Tensor Principal Component Analysis in High Dimensional CP Models
IEEE Transactions on Information Theory(2023)
摘要
The CP decomposition for high dimensional non-orthogonal spiked tensors is an important problem with broad applications across many disciplines. However, previous works with theoretical guarantee typically assume restrictive incoherence conditions on the basis vectors for the CP components. In this paper, we propose new computationally efficient composite PCA and concurrent orthogonalization algorithms for tensor CP decomposition with theoretical guarantees under mild incoherence conditions. The composite PCA applies the principal component or singular value decompositions twice, first to a matrix unfolding of the tensor data to obtain singular vectors and then to the matrix folding of the singular vectors obtained in the first step. It can be used as an initialization for any iterative optimization schemes for the tensor CP decomposition. The concurrent orthogonalization algorithm iteratively estimates the basis vector in each mode of the tensor by simultaneously applying projections to the orthogonal complements of the spaces generated by other CP components in other modes. It is designed to improve the alternating least squares estimator and other forms of the high order orthogonal iteration for tensors with low or moderately high CP ranks, and it is guaranteed to have second or higher order convergence when the error of any given initial estimator is bounded by a small constant. Our theoretical investigation provides estimation accuracy and convergence rates for the two proposed algorithms. Both proposed algorithms are applicable to deterministic tensor, its noisy version, and the order-
$2K$
covariance tensor of order-
$K$
tensor data in a factor model with uncorrelated factors. Simulation experiments demonstrate significant practical superiority of our approach over existing methods.
更多查看译文
关键词
Tensor principal component analysis,PCA,CP decomposition,spiked covariance,dimension reduction,unfolding,orthogonal projection
AI 理解论文
溯源树
样例
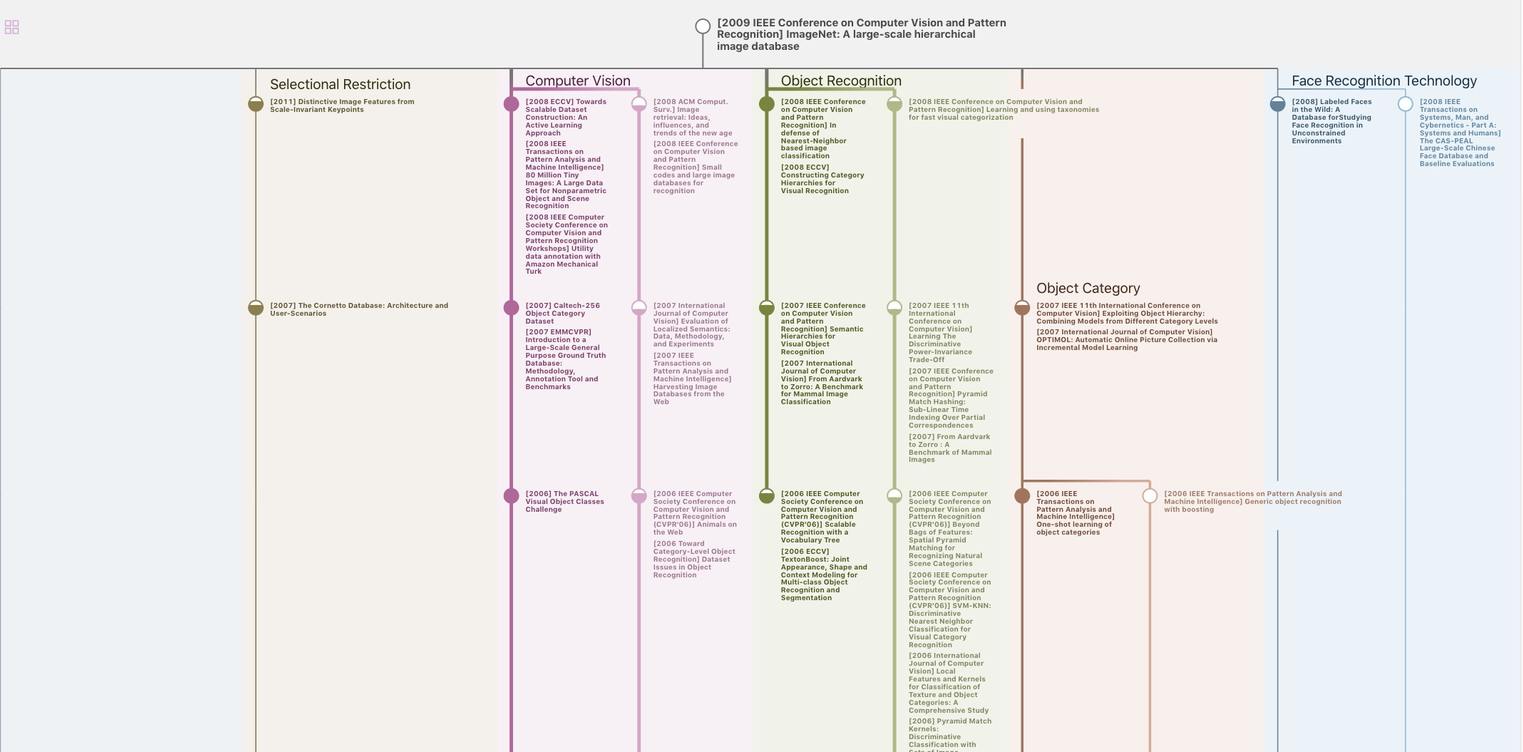
生成溯源树,研究论文发展脉络
Chat Paper
正在生成论文摘要