Improving Label Noise Robustness With Data Augmentation And Semi-Supervised Learning (Student Abstract)
THIRTY-FIFTH AAAI CONFERENCE ON ARTIFICIAL INTELLIGENCE, THIRTY-THIRD CONFERENCE ON INNOVATIVE APPLICATIONS OF ARTIFICIAL INTELLIGENCE AND THE ELEVENTH SYMPOSIUM ON EDUCATIONAL ADVANCES IN ARTIFICIAL INTELLIGENCE(2021)
摘要
Modern machine learning algorithms typically require large amounts of labeled training data to fit a reliable model. To minimize the cost of data collection, researchers often employ techniques such as crowdsourcing and web scraping. However, web data and human annotations are known to exhibit high margins of error, resulting in sizable amounts of incorrect labels. Poorly labeled training data can cause models to overfit to the noise distribution, crippling performance in real-world applications. In this work, we investigate the viability of using data augmentation in conjunction with semi supervised learning to improve the label noise robustness of image classification models. We conduct several experiments using noisy variants of the CIFAR-10 image classification dataset to benchmark our method against existing algorithms. Experimental results show that our augmentative SSL approach improves upon the state-of-the-art.
更多查看译文
关键词
label noise robustness,data augmentation,learning,semi-supervised
AI 理解论文
溯源树
样例
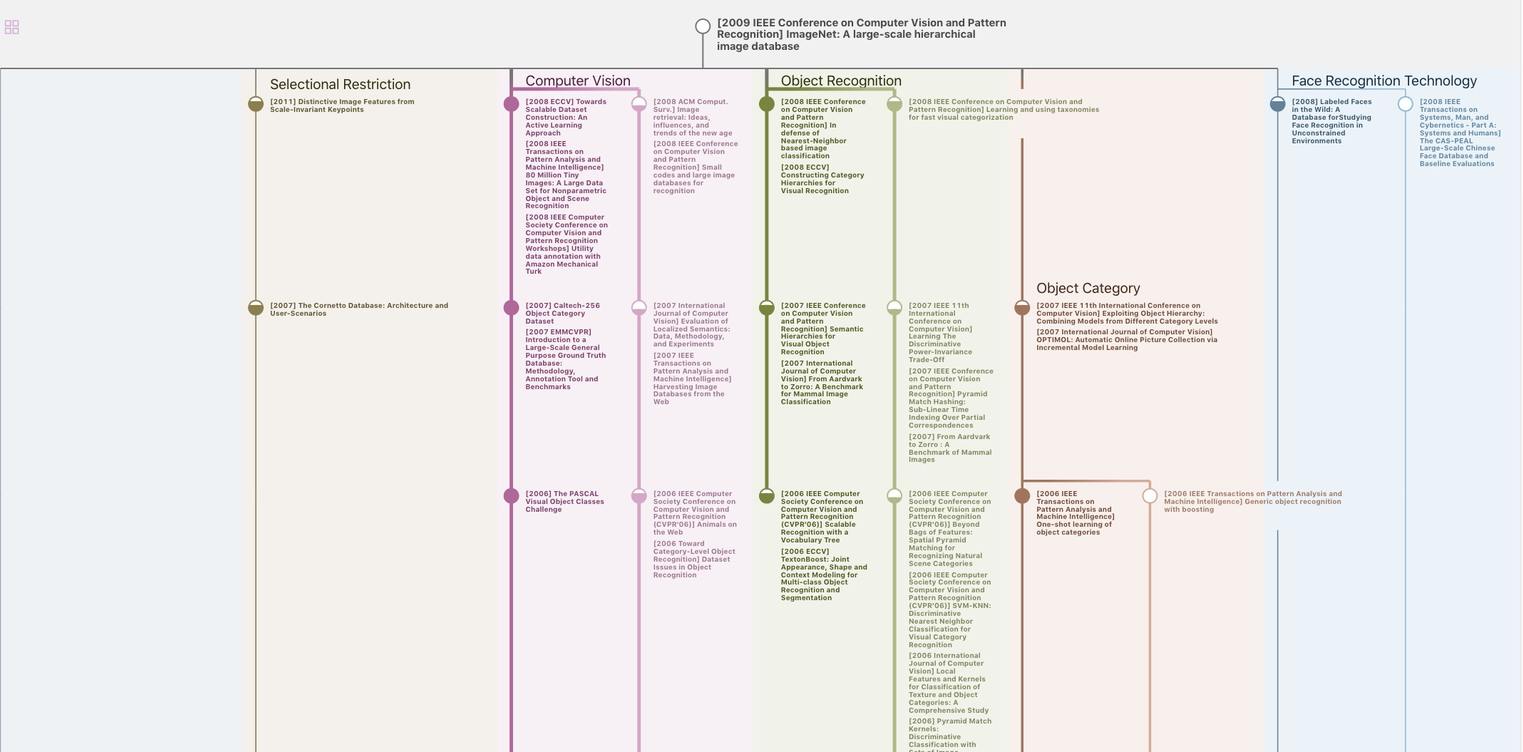
生成溯源树,研究论文发展脉络
Chat Paper
正在生成论文摘要