Predicting Intraoperative Difficulty of Open Liver Resections: The DIFF-scOR Study, An Analysis of 1393 Consecutive Hepatectomies From a French Multicenter Cohort.
Annals of surgery(2021)
摘要
OBJECTIVE:The aim of this study was to build a predictive model of operative difficulty in open liver resections (LRs).
SUMMARY BACKGROUND DATA:Recent attempts at classifying open-LR have been focused on postoperative outcomes and were based on predefined anatomical schemes without taking into account other anatomical/technical factors.
METHODS:Four intraoperative variables were perceived by the authors as to reflect operative difficulty: operation and transection times, blood loss, and number of Pringle maneuvers. A hierarchical ascendant classification (HAC) was used to identify homogeneous groups of operative difficulty, based on these variables. Predefined technical/anatomical factors were then selected to build a multivariable logistic regression model (DIFF-scOR), to predict the probability of pertaining to the highest difficulty group. Its discrimination/calibration was assessed. Missing data were handled using multiple imputation.
RESULTS:HAC identified 2 clusters of operative difficulty. In the "Difficult LR" group (20.8% of the procedures), operation time (401 min vs 243 min), transection time (150 vs.63 minute), blood loss (900 vs 400 mL), and number of Pringle maneuvers (3 vs 1) were higher than in the "Standard LR" group. Determinants of operative difficulty were body weight, number and size of nodules, biliary drainage, anatomical or combined LR, transection planes between segments 2 and 4, 4, and 8 or 7 and 8, nonanatomical resections in segments 2, 7, or 8, caval resection, bilioentric anastomosis and number of specimens. The c-statistic of the DIFF-scOR was 0.822. By contrast, the discrimination of the DIFF-scOR to predict 90-day mortality and severe morbidity was poor (c-statistic: 0.616 and 0.634, respectively).
CONCLUSION:The DIFF-scOR accurately predicts open-LR difficulty and may be used for various purposes in clinical practice and research.
更多查看译文
AI 理解论文
溯源树
样例
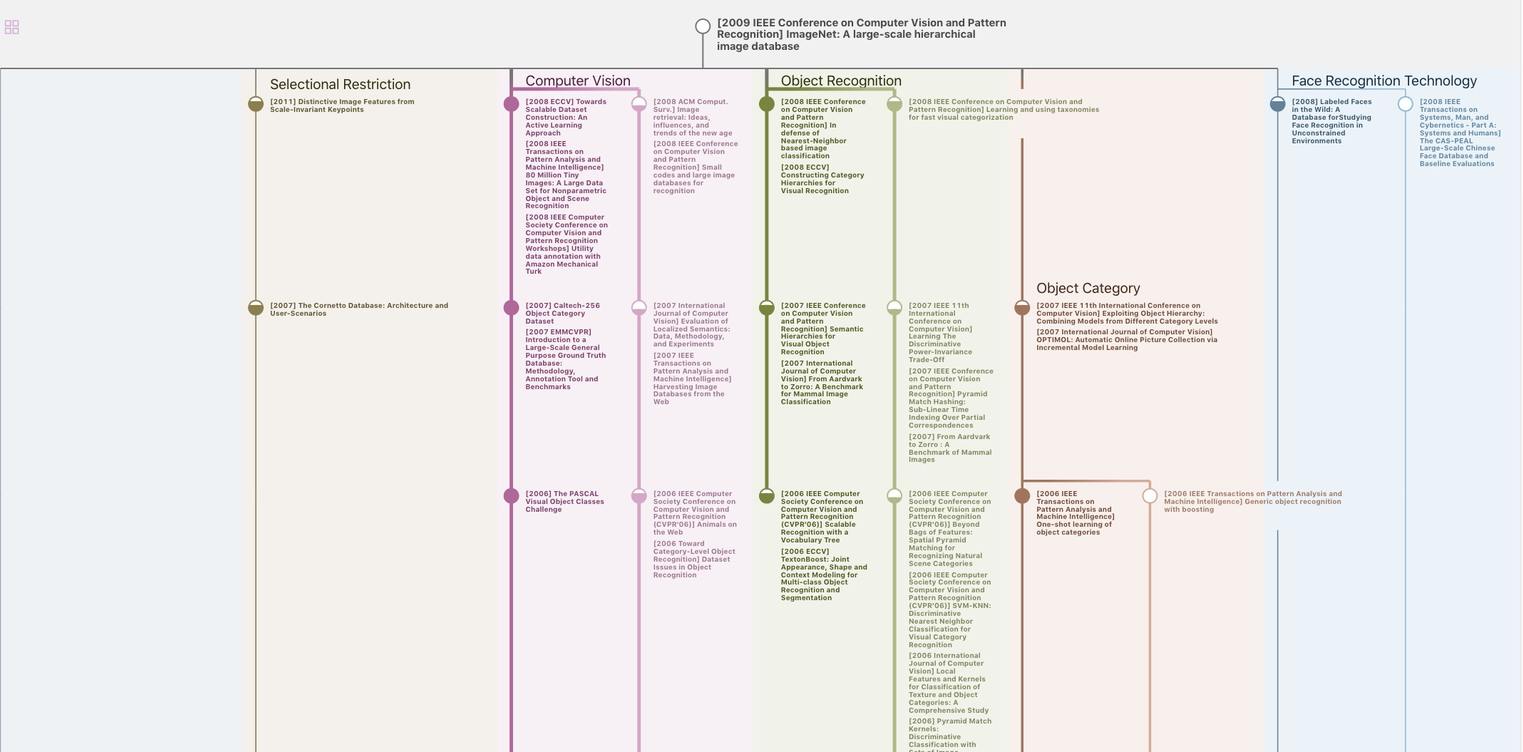
生成溯源树,研究论文发展脉络
Chat Paper
正在生成论文摘要