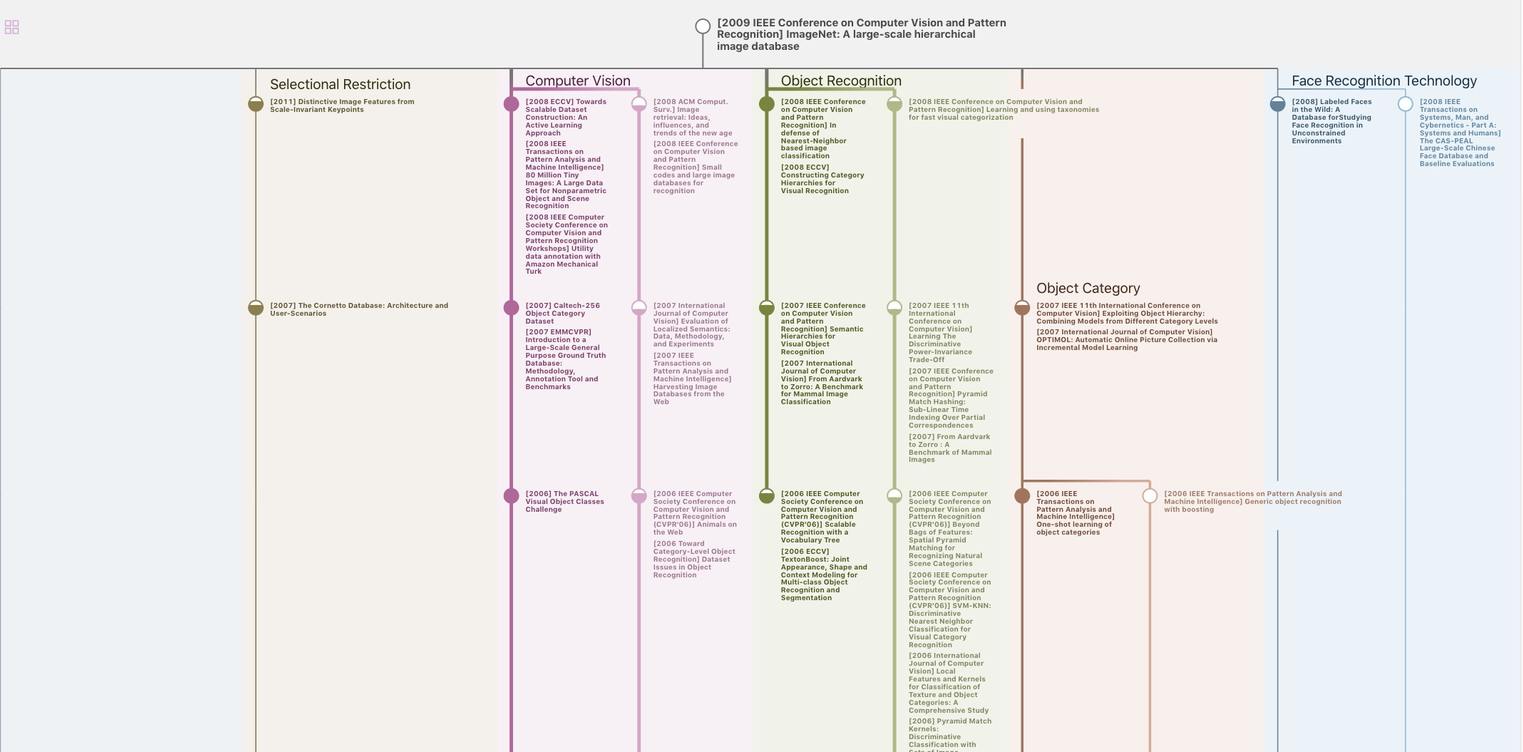
生成溯源树,研究论文发展脉络
Chat Paper
正在生成论文摘要