Hierarchical Representations and Explicit Memory: Learning Effective Navigation Policies on 3D Scene Graphs using Graph Neural Networks
IEEE International Conference on Robotics and Automation(2022)
摘要
Representations are crucial for a robot to learn effective navigation policies. Recent work has shown that mid-level perceptual abstractions, such as depth estimates or 2D semantic segmentation, lead to more effective policies when provided as observations in place of raw sensor data (e.g., RGB images). However, such policies must still learn latent three-dimensional scene properties from mid-level abstractions. In contrast, high-level, hierarchical representations such as 3D scene graphs explicitly provide a scene's geometry, topology, and semantics, making them compelling representations for navigation. In this work, we present a reinforcement learning framework that leverages high-level hierarchical representations to learn navigation policies. Towards this goal, we propose a graph neural network architecture and show how to embed a 3D scene graph into an agent-centric feature space, which enables the robot to learn policies that map 3D scene graphs to a platform-agnostic control space (e.g., go straight, turn left). For each node in the scene graph, our method uses features that capture occupancy and semantic content, while explicitly retaining memory of the robot trajectory. We demonstrate the effectiveness of our method against commonly used visuomotor policies in a challenging multi-object search task. These experiments and supporting ablation studies show that our method leads to more effective object search behaviors, exhibits improved long-term memory, and successfully leverages hierarchical information to guide its navigation objectives.
更多查看译文
关键词
explicit memory,effective navigation policies,graph neural networks,mid-level perceptual abstractions,2D semantic segmentation,effective policies,raw sensor data,three-dimensional scene properties,mid-level abstractions,compelling representations,reinforcement learning framework,high-level hierarchical representations,graph neural network architecture,scene graph,agent-centric feature space,map 3D scene graphs,commonly used visuomotor policies,effective object search behaviors,leverages hierarchical information,navigation objectives
AI 理解论文
溯源树
样例
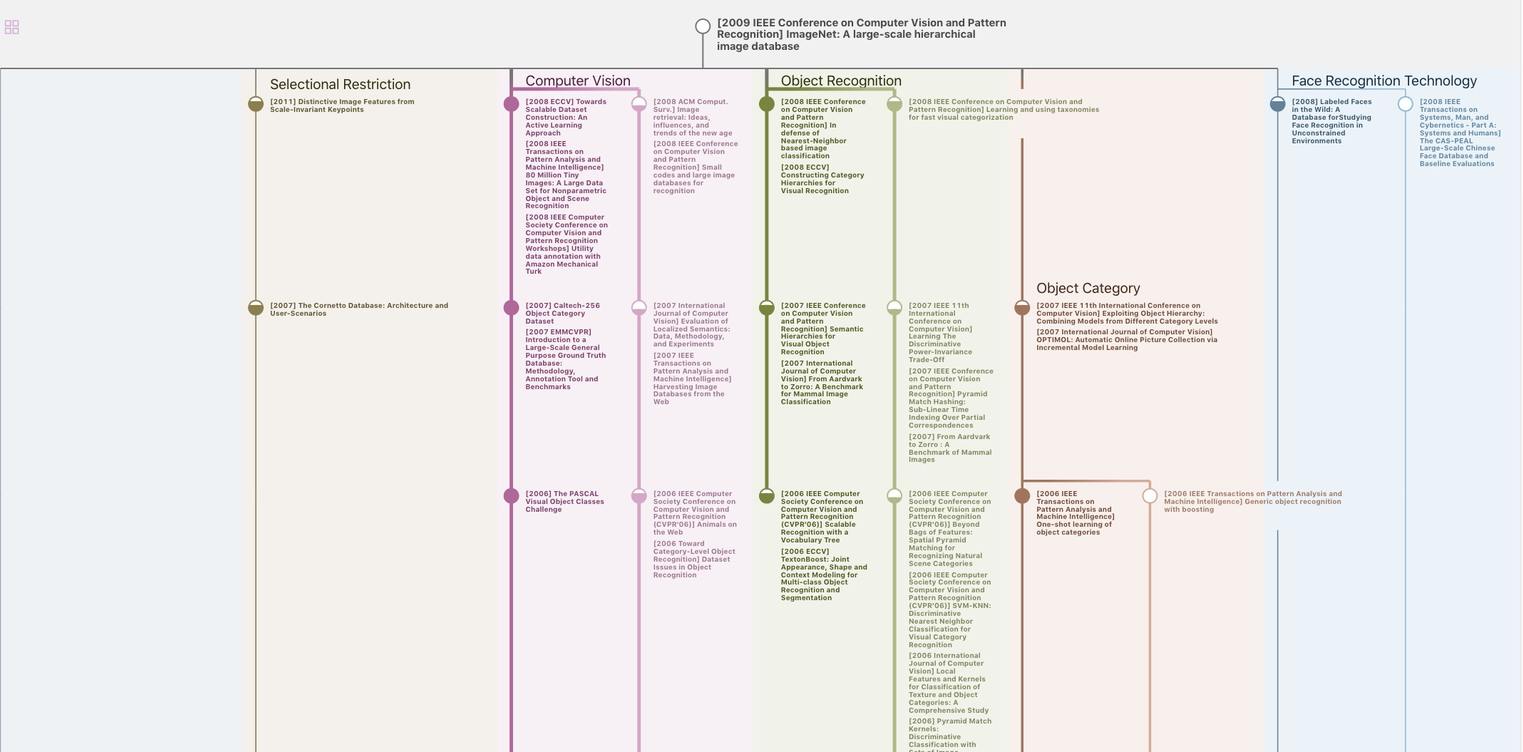
生成溯源树,研究论文发展脉络
Chat Paper
正在生成论文摘要