Exploring target specificity of antimicrobial peptides through deep learning embeddings
BCB(2021)
摘要
ABSTRACTIn the face of increasing bacterial resistance to antibiotics, antimicrobial peptides (AMPs) have stood out as an encouraging target for the development of new drugs. Machine learning approaches can be applied to this area to characterize large sets of AMPs based on their bacterial targets, activity measures, and other sequence features. Such methods enable wet-laboratory researchers to optimize the speed and accuracy of their work by focusing on prioritized candidates [5]. Prior work on computational AMP recognition has largely focused on binary sequence classification (predicting AMP vs non-AMP) but is beginning to venture into de novo peptide design [5]. This work takes steps to further understand AMP function and specificity by learning sequence embeddings based on both molecular sequence and activity measures against different bacteria targets. The model uses a Siamese network architecture [1] to learn from pairs of AMPs to predict how their activity differs against 10 different genera of bacteria. Unlike many other approaches, we also consider N- and C-termini modifications to sequences. Training and testing data originates from the Database of Antimicrobial Activity and Structure of Peptides (DBAASP) [4] and was parsed to consider monomer AMPs with activity measurements recorded as minimum inhibitory concentration (MIC). Due to the large heterogeneity of bacteria at the species-level, responses were grouped by genera and MIC values averaged. Based on the percentage of all AMPs with a mean MIC response available, the top 10 genera were considered. That data set was split into training (4, 170 AMPs), validation (1, 142 AMPs), and testing (535 AMPs) partitions. To reduce the chance of data leakage between testing and training data, the CD-HIT server [2] was used (after removing termini modifications) to ensure all testing sequences share < 90% identity with all training/validation sequences. Each partition was further arranged into pairs of sequences sharing the same target, with responses calculated as the difference in mean MIC values. The Siamese network consists of an embedding and long short-term memory layer [3] that are trained in a supervised setting. It compares AMP sequence pairs to train a shared set of weights. All input sequences are padded to be the same length and a tokenizer is used to encode both amino acids and termini modifications. The model outputs sequence embeddings based on the difference in MIC for each AMP pair. To obtain insight into AMP activity and specificity, separate models are trained for gram-positive and gram-negative genera. Trained embeddings for each model are then plotted and compared to visualize how bacterial membrane structure can influence AMP sequence composition. These results present another step towards making AMP deep learning models more informative and understandable to the research community.
更多查看译文
AI 理解论文
溯源树
样例
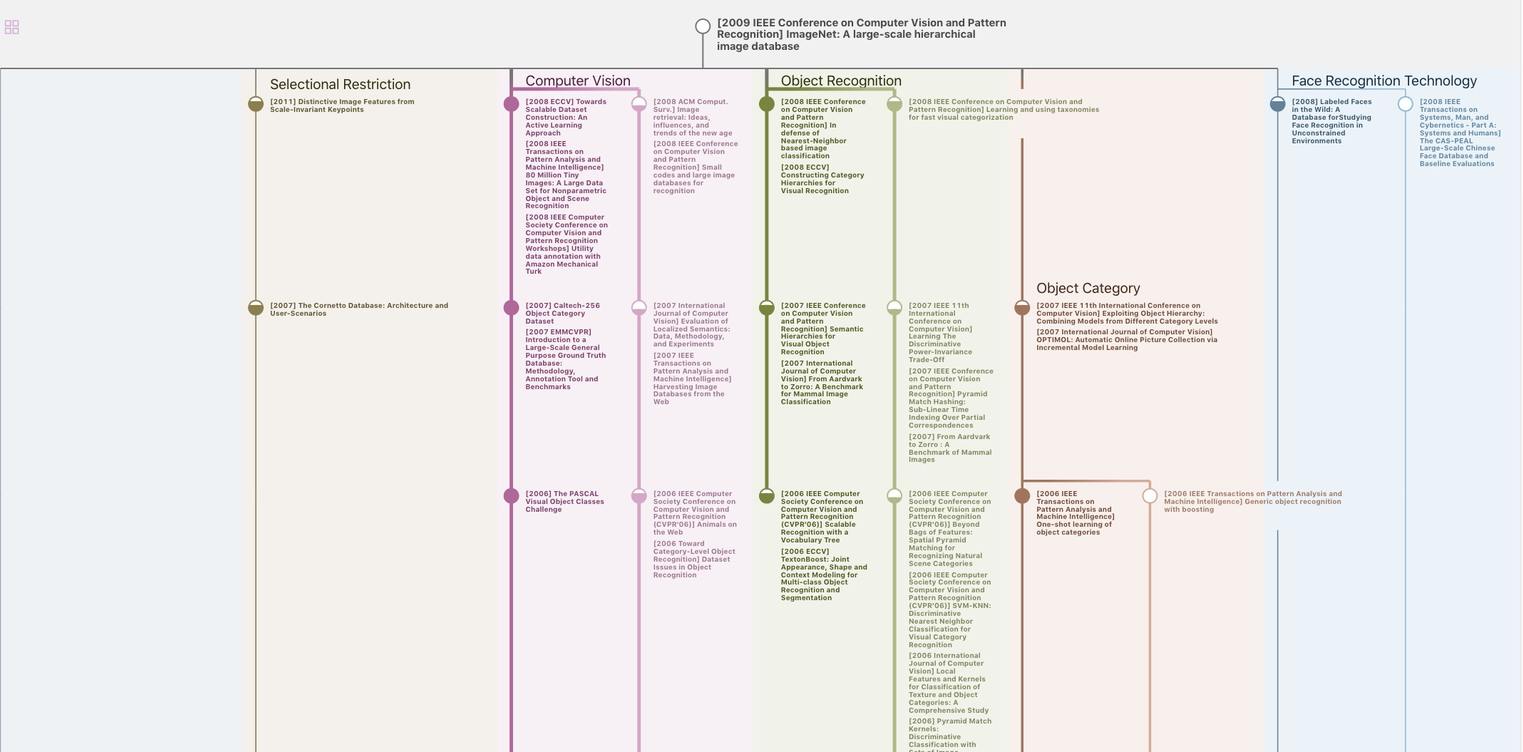
生成溯源树,研究论文发展脉络
Chat Paper
正在生成论文摘要