Whole Body Model Predictive Control with a Memory of Motion: Experiments on a Torque-Controlled Talos
2021 IEEE INTERNATIONAL CONFERENCE ON ROBOTICS AND AUTOMATION (ICRA 2021)(2021)
摘要
This paper presents the first successful experiment implementing whole-body model predictive control with state feedback on a torque-control humanoid robot. We demonstrate that our control scheme is able to do whole-body target tracking, control the balance in front of strong external perturbations and avoid collision with an external object. The key elements for this success are threefold. First, optimal control over a receding horizon is implemented with Crocoddyl, an optimal control library based on differential dynamics programming, providing state-feedback control in less than 10 ms. Second, a warm start strategy based on memory of motion has been implemented to overcome the sensitivity of the optimal control solver to initial conditions. Finally, the optimal trajectories are executed by a low-level torque controller, feedbacking on direct torque measurement at high frequency. This paper provides the details of the method, along with analytical benchmarks with the real humanoid robot Talos.A video of the experiment is available at https://peertube.laas.fr/videos/watch/cbc25927-337c-4635-a1bc-153b9aeb4135
更多查看译文
关键词
Optimal control,Humanoid robot,Model predictive control,Torque,Sensitivity (control systems),Solver,Control theory,Differential (mechanical device),State (computer science),Computer science
AI 理解论文
溯源树
样例
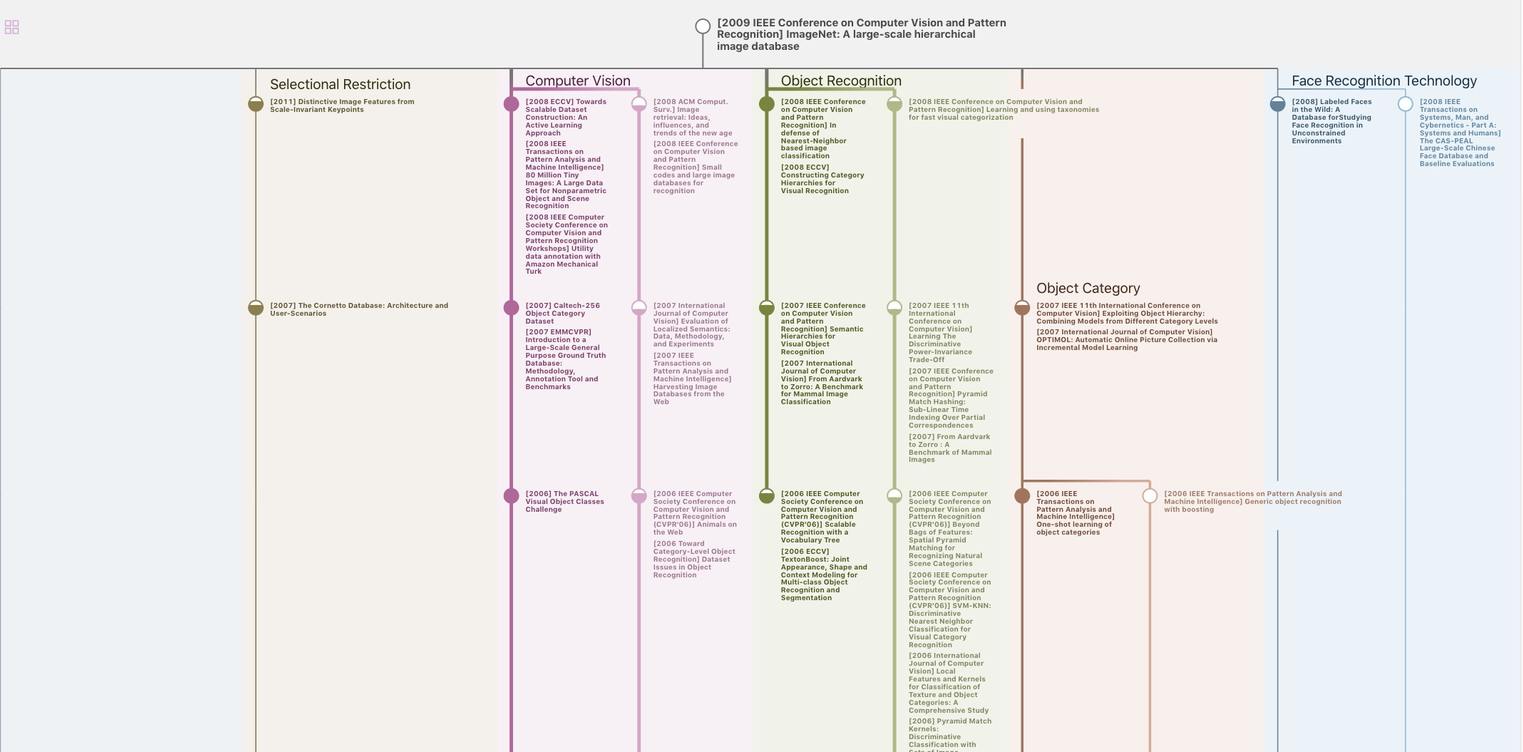
生成溯源树,研究论文发展脉络
Chat Paper
正在生成论文摘要