Machine Learning-Based Auto-Scaler for Video Conferencing Systems
2021 IEEE 7th International Conference on Network Softwarization (NetSoft)(2021)
摘要
Video conferencing systems have been developed for a long time. However, due to COVID-19, people came to realize the importance of such systems that their widespread usage increased drastically. With the growing number of users of video conferencing systems, it is essential to be able to manage the system better. Even though Network Function Virtualization (NFV) has helped in handling the dynamic nature of video conferencing systems by providing the ability to deploy and/or remove virtual instances, being able to manage the virtual instances efficiently and optimally is a necessity. One of the main problems to be solved to achieve this goal is the auto-scaling problem. In this problem, an auto-scaling agent has to decide whether the number of a virtual instance type has to be increased, decreased, or maintained. In this paper, we designed and experimented a new auto-scaling algorithm by using Reinforcement Learning (RL), specifically the Deep Q-Network (DQN) algorithm. The experiment was done in both emulated and simulated environments. We then evaluated our proposed approach by comparing it with a threshold-based auto-scaler, the baseline algorithm. The results showed that the DQN-based auto-scaler performed better than the baseline algorithm, using fewer virtual instances with a better quality of service overall, as well as being more robust to shifting data distribution. Overall, the applications of DQN on auto-scaling algorithms for cost-effective and high-performance video conferencing systems are promising.
更多查看译文
关键词
Video Conferencing System,Network Function Virtualization (NFV),Auto-Scaling,Reinforcement Learning (RL),Deep Q-Network (DQN)
AI 理解论文
溯源树
样例
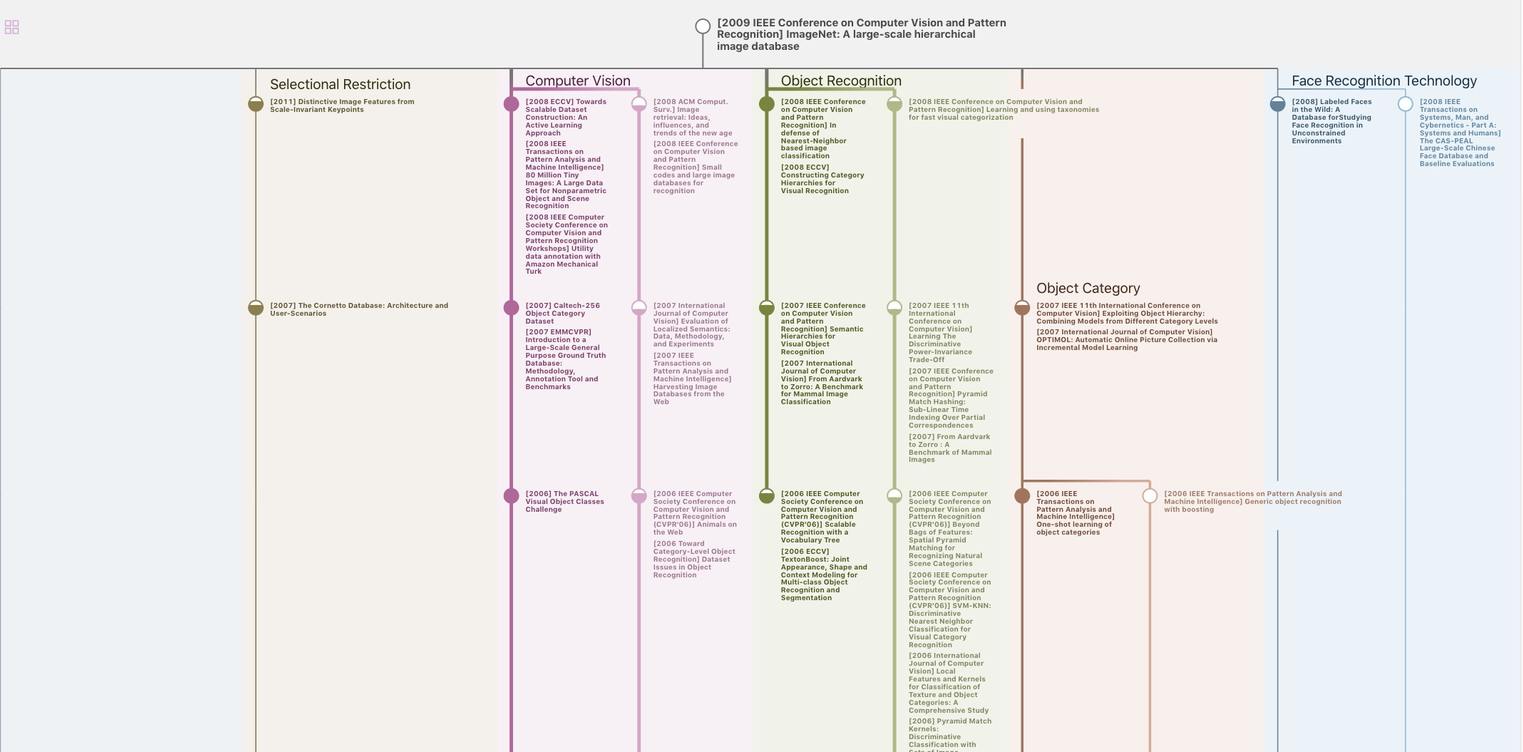
生成溯源树,研究论文发展脉络
Chat Paper
正在生成论文摘要