Local anatomy for personalised privacy protection.
Int. J. Inf. Comput. Secur.(2021)
摘要
Anonymisation technique has been extensively studied and widely applied for privacy-preserving data publishing. However, most existing methods ignore personal anonymity requirements. In these approaches, the microdata consist of three categories of attribute: explicit-identifier, quasi-identifier and sensitive attribute. In fact, the data sensitivity should be determined by individuals. An attribute is semi-sensitive if it contains both QI and sensitive values. In this paper, we propose a novel anonymisation approach, called local anatomy, to address personalised privacy protection. Local anatomy partitions the tuples who consider the value as sensitive into buckets inside each attribute. We conduct some experiments to illustrate that local anatomy can protect all the sensitive values and preserve great information utility. Additionally, we also present the concept of intelligent anonymisation system as our direction of future work.
更多查看译文
关键词
data publishing,personalised privacy protection,semi-sensitive attribute
AI 理解论文
溯源树
样例
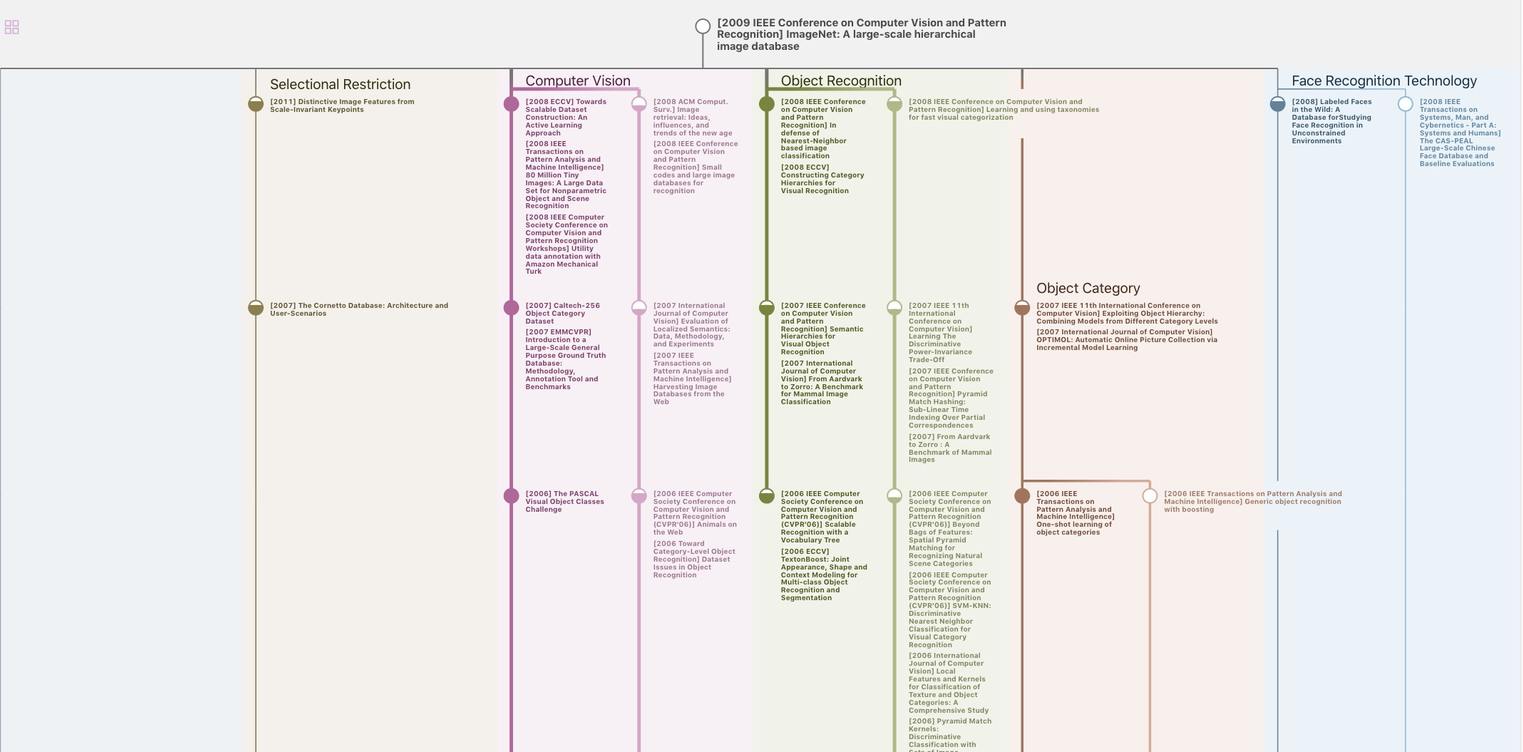
生成溯源树,研究论文发展脉络
Chat Paper
正在生成论文摘要