Scoring model to predict low image quality of drug-eluting stent evaluated by computed tomography coronary angiography
HEART AND VESSELS(2021)
摘要
Evaluation of in-stent restenosis (ISR) by computed tomography coronary angiography (CTCA) is less invasive but often impossible. We aimed to create a scoring model for predicting which drug-eluting stents (DES) cannot be evaluated with CTCA. We enrolled 757 consecutive implanted DES assessed with CTCA. Non-diagnostic evaluation was defined as poor/not evaluative by two different observers. These stents were randomly divided into a derivation ( n = 379) and validation ( n = 378) group. In the derivation group, we assessed predictors using logistic regression analysis and created a scoring model that would stratify non-diagnostic evaluation of DES-ISR. The validity of this scoring model was evaluated in the validation group using receiver-operating characteristic analysis. The percentage of non-diagnostic stents was 19/21% in the derivation/validation group ( p = 0.71). Non-diagnostic evaluation was independently associated with implanted stent diameter (2.25–2.5. vs. 2.5–3 vs. > 3.0 mm), severe calcification, stent-in-stent lesion, and type of DES (stainless vs. CoCr vs. PtCr) in the derivation group. The predicting system of implanted DES non-diagnostic by CTCA (PIDENT) for non-diagnostic evaluation, including these four baseline factors, was derived (C-statistic = 0.86 in derivation group, cutoff: 8 points). The PIDENT score had a high predictive value for non-diagnostic DES in the validation model (C-statistic = 0.87, sensitivity 86%, specificity 74%, cutoff 8 points, p < 0.001). The PIDENT score, consisting of baseline characteristics including implanted stent diameter, severe calcification, stent-in-stent lesion, and type of DES, could identify non-diagnostic evaluation of DES-ISR with CTCA. The PIDENT score was valuable in reducing nonevaluable and meaningless CTCA for DES-ISR.
更多查看译文
关键词
Computed tomography angiography,Drug-eluting stent,In-stent restenosis
AI 理解论文
溯源树
样例
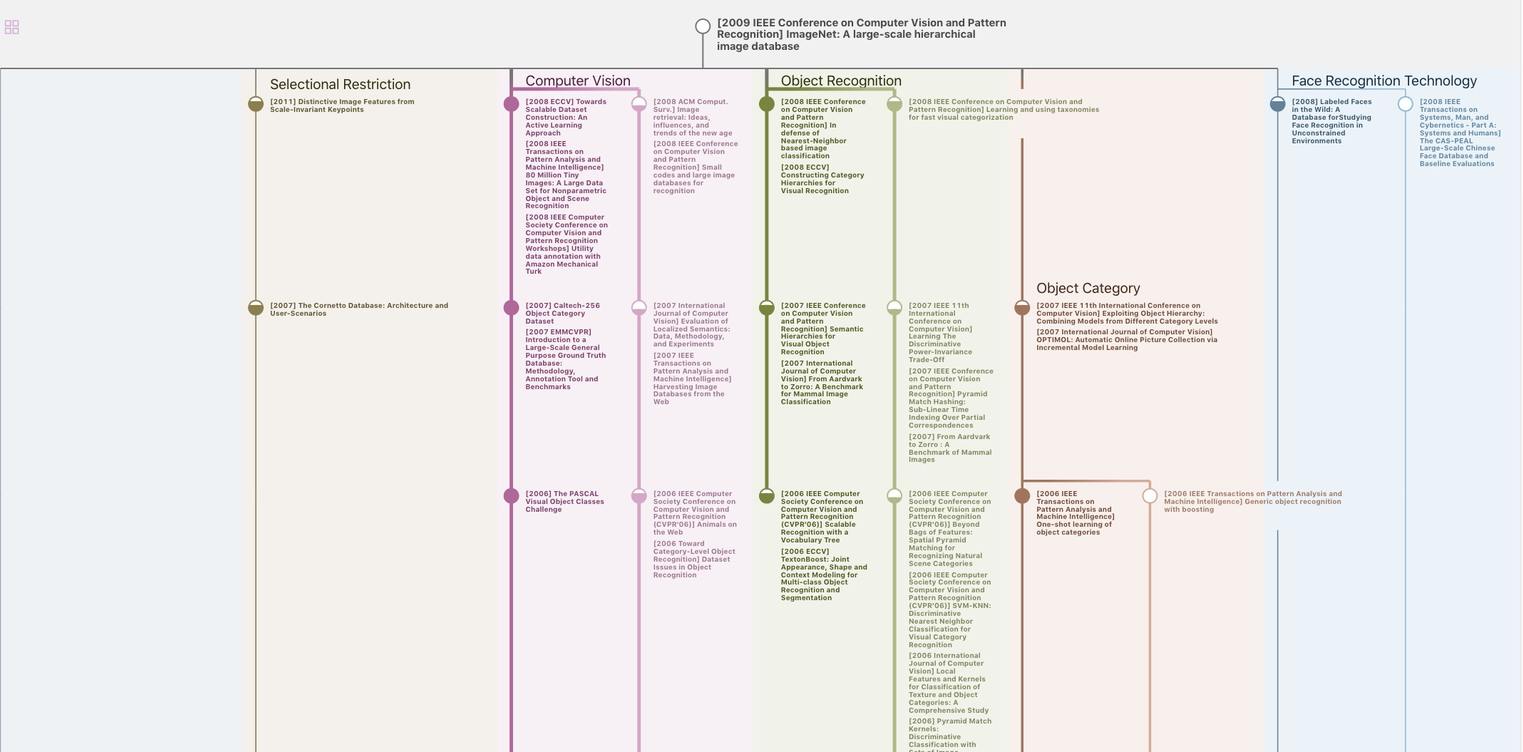
生成溯源树,研究论文发展脉络
Chat Paper
正在生成论文摘要