Blood glucose prediction using a two phase tsk fuzzy rule based system
2021 IEEE Congress on Evolutionary Computation (CEC)(2022)
摘要
Blood glucose management is a difficult task that people with diabetes usually have to perform by themselves. An accurate and timely prediction is vital in order to take decisions and recommend corrective actions to the patient when the future blood glucose value lies outside of a target range. It is crucial to predict events like hyperglycemia and hypoglycemia that may cause health damages in the short term and potential permanent damages in the long term. The aim of this paper is to describe our research on predicting blood glucose values using a two phase Takagi-Sugeno-Kang Fuzzy Rule Based System. The first phase is a learning process where membership functions and rules are optimised by a genetic algorithm. In the second phase of tuning, we used a genetic algorithm to perform the selection and optimisation of the rules. To train our model we used two different scenarios, What-if and Agnostic; in both of them the inputs are values measured by a continuous monitoring glucose system as well as previous carbohydrate intake and insulin injections. In the What-if scenario, assumed future values of meals and insulin injections are permitted. On the other hand, in the Agnostic scenario, only information of the past and present events are available for the prediction. We trained and tested our system with real data collected from 10 different diabetic patients, producing 30, 60, 90 and 120 minutes predictions with encouraging accuracy results.
更多查看译文
关键词
Diabetes,Glucose prediction,Fuzzy Rule System
AI 理解论文
溯源树
样例
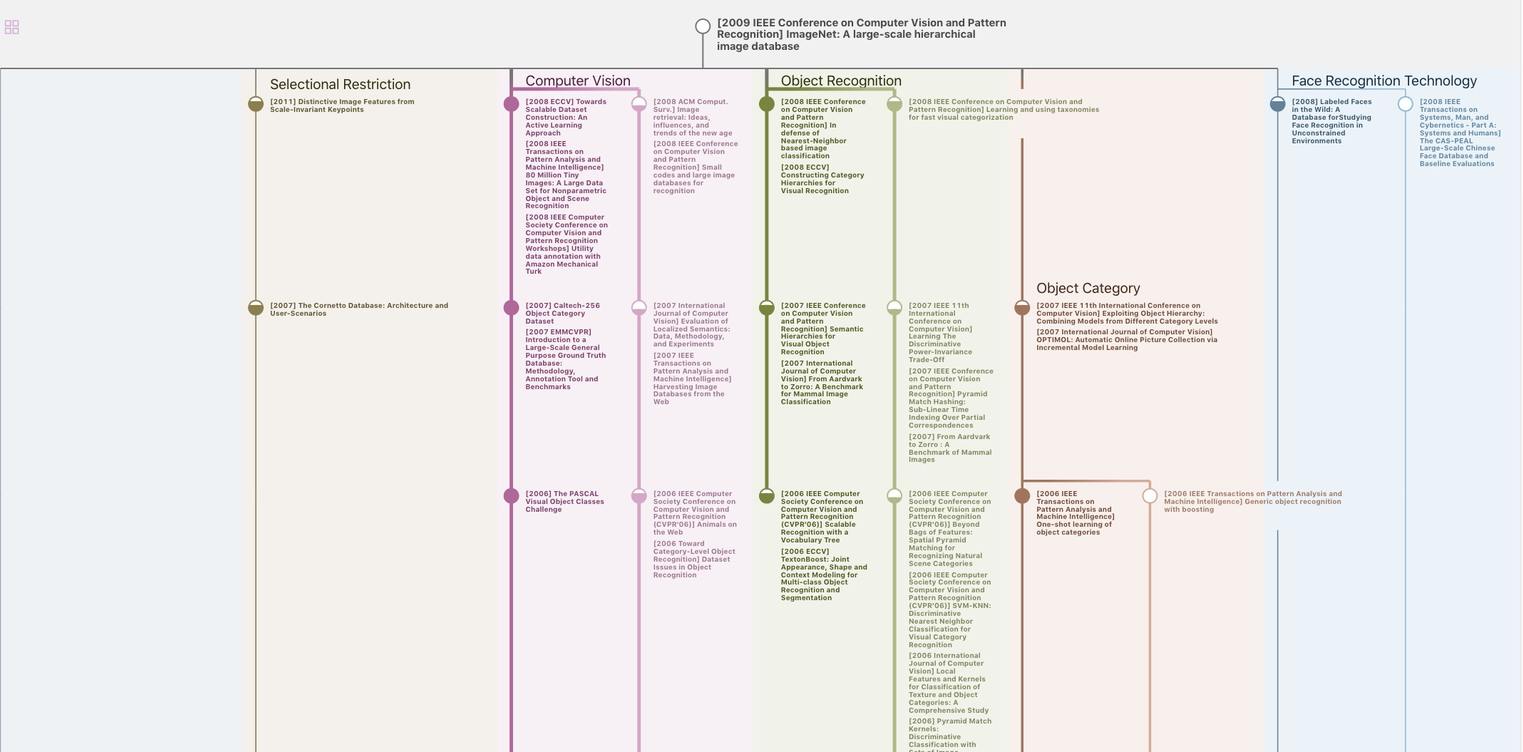
生成溯源树,研究论文发展脉络
Chat Paper
正在生成论文摘要