Damage Detection in Structural Health Monitoring with Spiking Neural Networks
2021 IEEE International Workshop on Metrology for Industry 4.0 & IoT (MetroInd4.0&IoT)(2021)
摘要
Structural Health Monitoring (SHM) is an evolving research field involving internet-of-things and machine-learning technologies. Recent research in this field demonstrated the effectiveness of low-cost MEMS accelerometers to monitor the vibrations of buildings, and neural networks analyse generated data streams. In this work, we propose a novel SHM approach using Spiking Neural Networks (SNNs) applied to MEMS data to detect infrastructural damages in a motorway bridge. SNNs are brain-inspired network models that are promising as being more compact and potentially energy-efficient than traditional networks. In particular, Long Short-Term SNN (LSNN) are very effective in analysing streams of data, but they require a nontrivial learning process. We study the feasibility of LSNNs for SHM, and we compare their accuracy with alternative artificial neural network (ANN) models. We demonstrate that SNN can effectively discriminate whether a structure is in a healthy or damaged condition with an accuracy level similar to ANN. To this purpose, we exploited a state-of-the-art fast training algorithm that approximates the Back Propagation Through Time (BPTT). We also show that inference times are compliant with real-time SHM requirements.
更多查看译文
关键词
Spiking Neural Networks,Machine Learning,Structural Health Monitoring
AI 理解论文
溯源树
样例
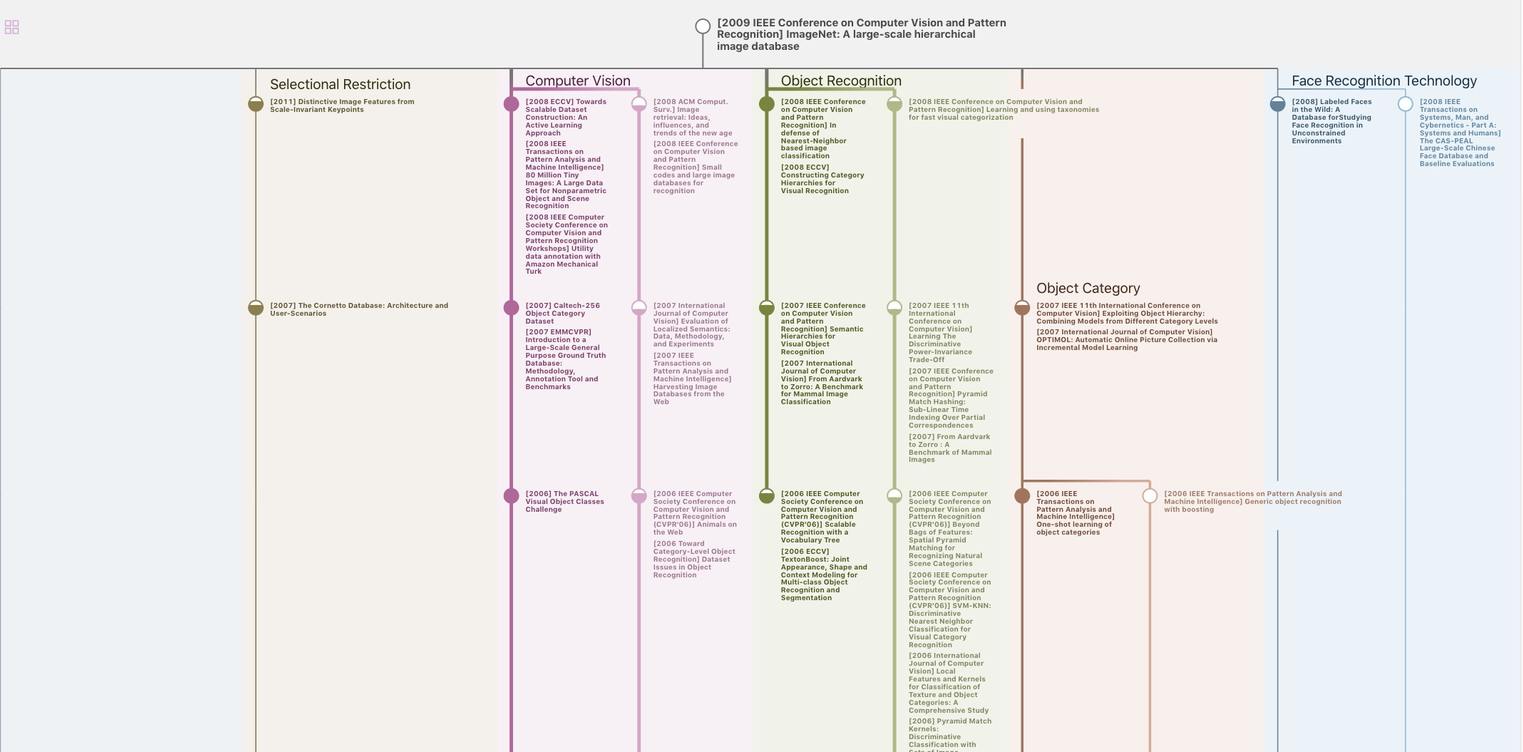
生成溯源树,研究论文发展脉络
Chat Paper
正在生成论文摘要