InsideOut: Model to Predict Outside CO Concentrations from Mobile CO Dosimeter Measurements Inside Vehicles
PROCEEDINGS OF THE 17TH EAI INTERNATIONAL CONFERENCE ON MOBILE AND UBIQUITOUS SYSTEMS: COMPUTING, NETWORKING AND SERVICES (MOBIQUITOUS 2020)(2020)
摘要
The current pollution measurement methodology is coarse-grained where the pollution measurements are spatiotemporally few and far in-between. Our vision is to provide broadly accessible, fine-grained pollution information to a variety of end-users, and in turn, allow them to make better informed decisions using a new, more accurate information stream. To this end, this study proposes a new neural network model to estimate Carbon Monoxide (CO) concentrations outside vehicle from crowd-sourced CO measurements inside vehicles measured using mobile devices (dosimeters). End-users can benefit from the fine-grained pollution information generated by this prediction model along with data from direct measurements. A neural network is used to model the dynamic relationship between the CO measurements inside and outside a moving vehicle. The resulting neural network model is then used to predict outside CO concentrations from CO measurements inside vehicles. Mobile CO dosimeters were used inside and outside vehicles to collect measurements used in training a neural network based regression model. For this regression task, a new neural network architecture was designed using Convolutional layers and Gated Recurrent Unit (GRU) layers. The results show that outside CO concentrations can be estimated from inside vehicle CO measurements with high accuracy. The proposed neural network model provides a promising new and novel source of fine-grained pollution information along with direct measurement streams.
更多查看译文
关键词
Mobile Pollution Sensing, Crowd-sourced Data Collection, Neural Networks, Internet of Things
AI 理解论文
溯源树
样例
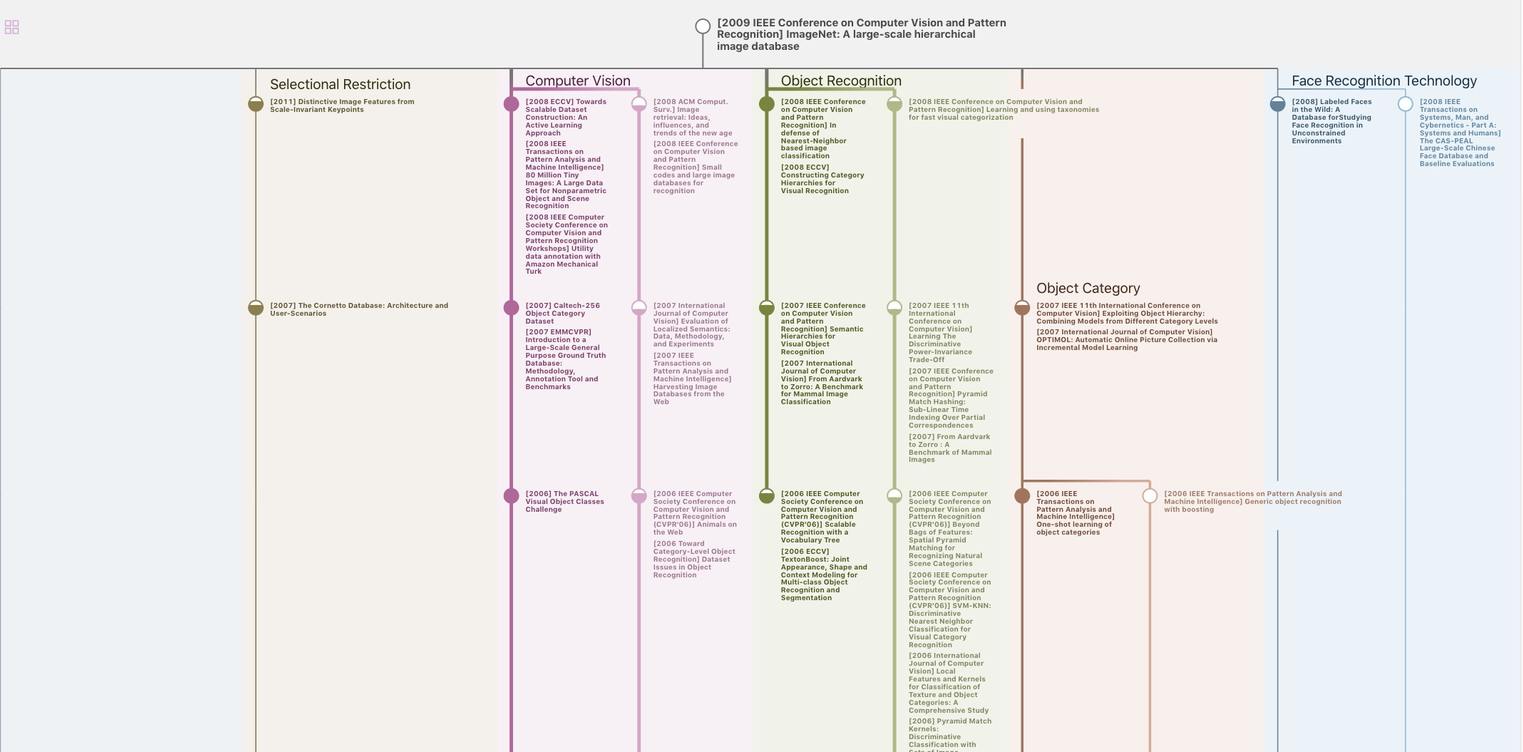
生成溯源树,研究论文发展脉络
Chat Paper
正在生成论文摘要