Learning Bias-Invariant Representation by Cross-Sample Mutual Information Minimization.
ICCV(2021)
摘要
Deep learning algorithms mine knowledge from the training data and thus would likely inherit the dataset's bias information. As a result, the obtained model would generalize poorly and even mislead the decision process in real-life applications. We propose to remove the bias information misused by the target task with a cross-sample adversarial debiasing (CSAD) method. CSAD explicitly extracts target and bias features disentangled from the latent representation generated by a feature extractor and then learns to discover and remove the correlation between the target and bias features. The correlation measurement plays a critical role in adversarial debiasing and is conducted by a cross-sample neural mutual information estimator. Moreover, we propose joint content and local structural representation learning to boost mutual information estimation for better performance. We conduct thorough experiments on publicly available datasets to validate the advantages of the proposed method over state-of-the-art approaches.
更多查看译文
关键词
learning,representation,information,mutual
AI 理解论文
溯源树
样例
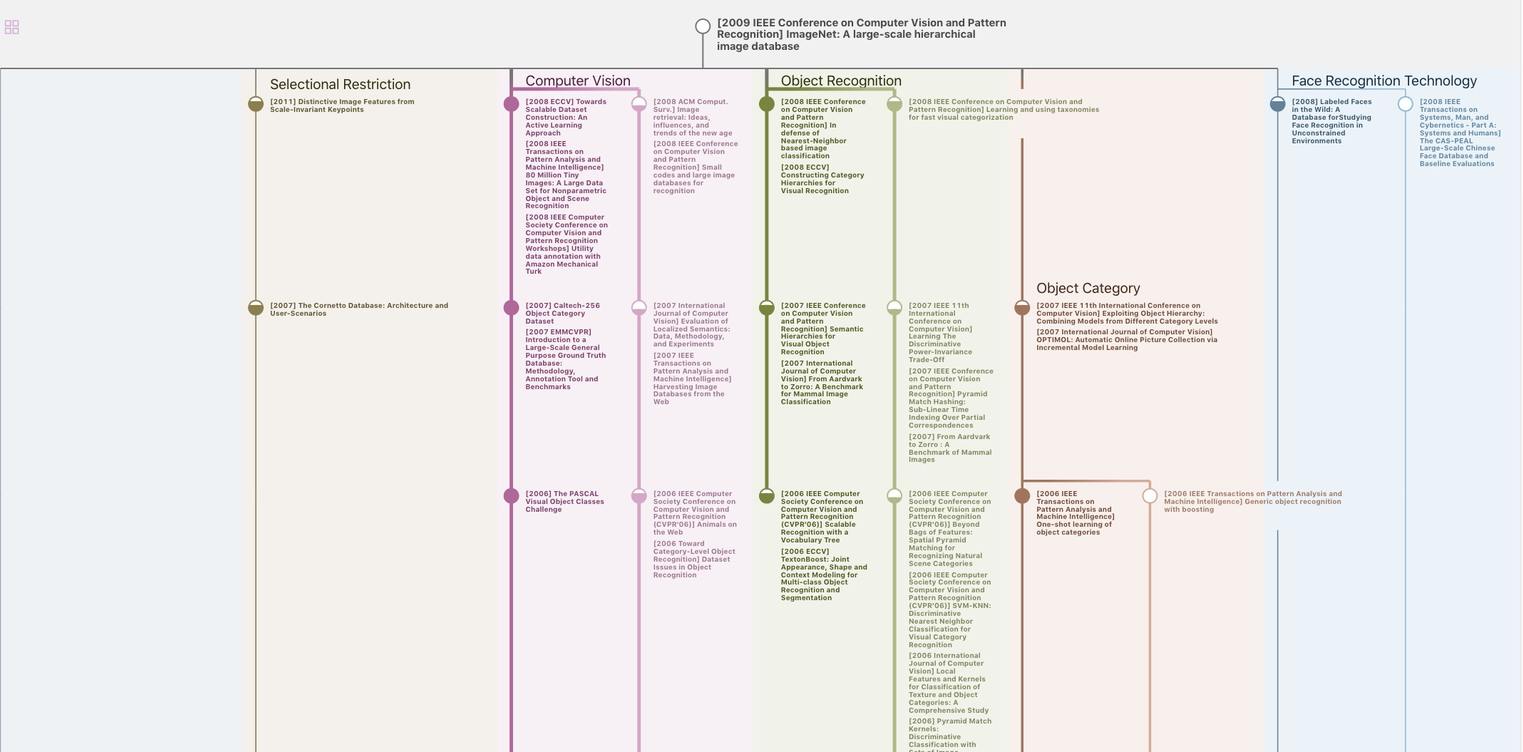
生成溯源树,研究论文发展脉络
Chat Paper
正在生成论文摘要