Robustness via Probabilistic Cross-Task Ensembles
user-60ab1d9b4c775e04970067d6(2021)
摘要
We present a method for making predictions using neural networks that, at the test time, is robust against shifts from the training data distribution. The proposed method is based on making \emph{one prediction via different cues} (called middle domains) and ensembling their outputs into one strong prediction. The premise of the idea is that predictions via different cues respond differently to distribution shifts, hence one can merge them into one robust final prediction, if ensembling can be done successfully. We perform the ensembling in a straightforward but principled probabilistic manner. The evaluations are performed using multiple vision dataset under a range of natural and synthetic distribution shifts which demonstrate the proposed method is considerably more robust compared to its standard learning counterpart, conventional ensembles, and several other baselines.
更多查看译文
关键词
Robustness (computer science),Probabilistic logic,Artificial neural network,Pattern recognition,Range (statistics),Merge (linguistics),Computer science,Task (project management),Artificial intelligence,Training set,Uncertainty estimation
AI 理解论文
溯源树
样例
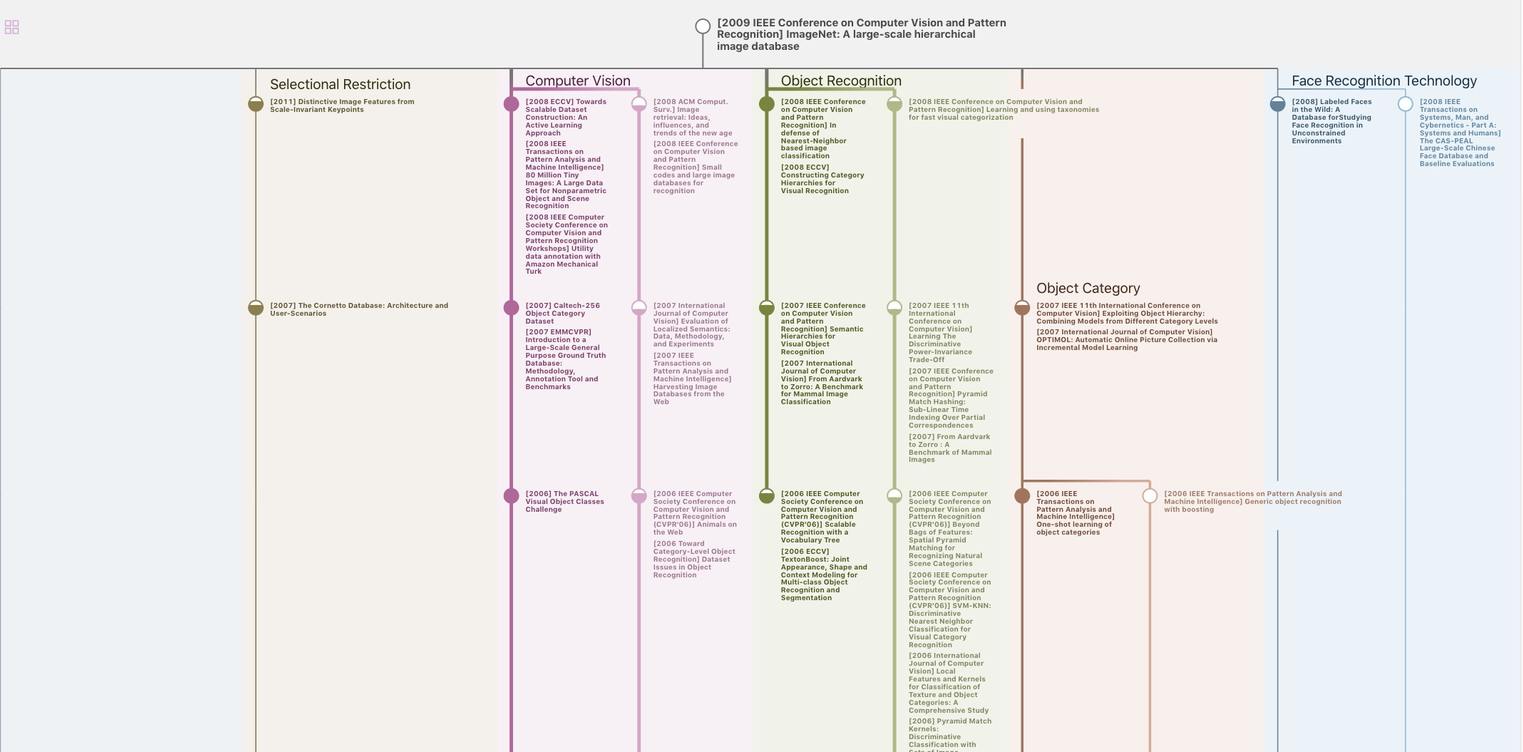
生成溯源树,研究论文发展脉络
Chat Paper
正在生成论文摘要