A lightweight multi-scale channel attention network for image super-resolution
Neurocomputing(2021)
摘要
In recent years, deep learning techniques have significantly improved the performance of single image super-resolution (SISR). However, this improvement is often achieved at the cost of introducing a large amount of parameters, which limits the real-world applications for SISR. In this paper, we propose a lightweight SISR network called Multi-scale Channel Attention Network for Image Super-Resolution (MCSN). Our contributions are threefold. First of all, the multi-scale feature fusion block (MSFFB) can extract multi-scale features by filters with different receptive fields. Secondly, the channel shuffle attention mechanism (CSAM) encourages the flow of the information across feature channels and enhances the ability of feature selection. Thirdly, the global feature fusion connection (GFFC) can effectively improve feature utilization. Extensive experiments demonstrate that the parameter amount of our method is reduced by 3/4 compared with the current state-of-the-art MSRN method, while both the subjective visual effect and objective quality of the reconstructed high-resolution images are significantly better.
更多查看译文
关键词
Super-resolution,Attention mechanism,Multi-scale features,Deep learning
AI 理解论文
溯源树
样例
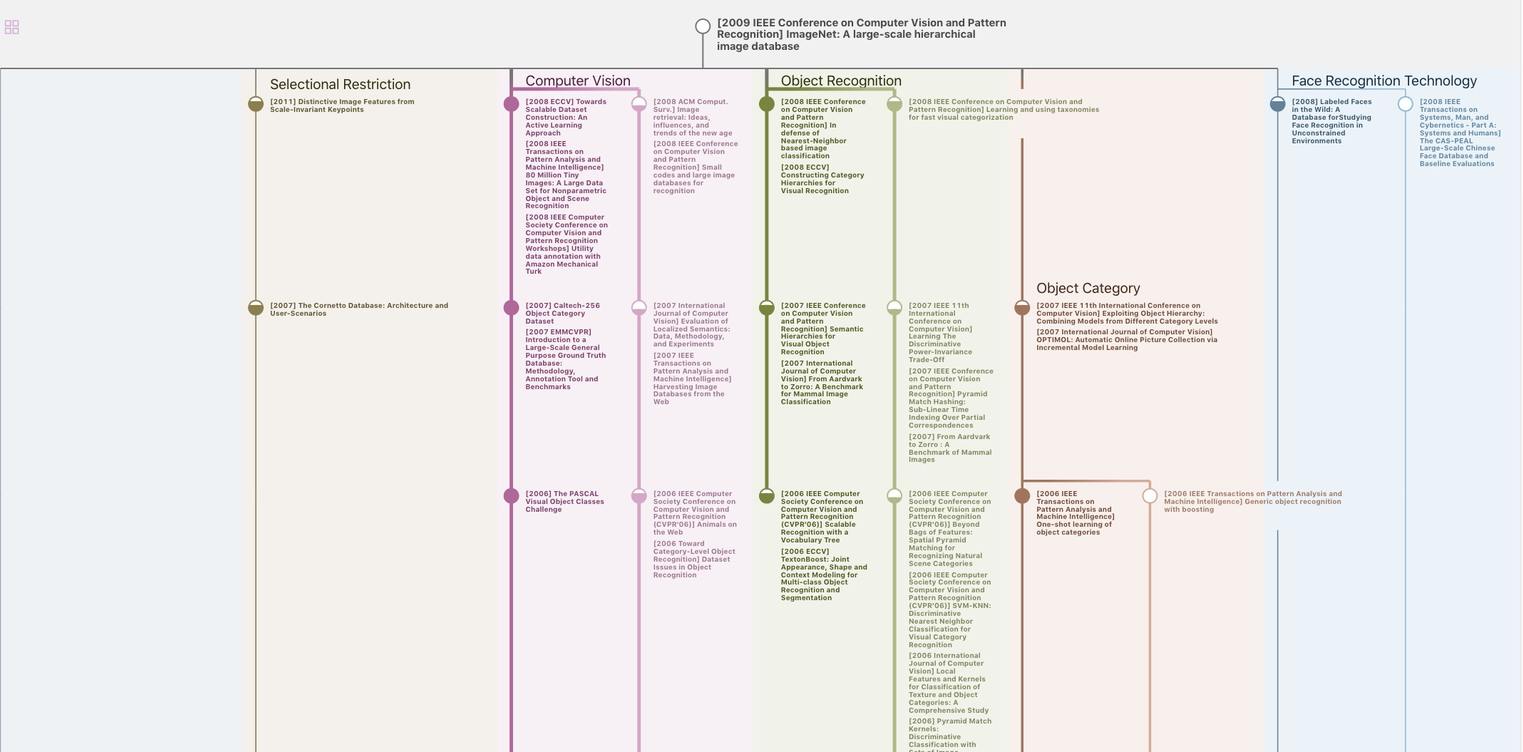
生成溯源树,研究论文发展脉络
Chat Paper
正在生成论文摘要