Treatment outcome clustering patterns correspond to discrete asthma phenotypes in children
Asthma research and practice(2021)
摘要
Despite widely and regularly used therapy asthma in children is not fully controlled. Recognizing the complexity of asthma phenotypes and endotypes imposed the concept of precision medicine in asthma treatment. By applying machine learning algorithms assessed with respect to their accuracy in predicting treatment outcome, we have successfully identified 4 distinct clusters in a pediatric asthma cohort with specific treatment outcome patterns according to changes in lung function (FEV 1 and MEF 50 ), airway inflammation (FENO) and disease control likely affected by discrete phenotypes at initial disease presentation, differing in the type and level of inflammation, age of onset, comorbidities, certain genetic and other physiologic traits. The smallest and the largest of the 4 clusters- 1 ( N = 58) and 3 ( N = 138) had better treatment outcomes compared to clusters 2 and 4 and were characterized by more prominent atopic markers and a predominant allelic (A allele) effect for rs37973 in the GLCCI1 gene previously associated with positive treatment outcomes in asthmatics. These patients also had a relatively later onset of disease (6 + yrs). Clusters 2 ( N = 87) and 4 ( N = 64) had poorer treatment success, but varied in the type of inflammation (predominantly neutrophilic for cluster 4 and likely mixed-type for cluster 2), comorbidities (obesity for cluster 2), level of systemic inflammation (highest hsCRP for cluster 2) and platelet count (lowest for cluster 4). The results of this study emphasize the issues in asthma management due to the overgeneralized approach to the disease, not taking into account specific disease phenotypes.
更多查看译文
关键词
Allergy,Asthma phenotypes,Childhood asthma,Clustering,Machine learning,Treatment outcome
AI 理解论文
溯源树
样例
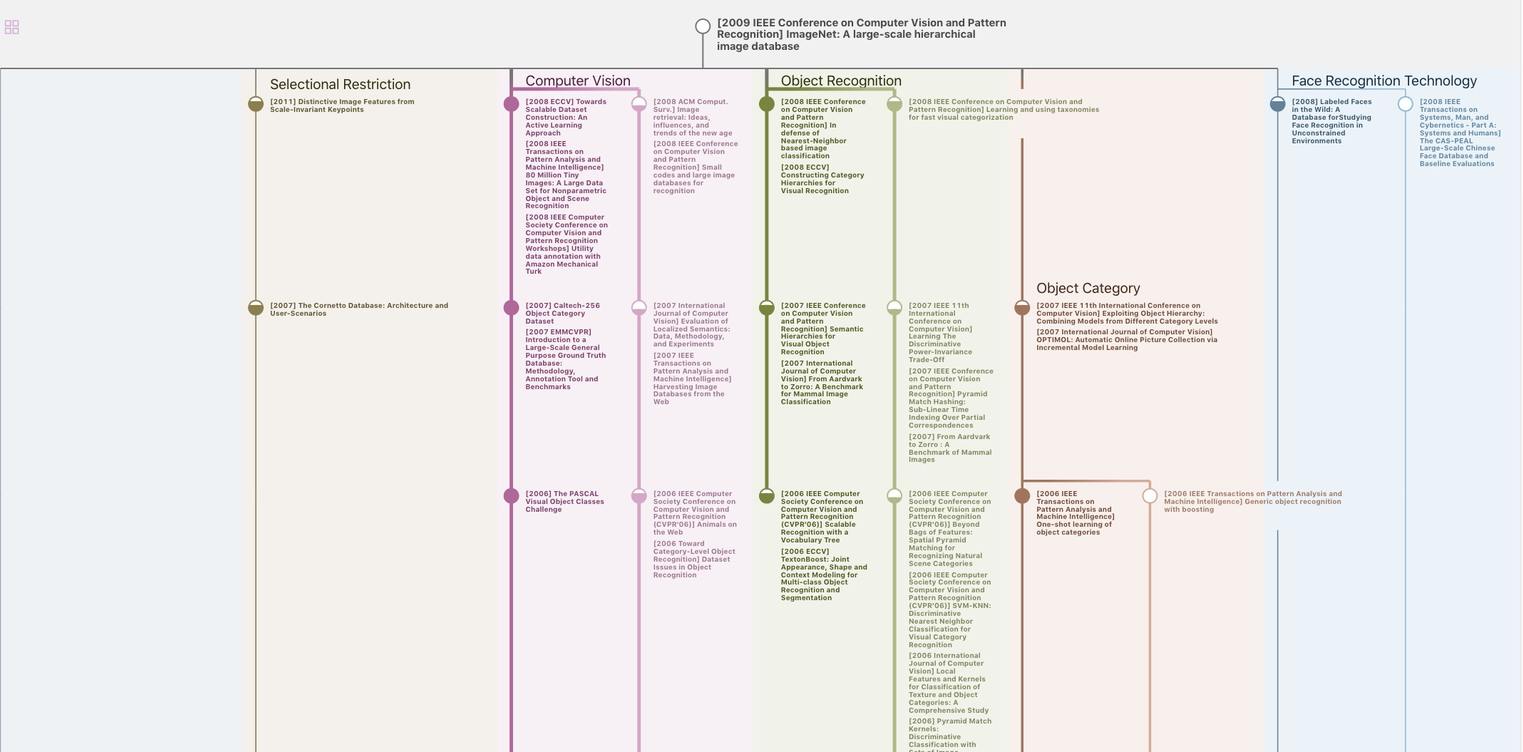
生成溯源树,研究论文发展脉络
Chat Paper
正在生成论文摘要