Sequence model evaluation framework for STARR-seq peak calling
BCB(2021)
摘要
ABSTRACTEnhancers are short regions of non-coding DNA that increase transcription rates of genes despite being located distantly from the genes themselves [5]. Enhancers are identified through experimental techniques such as ChIP-Seq or CUT&RUN with H3K4me1 and H3K27ac histone modifications, self-transcribing active regulatory region sequencing (STARR-Seq), and massively parallel reporter assays (MPRA). Machine learning models have been used in conjunction with experimental data to identify enhancer activity from sequences [3], predict enhancer-transcription factor interactions [4], and decode the enhancer regulatory language [2]. We describe a framework that connects peak calling errors to the prediction accuracy of sequence models. The key assumptions of our framework are that (1) enhancers have consistent sequence patterns that can be used to separate enhancers from control sequences, (2) errors in the training data impact prediction accuracies in predictable ways, and (3) prediction accuracy is a useful proxy for evaluating peak calling accuracy. In the framework, data sets are constructed from peak (positive) and randomly sampled (control) sequences. Machine learning models are trained and evaluated on the sequences in a cross-chromosome (cross-fold) setup. Lastly, precision of the originating peaks are evaluated by calculating true and false positive rates. We applied our framework to evaluate peaks for D. melanogaster STARR-Seq data [1] called with the MACS software [6]. Although designed for ChIP-Seq data, MACS can be used to process other types of data, but users must be careful about parameter choices. We evaluated different parameter combinations with our framework and visual comparisons of called peaks. True and false positive rates ranged from a high of 88.0% to a low of 74.7% and from a low of 18.6% to a high of 49.4%, respectively. The default MACS parameters produced the highest true and lowest false positive rates, suggesting that the default parameters are also suitable for STARR-Seq data. Our results demonstrate the utility of our framework through a practical application and provide a base for future development.
更多查看译文
关键词
peak calling, DNA enrichment assays, enhancers, sequence modeld
AI 理解论文
溯源树
样例
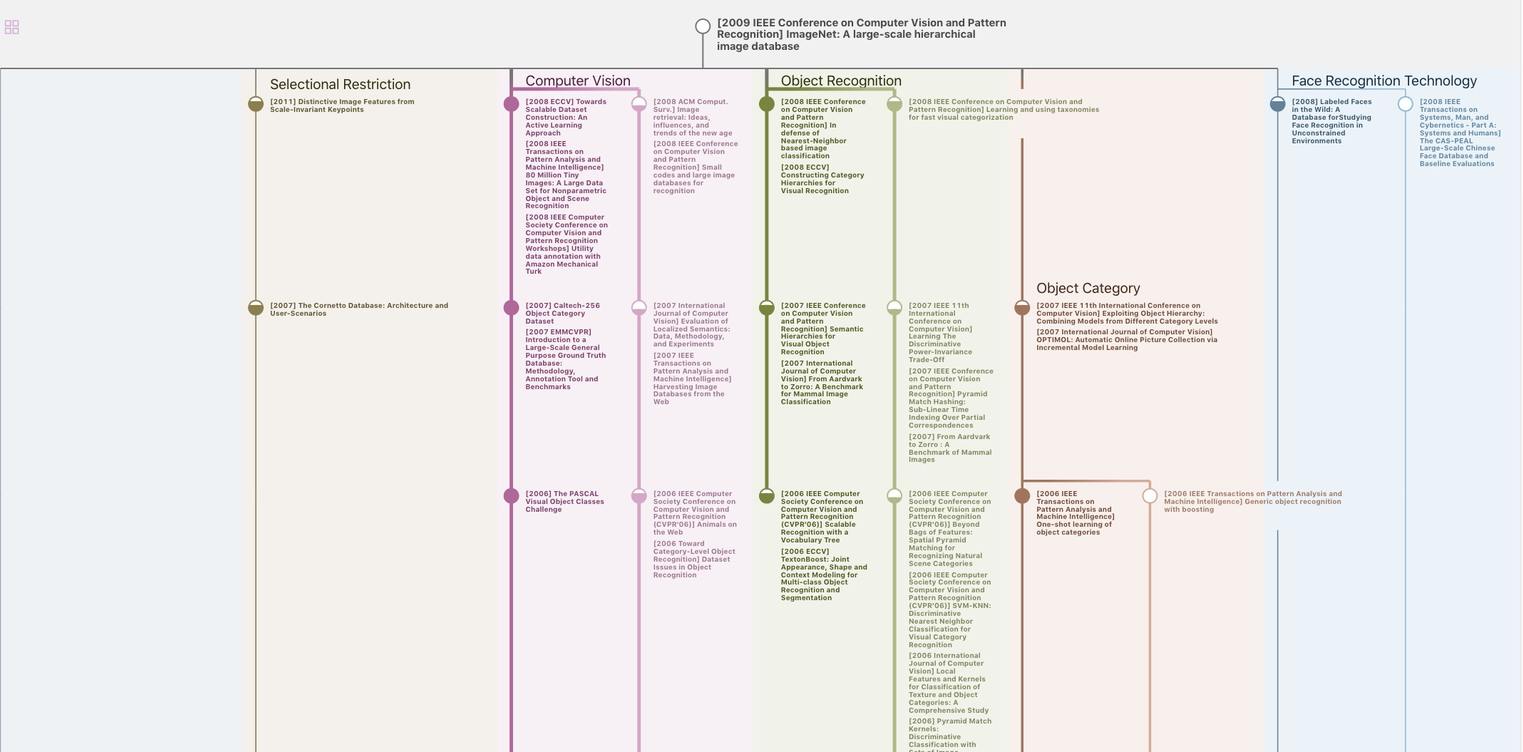
生成溯源树,研究论文发展脉络
Chat Paper
正在生成论文摘要