Bayesian deep learning for partial differential equation parameter discovery with sparse and noisy data
Journal of Computational Physics: X(2022)
摘要
Scientific machine learning has been successfully applied to inverse problems and PDE discovery in computational physics. One caveat concerning current methods is the need for large amounts of (“clean”) data, in order to characterize the full system response and discover underlying physical models. Bayesian methods may be particularly promising for overcoming these challenges, as they are naturally less sensitive to the negative effects of sparse and noisy data. In this paper, we propose to use Bayesian neural networks (BNN) in order to: 1) Recover the full system states from measurement data (e.g. temperature, velocity field, etc.). We use Hamiltonian Monte-Carlo to sample the posterior distribution of a deep and dense BNN, and show that it is possible to accurately capture physics of varying complexity, without overfitting. 2) Recover the parameters instantiating the underlying partial differential equation (PDE) governing the physical system. Using the trained BNN, as a surrogate of the system response, we generate datasets of derivatives that are potentially comprising the latent PDE governing the observed system and then perform a sequential threshold Bayesian linear regression (STBLR), between the successive derivatives in space and time, to recover the original PDE parameters. We take advantage of the confidence intervals within the BNN outputs, and introduce the spatial derivatives cumulative variance into the STBLR likelihood, to mitigate the influence of highly uncertain derivative data points; thus allowing for more accurate parameter discovery. We demonstrate our approach on a handful of example, in applied physics and non-linear dynamics.
更多查看译文
关键词
Scientific machine learning,SciML,Bayesian inference,Neural network,Partial differential equation,Inverse problems
AI 理解论文
溯源树
样例
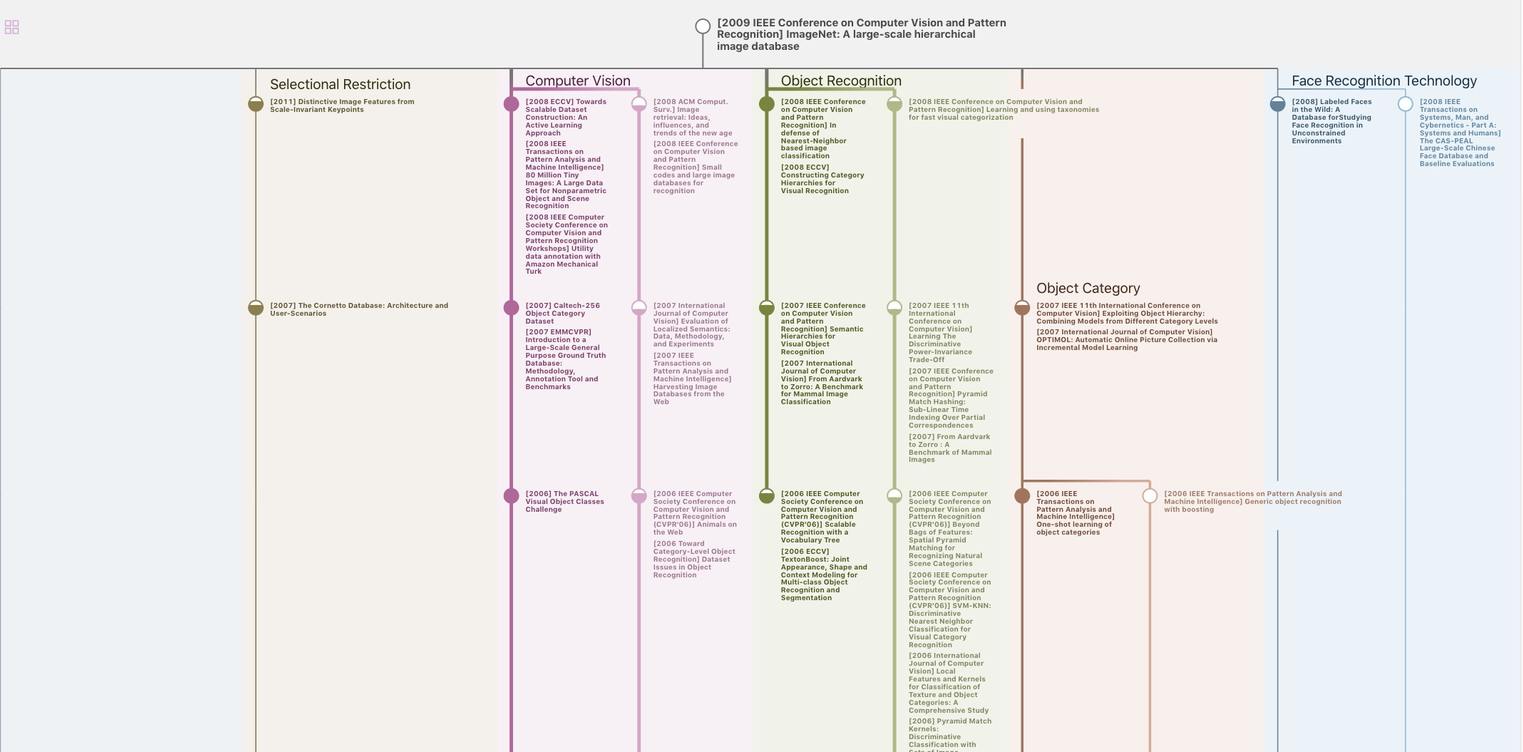
生成溯源树,研究论文发展脉络
Chat Paper
正在生成论文摘要