Multi-Objective Hyperparameter Optimization for Spiking Neural Network Neuroevolution
2021 IEEE CONGRESS ON EVOLUTIONARY COMPUTATION (CEC 2021)(2021)
摘要
Neuroevolution has had significant success over recent years, but there has been relatively little work applying neuroevolution approaches to spiking neural networks (SNNs). SNNs are a type of neural networks that include temporal processing component, are not easily trained using other methods because of their lack of differentiable activation functions, and can be deployed into energy-efficient neuromorphic hardware. In this work, we investigate two evolutionary approaches for training SNNs. We explore the impact of the hyperparameters of the evolutionary approaches, including tournament size, population size, and representation type, on the performance of the algorithms. We present a multi-objective Bayesian-based hyperparameter optimization approach to tune the hyperparameters to produce the most accurate and smallest SNNs. We show that the hyperparameters can significantly affect the performance of these algorithms. We also perform sensitivity analysis and demonstrate that every hyperparameter value has the potential to perform well, assuming other hyperparameter values are set correctly.
更多查看译文
关键词
spiking neural networks, neuromorphic computing, evolutionary algorithms
AI 理解论文
溯源树
样例
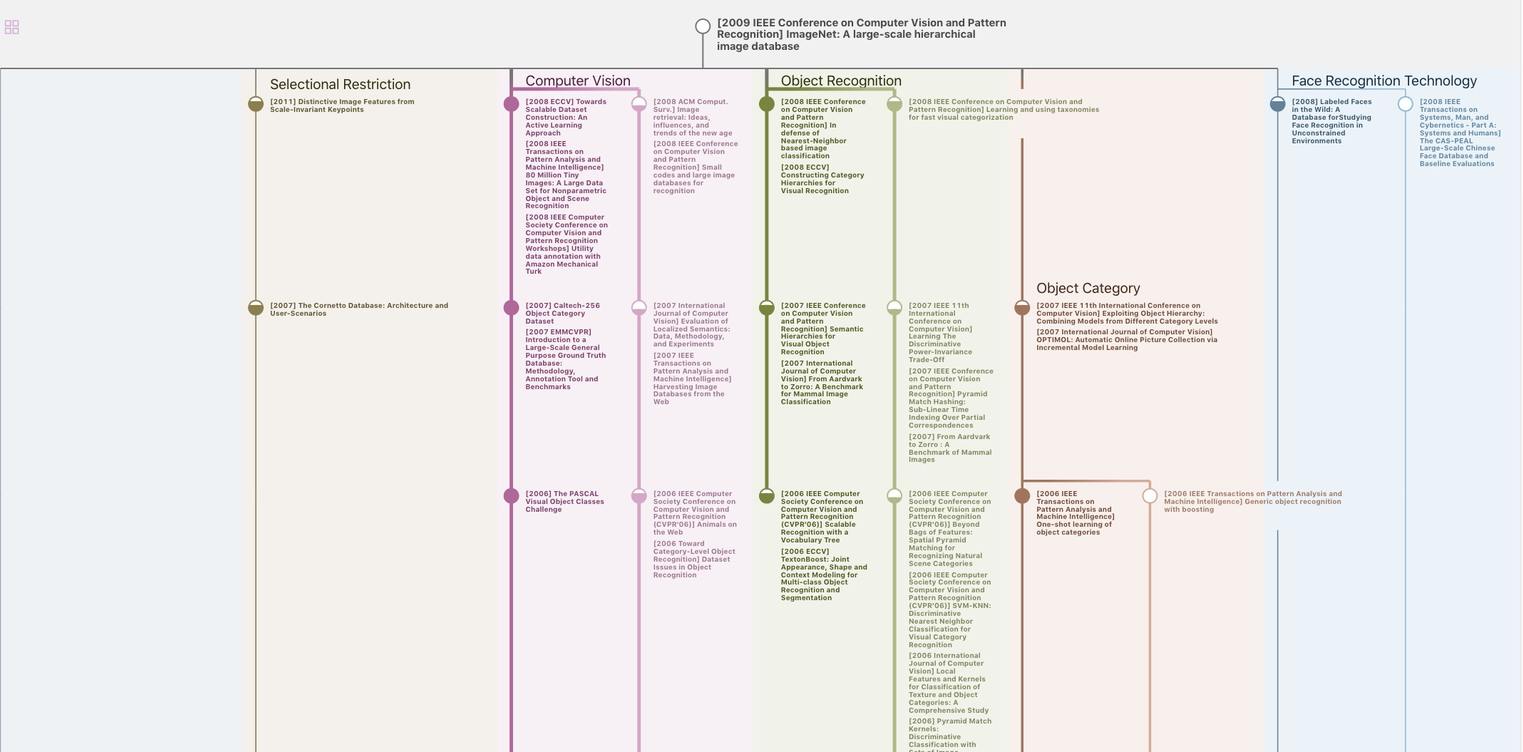
生成溯源树,研究论文发展脉络
Chat Paper
正在生成论文摘要