Poison Ink: Robust and Invisible Backdoor Attack
IEEE TRANSACTIONS ON IMAGE PROCESSING(2022)
摘要
Recent research shows deep neural networks are vulnerable to different types of attacks, such as adversarial attacks, data poisoning attacks, and backdoor attacks. Among them, backdoor attacks are the most cunning and can occur in almost every stage of the deep learning pipeline. Backdoor attacks have attracted lots of interest from both academia and industry. However, most existing backdoor attack methods are visible or fragile to some effortless pre-processing such as common data transformations. To address these limitations, we propose a robust and invisible backdoor attack called "Poison Ink". Concretely, we first leverage the image structures as target poisoning areas and fill them with poison ink (information) to generate the trigger pattern. As the image structure can keep its semantic meaning during the data transformation, such a trigger pattern is inherently robust to data transformations. Then we leverage a deep injection network to embed such input-aware trigger pattern into the cover image to achieve stealthiness. Compared to existing popular backdoor attack methods, Poison Ink outperforms both in stealthiness and robustness. Through extensive experiments, we demonstrate that Poison Ink is not only general to different datasets and network architectures but also flexible for different attack scenarios. Besides, it also has very strong resistance against many state-of-the-art defense techniques.
更多查看译文
关键词
Toxicology, Ink, Training, Robustness, Data models, Training data, Task analysis, Backdoor attack, stealthiness, robustness, generality, flexibility
AI 理解论文
溯源树
样例
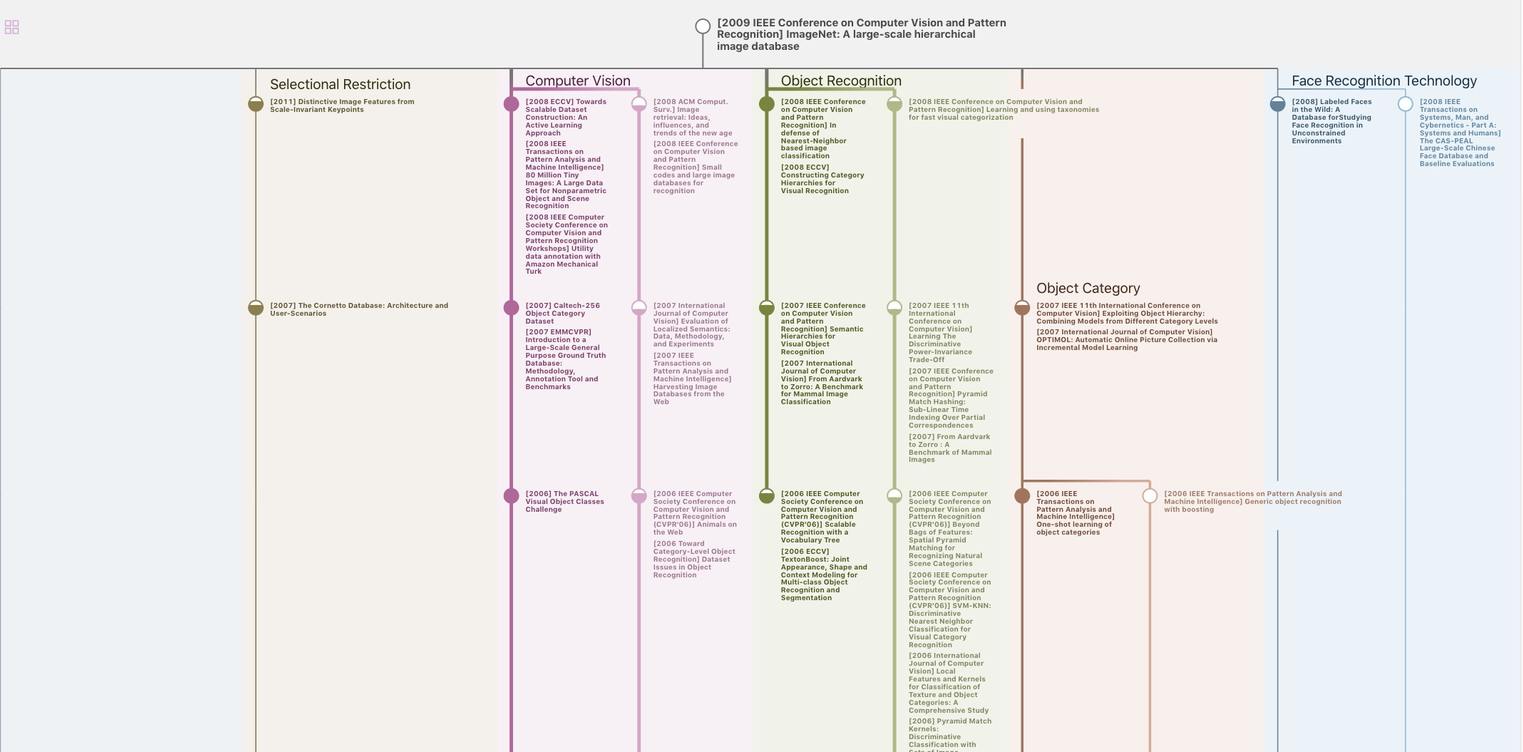
生成溯源树,研究论文发展脉络
Chat Paper
正在生成论文摘要