Syntax Matters! Syntax-Controlled in Text Style Transfer
Proceedings of the Conference Recent Advances in Natural Language Processing - Deep Learning for Natural Language Processing Methods and Applications(2021)
摘要
Existing text style transfer (TST) methods rely on style classifiers to disentangle the text's content and style attributes for text style transfer.While the style classifier plays a critical role in existing TST methods, there is no known investigation on its effect on the TST methods.In this paper, we conduct an empirical study on the limitations of the style classifiers used in existing TST methods.We demonstrate that the existing style classifiers cannot learn sentence syntax effectively and ultimately worsen existing TST models' performance.To address this issue, we propose a novel Syntax-Aware Controllable Generation (SACG) model, which includes a syntaxaware style classifier that ensures learned style latent representations effectively capture the syntax information for TST.Through extensive experiments on two popular TST tasks, we show that our proposed method significantly outperforms the state-of-the-art methods.Our case studies have also demonstrated SACG's ability to generate fluent target-style sentences that preserved the original content.
更多查看译文
关键词
Syntax-based Translation Models,Language Modeling,Topic Modeling
AI 理解论文
溯源树
样例
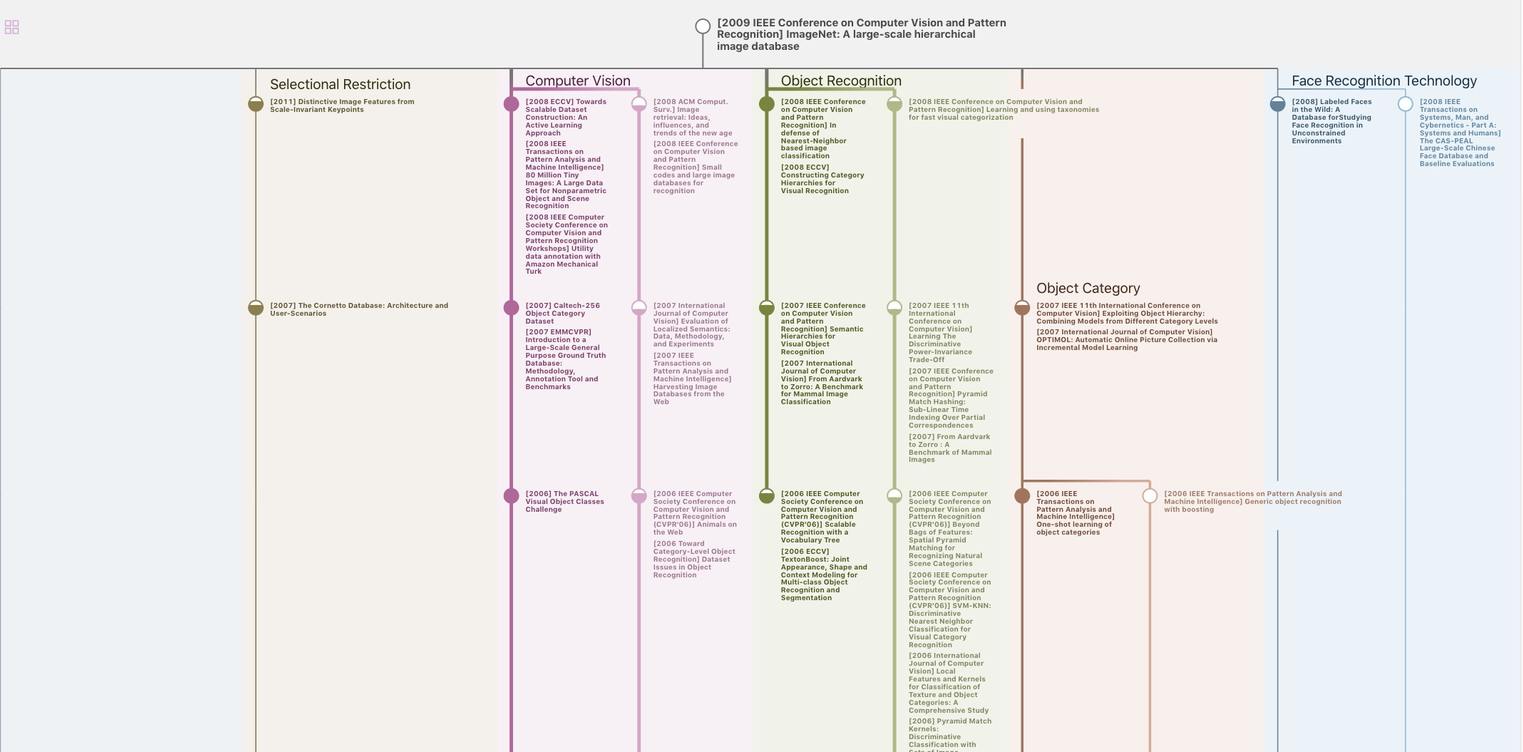
生成溯源树,研究论文发展脉络
Chat Paper
正在生成论文摘要