SARA: Scaling a Reconfigurable Dataflow Accelerator
2021 ACM/IEEE 48th Annual International Symposium on Computer Architecture (ISCA)(2021)
摘要
The need for speed in modern data-intensive work-loads and the rise of "dark silicon" in the semiconductor industry are pushing for larger, faster, and more energy and area-efficient architectures, such as Reconfigurable Dataflow Accelerators (RDAs). Nevertheless, challenges remain in developing mechanisms to effectively utilize the compute power of these large-scale RDAs. To address these challenges, we present SARA, a compiler that employs a novel mapping strategy to efficiently utilize large-scale RDAs. Starting from a single-threaded imperative abstraction, SARA spatially maps a program onto RDA's distributed resources, exploiting dataflow parallelism within and across hyperblocks to saturate the compute throughput of an RDA. SARA introduces (a) compiler-managed memory consistency (CMMC), a control paradigm that hierarchically pipelines a nested and data-dependent control-flow graph onto a dataflow architecture, and (b) a compilation flow that decomposes the program graph across distributed heterogeneous resources to hide low-level RDA constraints from programmers. Our evaluation shows that SARA achieves close to perfect performance scaling on a recently proposed RDA—Plasticine. Over a mix of deep-learning, graph-processing, and streaming applications, SARA achieves a 1.9× geo-mean speedup over a Tesla V100 GPU using only 12% of the silicon area.
更多查看译文
关键词
RDA,CGRA,Plasticine,Scalability,Domain-Specific Compiler
AI 理解论文
溯源树
样例
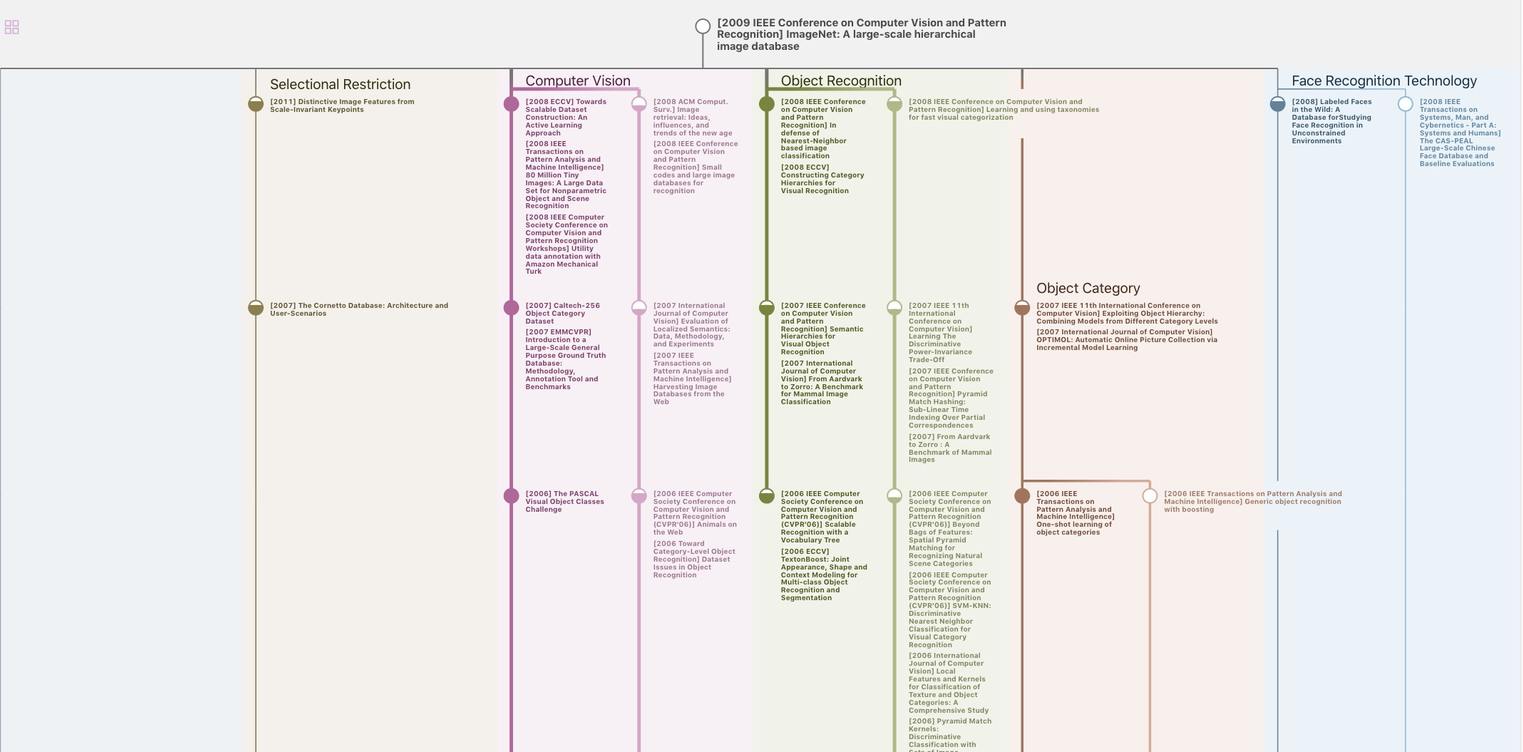
生成溯源树,研究论文发展脉络
Chat Paper
正在生成论文摘要