Simultaneous Semantic and Collision Learning for 6-DoF Grasp Pose Estimation
2021 IEEE/RSJ INTERNATIONAL CONFERENCE ON INTELLIGENT ROBOTS AND SYSTEMS (IROS)(2021)
摘要
Grasping in cluttered scenes has always been a great challenge for robots, due to the requirement of the ability to well understand the scene and object information. Previous works usually assume that the geometry information of the objects is available, or utilize a step-wise, multi-stage strategy to predict the feasible 6-DoF grasp poses. In this work, we propose to formalize the 6-DoF grasp pose estimation as a simultaneous multi-task learning problem. In a unified framework, we jointly predict the feasible 6-DoF grasp poses, instance semantic segmentation, and collision information. The whole framework is jointly optimized and end-to-end differentiable. Our model is evaluated on large-scale benchmarks as well as the real robot system. On the public dataset, our method outperforms prior state-of-the-art methods by a large margin (+4.08 AP). We also demonstrate the implementation of our model on a real robotic platform and show that the robot can accurately grasp target objects in cluttered scenarios with a high success rate. Project link: https://openbyterobotics.github.io/sscl.
更多查看译文
关键词
collision learning,simultaneous semantic
AI 理解论文
溯源树
样例
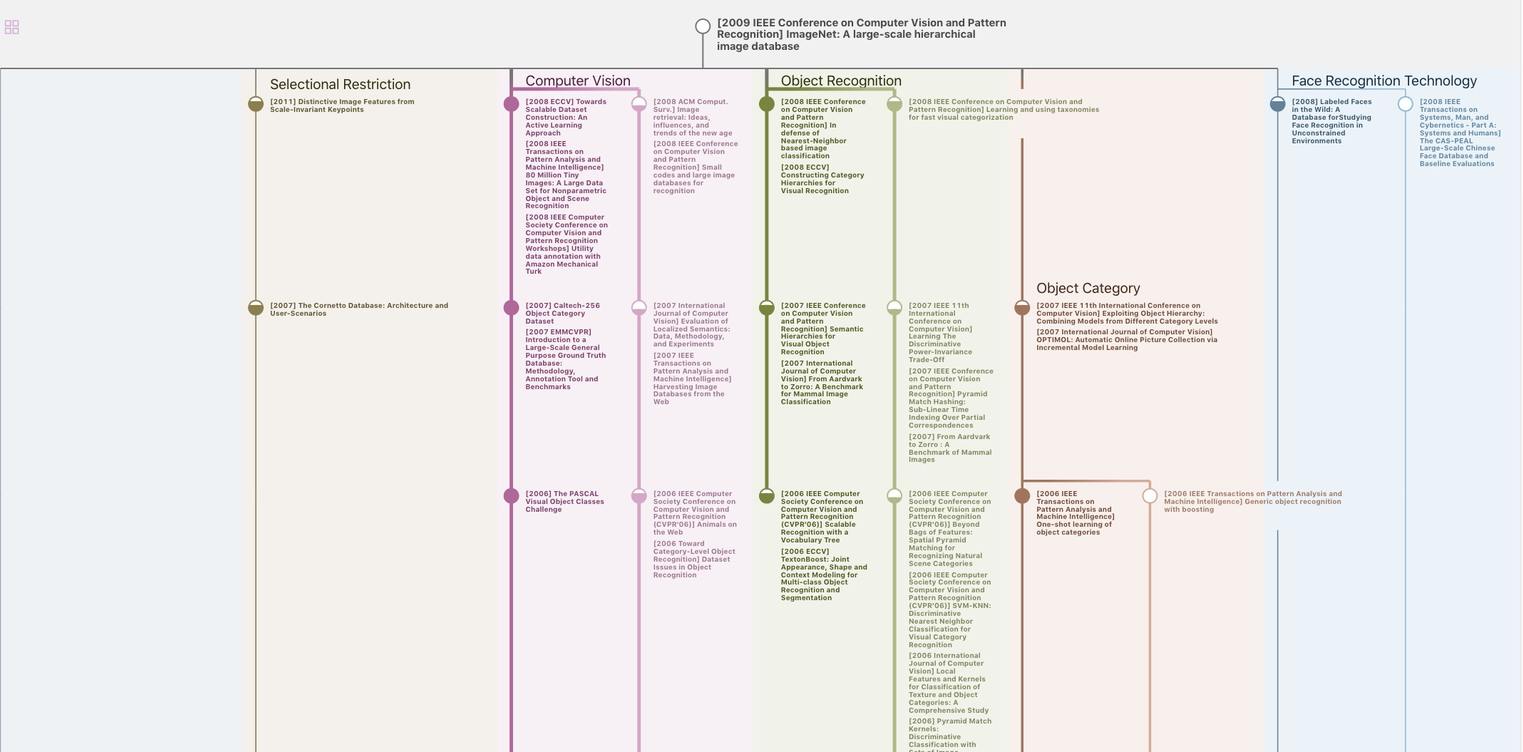
生成溯源树,研究论文发展脉络
Chat Paper
正在生成论文摘要