Re-envisioning Space-Air-Ground Integrated Networks: Reinforcement Learning for Link Optimization
IEEE INTERNATIONAL CONFERENCE ON COMMUNICATIONS (ICC 2021)(2021)
摘要
To provide ubiquitous connectivity and achieve high reliability in the under-served and under-connected areas, the integration of aerial and space communication infrastructures into terrestrial networks is envisioned as a promising solution. In this regard, unmanned aerial vehicles (UAVs), in the role of aerial base stations (BSs), have been recommended to solve the coverage problem. In order to effectively leverage the advantages of UAVs deployment, UAV trajectory and resource management are required to effectively adapt to the network conditions. However, providing backhaul connectivity for terrestrial and aerial BSs deployed in these areas is another tremendous challenge. In this paper, we aim at jointly optimizing backhaul link and access link in a space-air-ground integrated network. We consider low Earth orbit (LEO) satellites as an effective backhaul solution. For access links, we manage the radio resource among UAVs and small cell BSs, and optimize the trajectories of UAVs in the network. To solve the problem in a distributed manner, we utilize the tools of reinforcement learning, and propose two approaches based on the multi-armed bandit and satisfaction algorithms. Simulation results show that the proposed approaches yield significant performance compared to the benchmark algorithms.
更多查看译文
关键词
Low Earth Orbit Satellites, Reinforcement Learning, Resource Management, Small-cell Base Stations, Unmanned Aerial Vehicles
AI 理解论文
溯源树
样例
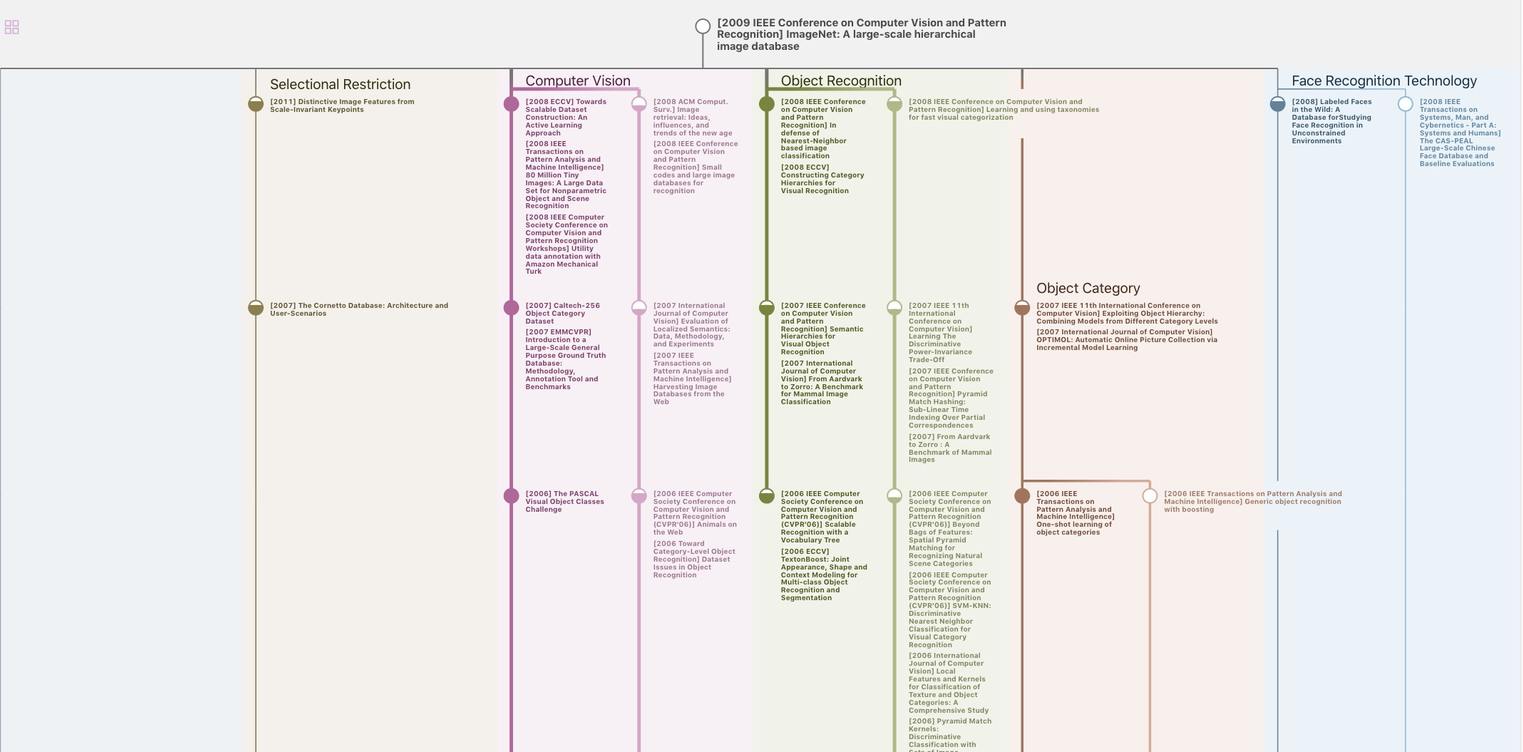
生成溯源树,研究论文发展脉络
Chat Paper
正在生成论文摘要