A New Subspace Multi-Objective Approach for the Clustering and Selection of Regions of Interests in Histopathological Images
2021 IEEE Congress on Evolutionary Computation (CEC)(2021)
摘要
Histopathology images represent a source of assistance for pathologists when diagnosing Cancer. However, in histopathology or in cancer image analysis, pathologists mostly diagnose the pathology as positive if a small part of it is considered cancer tissue. These small parts are called regions of interest (ROI) or patches. Finding the relevant patches is crucial as it can save computation time and memory. Subspace clustering discovers clusters embedded in multiple, overlapping subspaces of high dimensional data. It is an extension of feature selection, which tries to identify relevant subsets of features that are relevant to the clustering process. However, subspace clustering algorithms provide a partition of the data based on one cluster validity measure, assuming a homogeneous similarity measure over the entire data set makes the algorithms not robust to variations in the data characteristics. Therefore, it is beneficial to optimize multiple validity indices simultaneously to capture different aspects of the datasets. The goal of Multi-Objective clustering methods (MOC) is to derive significant clusters by applying two or more objective functions. This paper proposes a new clustering algorithm for patch selection based on subspace and multi-objective clustering to find the data's best partitioning and the images' most relevant patches.
更多查看译文
关键词
Subspace clustering,Multi-objective clustering,Histopathological images,Patch selection
AI 理解论文
溯源树
样例
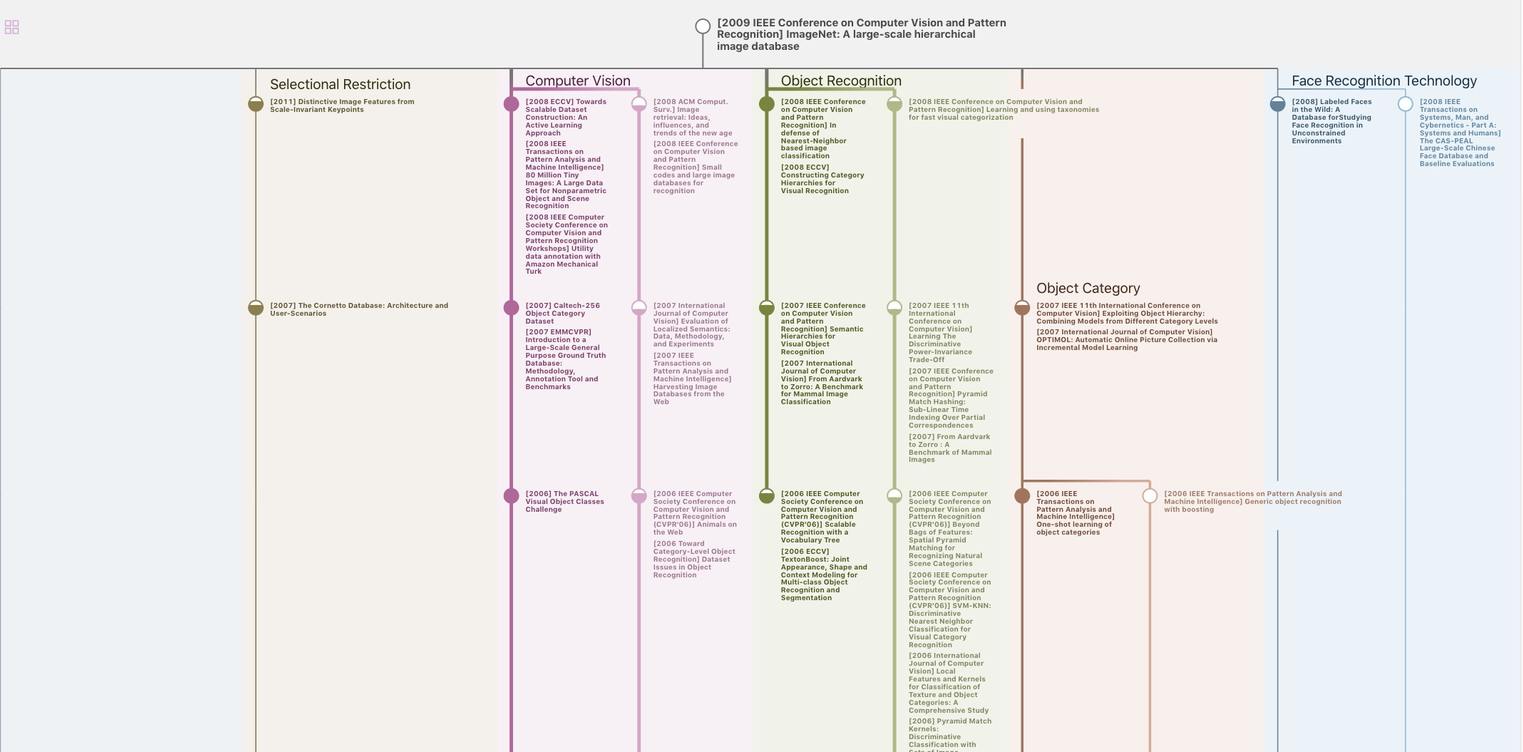
生成溯源树,研究论文发展脉络
Chat Paper
正在生成论文摘要