WeNet - Production Oriented Streaming and Non-Streaming End-to-End Speech Recognition Toolkit.
Interspeech(2021)
摘要
In this paper, we propose an open source, production first, and production ready speech recognition toolkit called WeNet in which a new two-pass approach is implemented to unify streaming and non-streaming end-to-end (E2E) speech recognition in a single model. The main motivation of WeNet is to close the gap between the research and the production of E2E speechrecognition models. WeNet provides an efficient way to ship ASR applications in several real-world scenarios, which is the main difference and advantage to other open source E2E speech recognition toolkits. In our toolkit, a new two-pass method is implemented. Our method propose a dynamic chunk-based attention strategy of the the transformer layers to allow arbitrary right context length modifies in hybrid CTC/attention architecture. The inference latency could be easily controlled by only changing the chunk size. The CTC hypotheses are then rescored by the attention decoder to get the final result. Our experiments on the AISHELL-1 dataset using WeNet show that, our model achieves 5.03\% relative character error rate (CER) reduction in non-streaming ASR compared to a standard non-streaming transformer. After model quantification, our model perform reasonable RTF and latency.
更多查看译文
关键词
Word error rate,Transformer (machine learning model),Context (language use),End-to-end principle,Speech recognition,Reduction (complexity),Latency (audio),Computer science,Inference,Production (computer science)
AI 理解论文
溯源树
样例
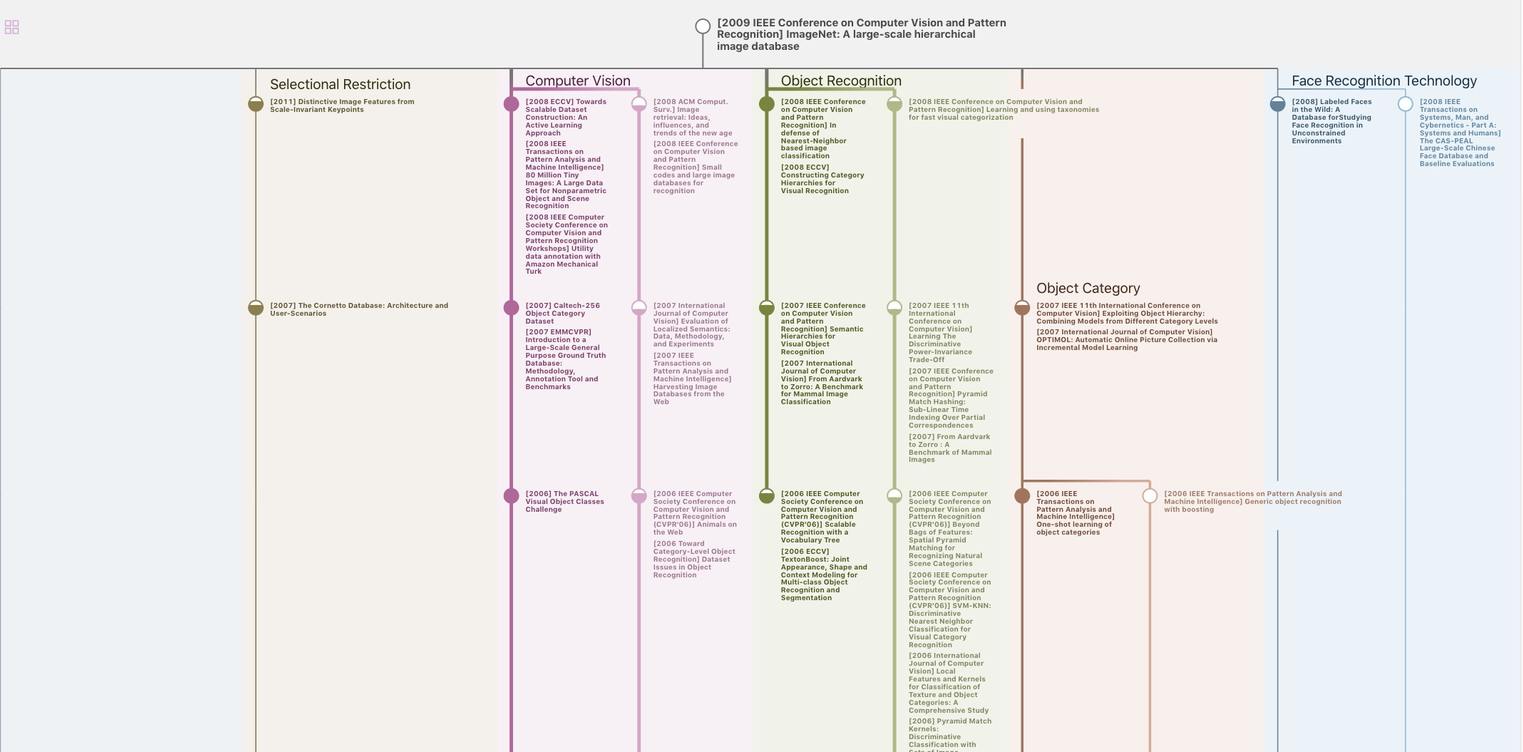
生成溯源树,研究论文发展脉络
Chat Paper
正在生成论文摘要