IMITA: Imitation Learning for Generalizing Cloud Orchestration
2021 IEEE/ACM 21st International Symposium on Cluster, Cloud and Internet Computing (CCGrid)(2021)
摘要
Operating large scale and feature-rich applications is becoming increasingly complex as engineers need to deploy highly configurable software releases on distributed cloud stacks while managing ever-shorter production cycles. Although recent proposals attempt to streamline cloud resources orchestration, there is still a significant challenge in making such solutions generalize to unseen cloud stacks. In other words, the behavior of application-specific Key Performance Indicators (KPIs) and resource configurations, crafted for specific stacks, may differ on heterogeneous deployments, requiring time-consuming policy adjustments. We introduce IMITA, a system that leverages imitation learning to create models by imitating an expert behavior that can be generalized seamlessly to new cloud stacks. To make a generalized model, IMITA maps expert actions taken based on the application KPI space to the space of resource utilization metrics that are universally available in cloud platforms. This mapping enables the model to trigger actions, mimicking expert behavior, upon the occurrence of similar re-source utilization footprints across deployments. We demonstrate IMITA by learning to scale-out Cassandra deployments with diverse configurations and workloads. Our results show IMITA can replicate expert actions across deployments and extrapolate to unseen environments by achieving 50 - 94% fewer false positives actions than traditional threshold-based policies while still adhering to Service-Level Objectives (SLO) and avoiding under-provisioning of resources. Moreover, since collecting data in clouds is costly, IMITA gathers data only for representative configurations to train the imitator model. This approach reduces the size of the collected data to 50%.
更多查看译文
关键词
Cloud Orchestration,Imitation Learning,Generalization
AI 理解论文
溯源树
样例
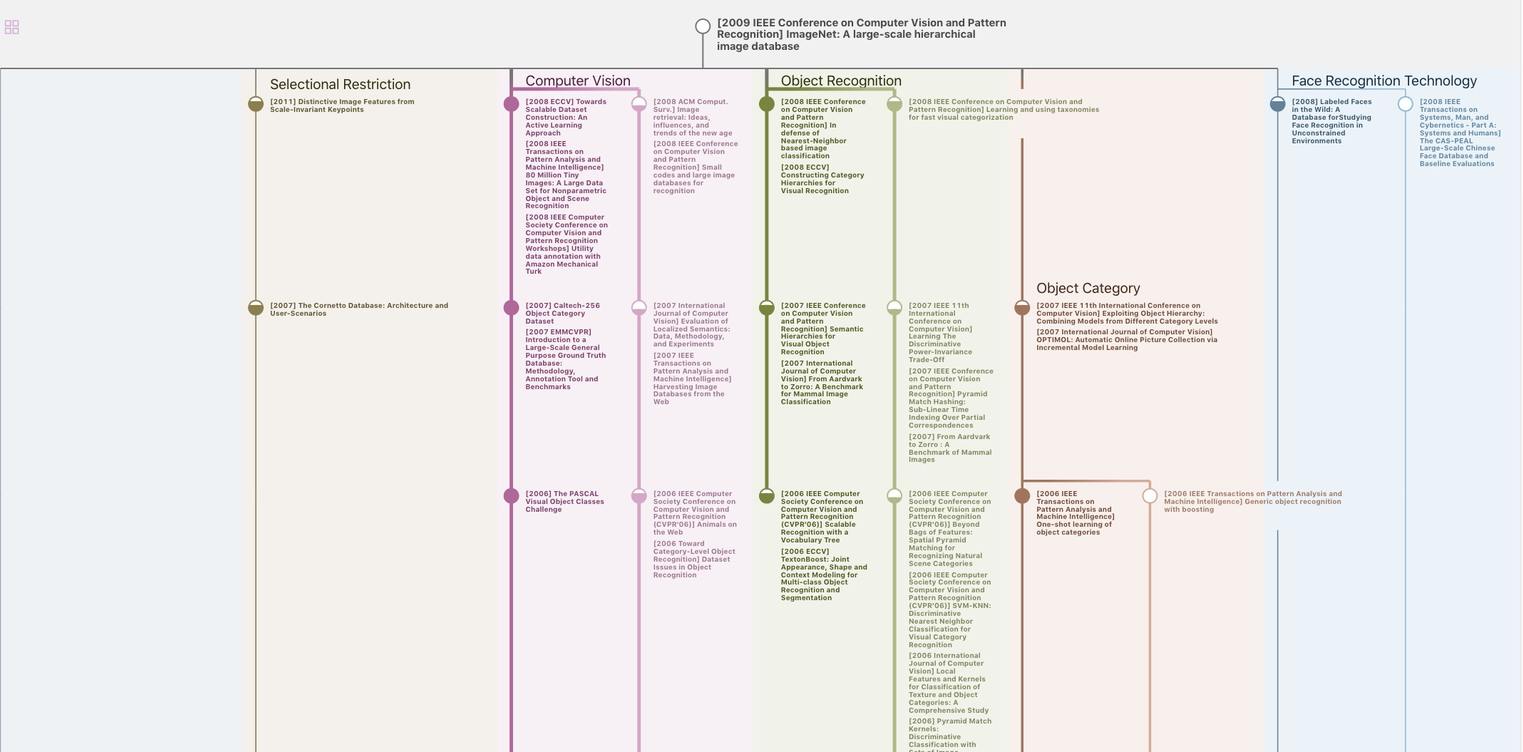
生成溯源树,研究论文发展脉络
Chat Paper
正在生成论文摘要